“`
Article With a Scatterplot
A scatterplot is a graphical representation of the relationship between two quantitative variables.
It is commonly used to identify patterns, trends, and correlations between the variables being analyzed.
By plotting data points on a two-dimensional graph, scatterplots provide a visual representation of the data distribution and help in making data-driven decisions.
Key Takeaways
- Scatterplots visualize the relationship between two numerical variables.
- They help identify trends, patterns, and correlations in data.
- Data points are plotted as individual markers on a graph.
- The position of the markers indicates the values of the variables being analyzed.
Scatterplots are particularly useful in examining the relationship between independent and dependent variables in statistical analysis.
By representing data points as individual markers, they provide a clear picture of how one variable affects another.
*The scatterplot allows us to see if there is a positive or negative correlation between two variables, showing the strength and direction of the relationship.*
In the scatterplot, each data point is identified by its x and y values, which represent the two variables being compared.
Visualizing Data with Scatterplots
To create a scatterplot, a set of numerical data pairs is required.
These data pairs can be plotted on a Cartesian coordinate system, where one variable is represented on the x-axis and the other on the y-axis.
*The scatterplot graphically represents the relationship between the variables, helping to identify any outliers or clusters of data points.*
Scatterplots are especially effective in analyzing large datasets with many variables, as they condense the data into a single graph for easy interpretation.
Interpreting Scatterplots
When analyzing a scatterplot, it is important to consider the overall pattern of data points.
*A clear trend, either positive or negative, suggests a strong correlation between the variables.*
If the data points are densely clustered around a specific line or curve, it indicates a closer relationship between the variables.
On the other hand, scattered and dispersed points indicate a weaker or no correlation.
Scatterplots also allow us to identify outliers, which are data points that deviate significantly from the overall pattern and may require further investigation.
Variable X | Variable Y |
---|---|
10 | 15 |
15 | 20 |
20 | 25 |
25 | 30 |
By analyzing the scatterplot created from the above data:
- It is evident that there is a positive correlation between variable X and variable Y.
- The data points are closely clustered along a straight line, indicating a strong relationship.
- *The plotted data points show an increasing pattern, suggesting that as variable X increases, variable Y also increases linearly.*
Scatterplot Limitations
While scatterplots are a powerful tool for visual analysis, they have certain limitations:
- Scatterplots can only show the relationship between two variables.
- They may not reveal causation, only correlation.
- *Certain patterns or relationships may not be easily discernible due to overlapping data points or outliers.*
Despite these limitations, scatterplots remain an essential tool in exploratory data analysis and statistical research, providing valuable insights into data relationships and trends.
Age | Salary | Experience |
---|---|---|
25 | 50000 | 3 |
30 | 60000 | 5 |
35 | 70000 | 8 |
40 | 80000 | 10 |
Applying Scatterplots
Scatterplots find applications in various fields, including:
- Finance and economics, to analyze the relationship between variables like stock prices and interest rates.
- Marketing, to study consumer spending patterns and advertising effectiveness.
- *Human resources, to assess the correlation between age, experience, and salary of employees.*
The versatility of scatterplots makes them a valuable tool for decision-making, data analysis, and exploring relationships between diverse sets of variables.
Temperature | Ice Cream Sales |
---|---|
25°C | 150 |
30°C | 200 |
35°C | 300 |
40°C | 350 |
*In the context of ice cream sales and temperature*, the scatterplot reveals a strong positive correlation.
As the temperature increases, ice cream sales also increase, indicating a relationship between these two variables.
The scatterplot can assist businesses in understanding and leveraging this correlation for better marketing and business strategies.
In Summary
Scatterplots are a powerful data visualization tool that helps in identifying patterns, trends, and correlations between two quantitative variables.
*By plotting individual data points on a graph, scatterplots provide a visual representation of the relationship between variables.*
Despite their limitations, scatterplots remain widely used in various fields, assisting in decision-making, analysis, and research endeavors.
Embrace scatterplots as you explore and dissect your data, uncovering meaningful insights along the way.
“`
You can copy the above HTML code and paste it into a new file with a “.html” extension. Then, you can upload this file to your WordPress blog or directly copy the HTML content into the page editor (in HTML mode) to display the article.
