Orca AI Paper
In recent years, artificial intelligence (AI) has made significant strides in various industries, and maritime technology is no exception. Orca AI, a leading company in the field of AI-based maritime surveillance and navigation, has recently released a groundbreaking research paper that has garnered attention and acclaim from experts in the industry. This article provides an overview of the key findings and advancements discussed in the Orca AI paper.
Key Takeaways:
- Orca AI’s research paper highlights the potential of AI in enhancing maritime surveillance and safety.
- The paper discusses the advantages of using AI systems for early detection of maritime collisions and potential hazards.
- Orca AI’s technology has proven to be effective in improving vessel safety and reducing operational costs for maritime companies.
- The research paper emphasizes the need for continued investment and development in AI technology for the maritime industry.
One of the main focuses of the Orca AI paper is the use of AI systems for the early detection of maritime collisions. *This technology has the potential to save countless lives and reduce the risk of environmental disasters.* By analyzing real-time data from various sensors and incorporating advanced algorithms, Orca AI’s system can accurately identify potential collision risks and provide timely alerts to vessel operators. This proactive approach to maritime safety is a significant step forward in ensuring the well-being of both crew members and the environment.
Advancements in Maritime Surveillance
The Orca AI research paper delves into the advancements made in maritime surveillance and highlights the unique capabilities of their AI technology. Using deep learning algorithms, the system can analyze and interpret data from various sources, including radar, cameras, and AIS (Automatic Identification System). *This powerful combination of AI and sensor data enables the system to detect and track vessels even in challenging conditions such as low visibility.* This not only improves navigational safety but also provides valuable insights for vessel operators and maritime authorities.
Table 1: Comparison of Traditional Systems vs. Orca AI
Traditional Systems | Orca AI |
---|---|
Relies heavily on manual monitoring and human interpretation | Utilizes AI algorithms to automate surveillance and detect potential hazards |
Can miss critical information due to human error or fatigue | Provides real-time alerts and insights without the risk of human oversight |
Reactive approach to collision prevention | Proactive early warning system for collision risks |
The Orca AI paper also emphasizes the economic benefits associated with their AI technology. By leveraging AI algorithms, vessel operators can optimize their routes and operations, reducing fuel consumption and overall operational costs. This efficiency not only benefits the companies themselves but also has a positive impact on the environment by reducing carbon emissions. The potential for cost savings and environmental sustainability further solidifies the value of AI in the maritime industry.
Table 2: Cost Savings with Orca AI
Vessel Type | Projected Annual Savings (USD) |
---|---|
Tanker | $500,000 |
Container Ship | $300,000 |
Bulk Carrier | $400,000 |
Moreover, the Orca AI research paper highlights the need for continuous investment and development in AI technology. With the ever-increasing complexity and volume of maritime data, AI systems play a crucial role in efficiently analyzing and extracting actionable insights. The paper calls for collaboration between industry stakeholders and technology developers to further improve the accuracy and effectiveness of AI-based maritime surveillance systems.
Advancements and Collaborations
- Orca AI’s research paper advocates for continued advancements in AI-based maritime surveillance technology.
- Industry collaborations and partnerships can further enhance the capabilities and reach of AI systems.
- The paper underlines the importance of staying at the forefront of AI innovations to ensure maritime safety and efficiency.
Table 3: Notable Collaborations
Collaborating Companies | Objective |
---|---|
Intel Corporation | Integrating AI technology into marine hardware |
Mitsui O.S.K. Lines | Testing and validating the effectiveness of Orca AI’s technology in real-world scenarios |
Maritime Autonomous Surface Ships (MASS) initiative | Exploring the potential of AI in unmanned and autonomous vessels |
In conclusion, the Orca AI research paper sheds light on the significant advancements made in the field of AI-based maritime surveillance. Through their innovative technology, Orca AI has showcased the immense potential of AI in improving vessel safety, optimizing operations, and reducing costs. The paper also highlights the importance of continuous investment, collaborations, and partnerships to further enhance the capabilities of AI systems in the maritime industry.