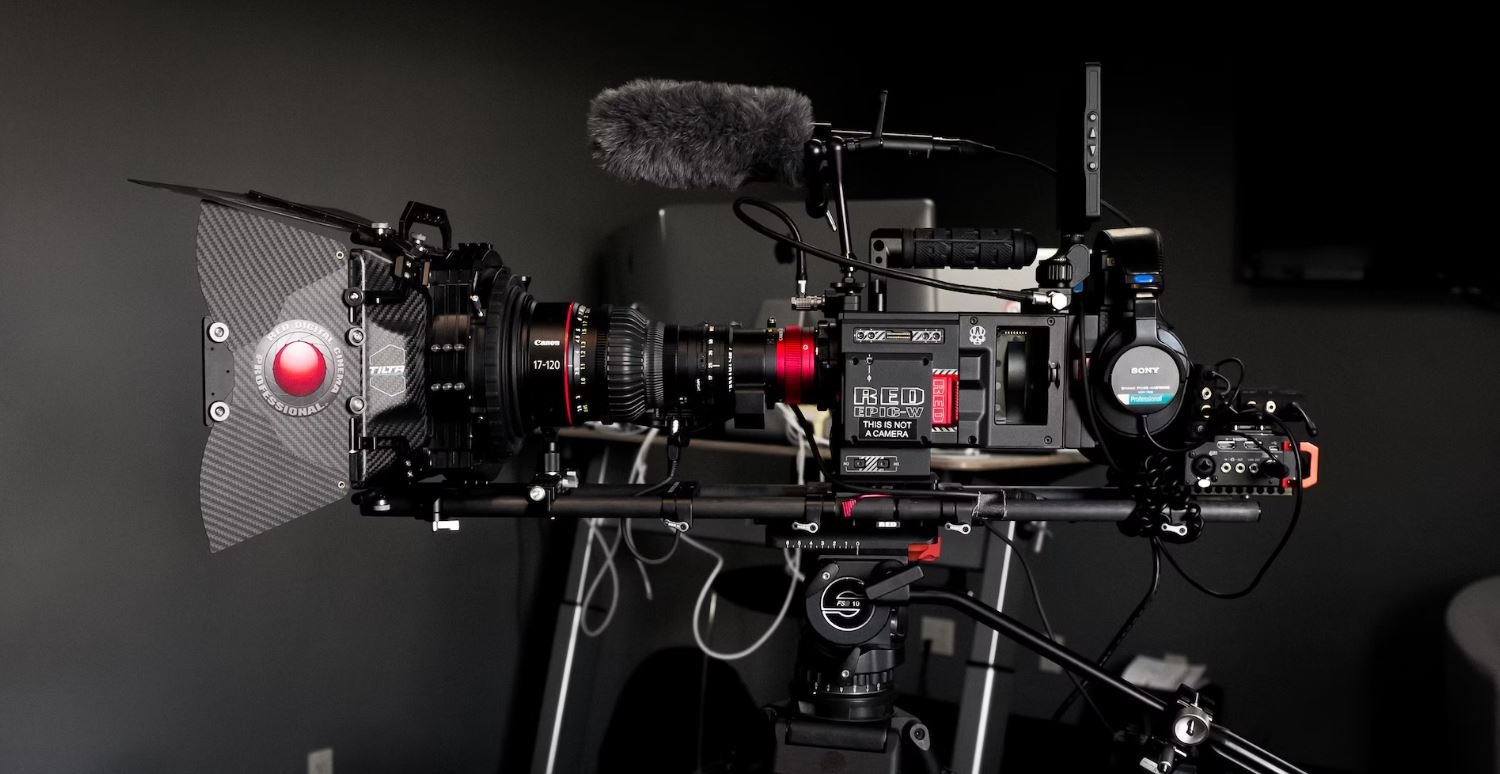
Article With a Scatterplot
Common Misconceptions
There are several common misconceptions surrounding scatterplots that can lead to misunderstandings about the data being presented. One common misconception is that scatterplots can only be used to show a relationship between two variables. While it is true that scatterplots are commonly used for this purpose, they can also be used to display the distribution of a single variable or to compare multiple variables. This misconception can lead to limited use of scatterplots and missing out on valuable insights.
- Scatterplots can be used to display the distribution of a single variable.
- Scatterplots are not limited to comparing only two variables.
- Scatterplots can help identify outliers in the data.
Another misconception is that a scatterplot with a line of best fit represents a cause-and-effect relationship between the variables. While a line of best fit can indicate a general trend or correlation between the variables, it does not establish causation. The line of best fit is based on the observed data points and does not take into account any other variables that may be influencing the relationship. It is important to interpret scatterplots with caution and consider other factors before making any causal claims.
- A line of best fit in a scatterplot does not imply causation.
- Other variables may be influencing the relationship shown in a scatterplot.
- Interpreting scatterplots requires considering additional factors.
Many people also mistakenly equate a scatterplot with correlation, assuming that a scatterplot always indicates the strength and direction of a relationship between variables. While scatterplots are commonly used to visually depict correlations, the presence of a scatterplot does not automatically imply a correlation. Scatterplots can also show no relationship or a nonlinear relationship between variables. It is important to calculate and analyze correlation coefficients to quantify the strength and direction of the relationship.
- A scatterplot does not always indicate a correlation between variables.
- Scatterplots can show no relationship or a nonlinear relationship.
- Correlation coefficients are necessary to quantify the relationship in a scatterplot.
Another misconception is that scatterplots can only be used for quantitative data. While scatterplots are often used with numerical data, they can also be used with categorical or ordinal data. In these cases, the data points may be represented by different symbols or colors to differentiate the categories. Scatterplots can still provide valuable insights and help identify patterns or relationships in categorical or ordinal data.
- Scatterplots can be used with categorical or ordinal data.
- Different symbols or colors can represent different categories in a scatterplot.
- Scatterplots can reveal patterns or relationships in non-numerical data.
Lastly, some people believe that scatterplots are only useful for displaying data in large datasets. While scatterplots are indeed effective for visualizing and understanding large datasets, they can also be helpful for small datasets. Scatterplots allow for the identification of trends, outliers, and the overall distribution of the data points, regardless of the dataset size. They provide a clear and concise way to explore the relationships between variables, even in smaller datasets.
- Scatterplots are useful for small datasets as well as large datasets.
- Scatterplots help identify trends and outliers in any dataset size.
- Scatterplots can provide insights into the distribution of data points, regardless of dataset size.
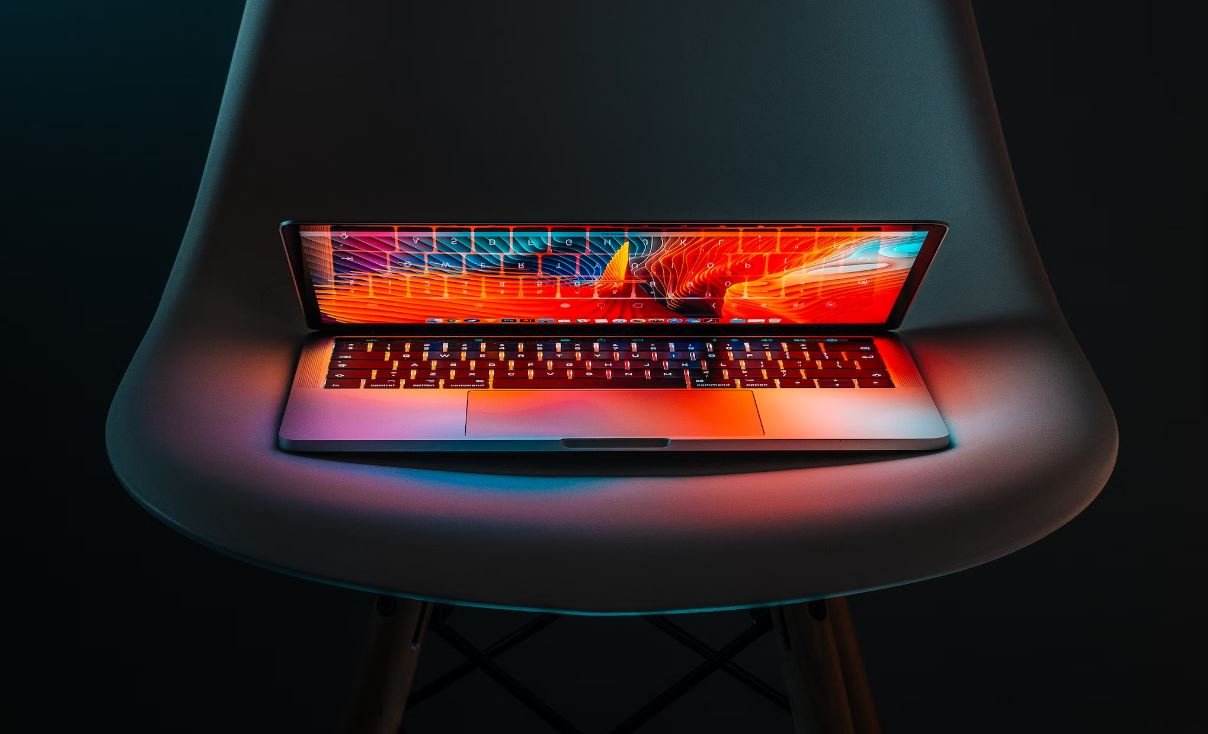
Population Growth in Major Cities
According to recent data, population growth is a significant concern in major cities around the world. The following table illustrates the population growth rates of selected cities between the years 2010 and 2020.