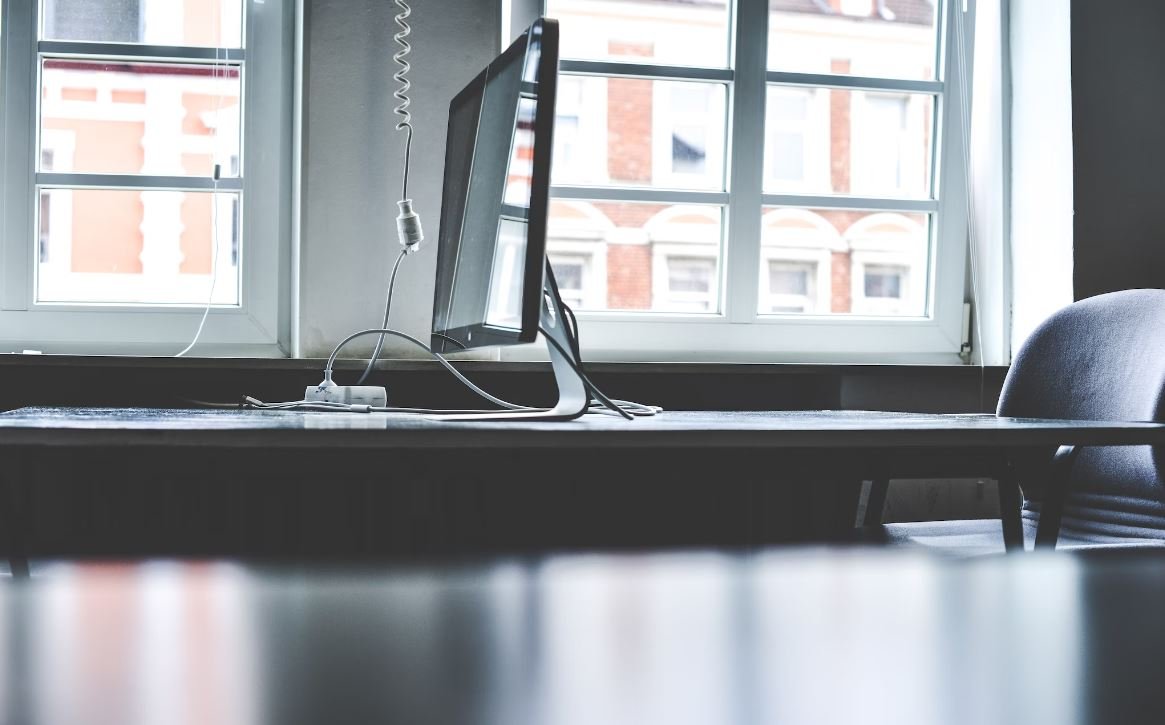
Common Misconceptions
Orca AI Paper Title
There are several common misconceptions that people have about Orca AI. These misconceptions often arise from a lack of information or misinterpretation of the available data. It is important to address these misconceptions to ensure a better understanding of this topic.
- Orca AI is just another fancy name for artificial intelligence.
- Orca AI is only useful for large corporations.
- Orca AI is a replacement for human intelligence.
Misconception 1: Orca AI is just another fancy name for artificial intelligence.
One common misconception is that Orca AI is simply a buzzword for artificial intelligence. While Orca AI does utilize AI technologies, it goes beyond traditional AI applications. Orca AI is a specialized platform that focuses specifically on maritime safety and risk management. It incorporates machine learning algorithms to analyze maritime data and provide actionable insights for ship operators.
- Orca AI is a specialized platform for maritime safety.
- It goes beyond traditional AI applications.
- Orca AI utilizes machine learning algorithms.
Misconception 2: Orca AI is only useful for large corporations.
Another common misconception is that Orca AI is only beneficial for large corporations with vast shipping fleets. However, this is not the case. While Orca AI can indeed provide significant benefits for large companies, its applications extend to small and medium-sized enterprises as well. Any ship operator can benefit from Orca AI’s risk management and safety solutions, regardless of fleet size.
- Orca AI is not limited to large corporations.
- Small and medium-sized enterprises can also benefit from it.
- Any ship operator, regardless of fleet size, can utilize Orca AI.
Misconception 3: Orca AI is a replacement for human intelligence.
Some people mistakenly believe that Orca AI aims to replace human intelligence in the maritime industry. However, Orca AI is designed to complement human intelligence, not replace it. The platform enhances human decision-making processes by providing valuable insights and actionable recommendations. Ultimately, it is a tool that empowers ship operators and improves their decision-making abilities.
- Orca AI complements human intelligence.
- It enhances decision-making processes.
- The platform empowers ship operators.