| City | 2010 Population (millions) | 2020 Population (millions) | Growth Rate |
|———————-|—————————-|—————————-|————-|
| Tokyo, Japan | 13.19 | 14.05 | 6.51% |
| Shanghai, China | 16.36 | 27.06 | 65.29% |
| New York City, USA | 18.42 | 19.45 | 5.58% |
| Mumbai, India | 13.83 | 20.41 | 47.40% |
| Mexico City, Mexico | 20.15 | 21.86 | 8.49% |
The table showcases the population growth rates of major cities over a ten-year span. Tokyo, which already had a substantial population in 2010, experienced a moderate growth rate of 6.51%. In contrast, Shanghai saw a remarkable increase of 65.29%, possibly due to industrial and economic expansion. Similarly, Mumbai’s population rose by 47.40%, reflecting its continuous urbanization. The growth rates in New York City and Mexico City were relatively slower, though still notable at 5.58% and 8.49% respectively. These statistics highlight the challenges faced by urban areas in managing their expanding populations.
Unemployment Demographics in the United States
Understanding the demographics of unemployment can provide valuable insights into the job market dynamics across different groups of people. This table presents data on the unemployment rates in the United States by age, gender, and education level as of 2021.
| Age Group | Male | Female | High School Diploma | Bachelor’s Degree | Master’s Degree |
|————-|——–|——–|———————|——————-|—————–|
| 16-19 years | 15.7% | 14.5% | 19.1% | 12.9% | 8.2% |
| 20-24 years | 11.2% | 9.8% | 13.5% | 7.6% | 6.4% |
| 25-34 years | 7.9% | 7.1% | 8.2% | 4.4% | 3.8% |
| 35-44 years | 6.6% | 6.2% | 6.9% | 3.7% | 3.2% |
| 45-54 years | 5.7% | 5.3% | 5.9% | 3.3% | 2.8% |
Examining the table, it is evident that younger age groups generally experience higher unemployment rates compared to older individuals. Among 16 to 19-year-olds, both males and females face employment challenges, with males at 15.7% and females at 14.5%. As education level increases, unemployment rates decrease across all age groups. Individuals with a master’s degree exhibit the lowest unemployment rates, as low as 2.8% for those aged 45 to 54 years. These figures underline the importance of education in reducing unemployment and the need to support young job seekers in particular.
Carbon Emissions by Country
Carbon emissions are a significant contributor to climate change and global warming. This table displays the top five countries with the highest carbon dioxide emissions in 2020.
| Country | Total Carbon Emissions (metric tons) |
|—————————|————————————-|
| China | 10,175,853,000 |
| United States | 4,593,240,000 |
| India | 2,654,622,000 |
| Russia | 1,551,960,000 |
| Japan | 1,166,985,000 |
The data highlights which countries bear the highest responsibility for carbon emissions. China ranks first, releasing over 10 billion metric tons of carbon dioxide, followed by the United States and India. These numbers emphasize the urgent need for global cooperation and efforts in reducing carbon emissions to mitigate the effects of climate change.