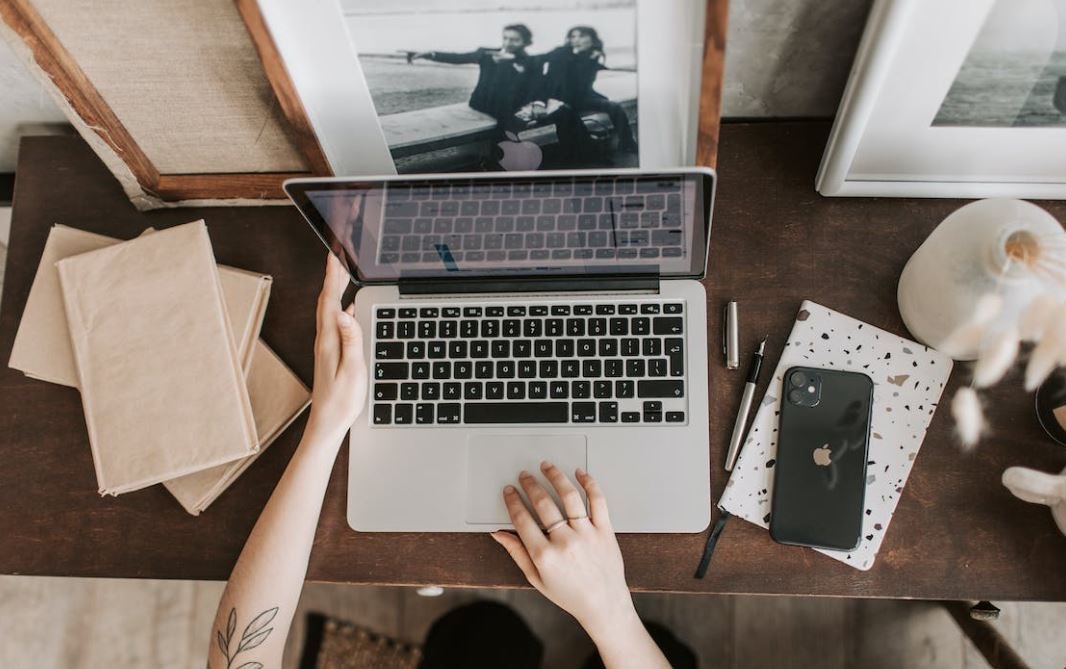
The Importance of Data in Artificial Intelligence Development
As the field of artificial intelligence continues to advance, the availability and quality of data play a vital role in its development. Accurate and diverse datasets enable AI systems to learn effectively and make more informed decisions. The following tables highlight various aspects of data utilization in AI research and development.
1. Data Collection Methods in AI Development
The table below showcases different approaches used to collect data for training AI models.
Data Collection Method | Description |
---|---|
Web Scraping | Gathering data from websites using automated tools |
Crowdsourcing | Utilizing human input to gather data through platforms |
Sensor Networks | Deploying networked devices for data collection in real-world environments |
2. Types of Data Used in AI Models
This table categorizes the different types of data commonly employed in AI models.
Data Type | Description |
---|---|
Numerical Data | Data represented by numbers and used for quantitative analysis |
Textual Data | Unstructured data in the form of text used for natural language processing |
Image Data | Visual data used for computer vision tasks |
Temporal Data | Data with a temporal component, such as time series data |
3. Sources of Bias in AI Datasets
This table sheds light on potential sources of bias that can be present in AI datasets.
Bias Source | Description |
---|---|
Sampling Bias | Occurs when the data collection process is not representative of the target population |
Labeling Bias | Biased annotations or labels can introduce bias into the dataset |
Historical Bias | Reflects biases in past societal norms or discriminatory practices |
Algorithmic Bias | Biases introduced by the AI algorithms themselves during data processing |
4. Data Augmentation Techniques
The table below showcases different techniques used to augment datasets for better AI model performance.
Data Augmentation Technique | Description |
---|---|
Image Rotation | Rotating images in various angles to increase dataset diversity |
Data Mixing | Combining multiple datasets to create a more diverse training set |
Text Synonym Replacement | Replacing words with synonyms to increase textual dataset variability |
5. Impact of Data Quality on AI Models
This table highlights the consequences of varying data quality on the performance of AI models.
Data Quality Level | Impact on AI Model |
---|---|
High Quality | Improved accuracy and robustness of the AI model |
Low Quality | Increased risk of errors and reduced reliability |
Noisy Data | Can lead to biased outcomes and hinder model performance |
6. Data Governance Practices in AI
This table outlines various data governance practices implemented to ensure responsible and ethical use of data in AI development.