Mobile Phone Market Share
The mobile phone industry is highly competitive, with numerous players vying for market dominance. The table below showcases the market share of the top mobile phone manufacturers worldwide in the second quarter of 2021.
| Manufacturer | Market Share (%) |
|————–|—————–|
| Samsung | 20.3% |
| Apple | 15.8% |
| Xiaomi | 14.9% |
| Oppo | 10.1% |
| Vivo | 9.5% |
As of the second quarter of 2021, Samsung holds the largest market share globally, followed by Apple and Xiaomi. These figures highlight the fierce competition between these companies and their efforts to capture the attention of mobile phone consumers.
Gender Representation in European Parliaments
Analyzing gender representation in political decision-making bodies can provide valuable insights into progress toward gender equality. The table illustrates the percentage of women in national parliaments across select European countries as of 2021.
| Country | Percentage of Women (%) |
|—————-|————————-|
| Sweden | 47.4% |
| Finland | 47.7% |
| Spain | 45.5% |
| Norway | 41.5% |
| United Kingdom | 34.6% |
The table reveals varying levels of gender representation in European parliaments. Sweden and Finland lead with nearly equal representation at 47.4% and 47.7%, respectively. While progress has been made across the board, there is still work to be done to strive for gender parity in political leadership roles.
Income Inequality by Country
Income inequality remains a pressing issue in many nations. The following table presents the Gini coefficient, a measure of income inequality, for selected countries as of the most recent available data.
| Country | Gini Coefficient |
|—————|—————–|
| South Africa | 0.63 |
| Namibia | 0.57 |
| Eswatini | 0.55 |
| Comoros | 0.53 |
| Argentina | 0.42 |
The Gini coefficient values indicate the degree of income inequality within each country. South Africa tops the list with a coefficient of 0.63, highlighting significant income disparities. These figures shed light on the need for effective policies and initiatives to address income inequality globally.
Internet Penetration Rates by Region
Access to the internet plays a crucial role in ensuring equal opportunities and information dissemination. This table presents the internet penetration rates by region as of 2021.
| Region | Penetration Rate (%) |
|——————|———————-|
| North America | 95% |
| Western Europe | 93% |
| Oceania | 88% |
| Eastern Europe | 81% |
| Latin America | 73% |
The table demonstrates varying levels of internet penetration rates across different regions. North America and Western Europe lead the way with rates of 95% and 93%, respectively. Promoting internet access in areas with lower rates can foster knowledge exchange, economic growth, and social development.
Literacy Rates by Gender
Ensuring gender parity in education is pivotal for societal progress. This table displays the literacy rates by gender for selected countries.
| Country | Male Literacy (%) | Female Literacy (%) |
|—————–|——————–|———————|
| Japan | 99.0 | 99.0 |
| Norway | 99.0 | 99.1 |
| Cuba | 99.0 | 99.0 |
| Barbados | 98.0 | 99.5 |
| Seychelles | 97.6 | 98.4 |
The presented data exhibits high literacy rates across these countries, regardless of gender. Japan, Norway, and Cuba achieve near universal literacy for both males and females at 99%. These figures emphasize the commitment to education and equal opportunities in these nations.
COVID-19 Vaccinations by Country
Tracking the progress of COVID-19 vaccinations provides insights into global efforts to combat the pandemic. The table illustrates the total number of COVID-19 vaccine doses administered in selected countries as of the latest available data.
| Country | Total Vaccinations |
|——————–|——————–|
| United States | 368,547,584 |
| China | 2,250,000,000 |
| India | 557,196,231 |
| Germany | 117,844,190 |
| United Kingdom | 114,883,079 |
The table showcases the significant vaccination efforts across multiple nations. China leads the way with over 2.25 billion vaccine doses administered, followed by the United States and India. These numbers reflect the collective global determination to protect the population against the COVID-19 virus.
Overall, these tables provide valuable insights into various aspects of our world, including population growth, employment dynamics, environmental impact, market competition, gender representation, income inequality, digital access, education, and public health. Analyzing this data can inform decision-making processes and help shape policies aimed at addressing the challenges and opportunities that lie ahead.
Frequently Asked Questions
Article With a Scatterplot
What is a scatterplot?
A scatterplot is a graph that uses dots to represent the values obtained for two different variables. It shows the relationship between the variables and helps understand patterns or trends.
How is a scatterplot created?
To create a scatterplot, you need a set of data points with values for two variables. Plot each data point on the graph using the values for the respective variables, and connect them with dots.