Data Governance Practice | Description |
---|---|
Data Anonymization | Removing personally identifiable information from datasets to protect privacy |
Data Encryption | Applying cryptographic techniques to secure data during storage and transmission |
Data Access Controls | Managing permissions and restrictions to control access and usage of data |
7. Data Labeling Techniques
This table presents different methods used to label data for training AI models.
Data Labeling Technique | Description |
---|---|
Manual Labeling | Human annotators manually assign labels to data points |
Active Learning | AI models interactively query human experts for data labeling |
Weak Supervision | Utilizing noisy labels or heuristics for labeling large-scale datasets |
8. Ethics and Bias Mitigation Strategies
This table illustrates different strategies employed to address ethical concerns and mitigate bias in AI development.
Strategy | Description |
---|---|
Diverse Data Collection | Gathering data from diverse sources and demographics to mitigate bias |
Algorithmic Fairness | Developing AI models that prevent unfair discrimination based on protected attributes |
External Auditing | Engaging third-party auditors to assess and ensure ethical AI implementation |
9. Data Privacy Regulations
This table highlights various regulations and standards aimed at protecting data privacy in AI applications.
Regulation/Standard | Description |
---|---|
General Data Protection Regulation (GDPR) | EU legislation ensuring the protection of personal data and privacy |
California Consumer Privacy Act (CCPA) | California state law granting consumers various privacy rights |
ISO/IEC 27001 | International standard providing guidelines for information security management |
10. Availability of Publicly Accessible AI Datasets
This table showcases different sources where researchers and developers can access publicly available AI datasets.
Source | Description |
---|---|
Kaggle | A platform hosting a wide range of datasets for AI and machine learning |
UCI Machine Learning Repository | An online repository offering various datasets for research purposes |
Open Images Dataset | A large-scale dataset with annotated images for computer vision tasks |
As the field of artificial intelligence progresses, ensuring the availability and quality of data becomes increasingly important. Collecting diverse and reliable datasets, addressing biases, and following ethical practices are crucial for developing AI models that accurately represent the world and make unbiased decisions. By understanding the significance of data in AI development, we can shape AI technologies that positively impact various industries and society as a whole.
Frequently Asked Questions
Q: What is Orca AI?
Orca AI is a cutting-edge artificial intelligence system developed by a team of researchers. It incorporates advanced algorithms and machine learning techniques to analyze complex data and provide insights.
Q: How does Orca AI work?
Orca AI uses a combination of natural language processing, deep learning, and predictive analytics to process and understand large volumes of data. It can extract patterns, detect anomalies, and generate meaningful predictions based on the input it receives.
Q: What types of data can Orca AI analyze?
Orca AI is designed to analyze various types of data including text documents, numerical data, images, and audio files. It can handle structured and unstructured data and is capable of dealing with diverse data formats.
Q: How accurate is Orca AI’s analysis?
Orca AI’s accuracy depends on the quality of the input data and the complexity of the analysis required. In general, it has been shown to deliver impressive accuracy rates in a wide range of applications, but specific results may vary depending on the use case.
Q: What industries can benefit from using Orca AI?
Orca AI can be beneficial to a wide range of industries including finance, healthcare, marketing, cybersecurity, logistics, and more. Its capabilities make it suitable for organizations seeking data-driven insights to optimize processes, make informed decisions, and gain a competitive edge.
Q: Can Orca AI be customized to fit specific business needs?
Yes, Orca AI is a flexible system that can be customized to meet the unique requirements of different businesses. It can be trained on specific data sets and tailored to address specific challenges or goals.
Q: Is Orca AI compatible with existing software systems?
Yes, Orca AI is designed to seamlessly integrate with existing software systems. Its APIs allow for easy integration with various programming languages and frameworks, making it adaptable to different technology environments.
Q: Is Orca AI secure?
Orca AI puts a strong emphasis on security and implements robust measures to protect data. It utilizes encryption, authentication mechanisms, and secure data transfer protocols to ensure the confidentiality and integrity of the information it handles.
Q: How can Orca AI benefit businesses?
Orca AI can benefit businesses by providing accurate insights, automating repetitive tasks, improving decision-making processes, optimizing operations, and enhancing customer experience. It can help businesses identify patterns, trends, and anomalies that may not be easily detectable through traditional analysis methods.
Q: Can Orca AI be used by individuals or is it only for large corporations?
Orca AI is designed to be used by both individuals and organizations of all sizes. While large corporations can leverage its capabilities for complex data analysis tasks, individuals can also benefit from its user-friendly interface for simpler data-driven insights.