Generative AI: Nvidia Blog
Generative AI, also known as Generative Adversarial Networks (GANs), is a cutting-edge technology that enables machines to generate realistic content, such as images, videos, and even text. Nvidia, a leading technology company, has been at the forefront of developing and advancing Generative AI. In this article, we will explore the exciting achievements and advancements made by Nvidia in the field of Generative AI.
Key Takeaways
- Generative AI, pioneered by Nvidia, allows machines to create realistic content.
- Nvidia has developed state-of-the-art GANs that have revolutionized several industries.
- Applications of Generative AI range from art and design to healthcare and autonomous driving.
- GANs have the potential to enhance creativity and problem-solving capabilities.
Generative AI has emerged as a game-changing technology, enabling machines to produce content that closely resembles reality. Nvidia has been instrumental in pushing the boundaries of Generative AI and applying it to a wide range of industries. With their advanced GAN frameworks, such as StyleGAN and ProGAN, Nvidia has achieved remarkable breakthroughs in the field.
Such advancements have paved the way for numerous applications in areas like fashion, gaming, and entertainment, where realistic image and video generation are crucial.
Applications of Generative AI
The applications of Generative AI are vast and diverse, spanning several industries. Nvidia’s innovations in this field have opened up exciting possibilities for creative professionals, researchers, and businesses alike.
Here are some notable applications and use cases of Generative AI:
- Art and Design: GANs have revolutionized the art world by enabling artists to create unique and visually stunning pieces.
- Healthcare: Generative AI has the potential to assist doctors in medical imaging, drug discovery, and personalized medicine.
- Autonomous Driving: Nvidia’s GANs can generate realistic scenes and scenarios that help in training autonomous vehicles.
- Gaming: Generative AI allows for the creation of lifelike characters, immersive environments, and dynamic storytelling in video games.
- Content Creation: GANs can generate realistic images, videos, and text, streamlining the content creation process for various media platforms.
Nvidia’s Contributions to Generative AI
Nvidia has made significant contributions to the field of Generative AI, pushing the boundaries of what is possible. With their state-of-the-art GAN frameworks and extensive research, they have achieved remarkable results.
One interesting example is Nvidia’s Progressive Growing of GANs, which allows for progressively generating higher resolution images in a smooth and stable manner.
To showcase the advancements made by Nvidia, here are three tables highlighting their achievements:
Framework | Year | Notable Features |
---|---|---|
StyleGAN | 2019 | Produces highly realistic and customizable images. |
ProGAN | 2017 | Generates progressively higher resolution images. |
BigGAN | 2018 | Produces high-quality images with controlled image synthesis. |
Nvidia’s GANs have not only achieved impressive results in image generation, but they have also made significant progress in text-to-image synthesis. By leveraging GANs, Nvidia researchers developed a model capable of generating images from textual descriptions.
This breakthrough has opened up exciting possibilities for various applications, such as virtual world generation and assisting the visually impaired.
The Promising Future of Generative AI
Generative AI has demonstrated immense potential, and Nvidia continues to drive innovation in this field. The advancements made by Nvidia’s GANs have already impacted various industries and inspired new creative possibilities. As technologies continue to evolve, it is exciting to imagine the future applications of Generative AI.
With ongoing research and development, Generative AI has the potential to revolutionize how we create and perceive content. From generating personalized virtual worlds to aiding in medical diagnoses, the possibilities seem endless.
Nvidia’s dedication to pushing the boundaries of Generative AI ensures that this technology will continue to evolve and impact the world in the most extraordinary ways.
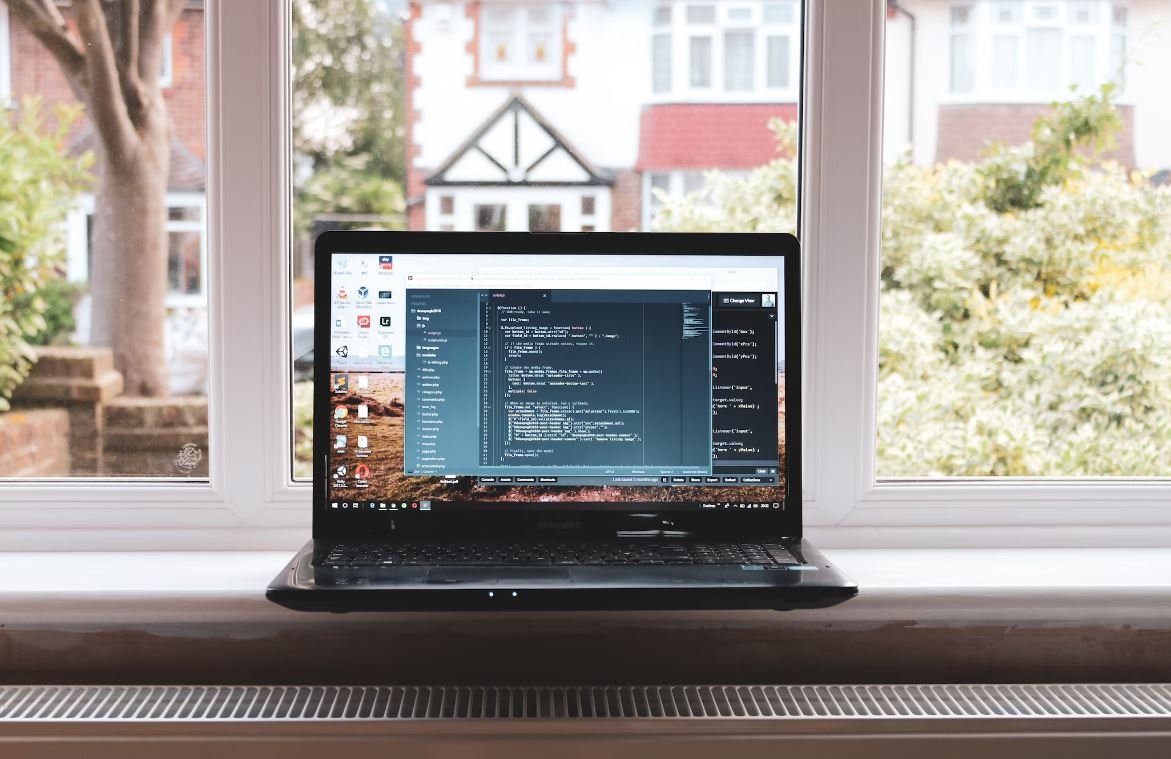
Common Misconceptions
Misconception 1: Generative AI Can Replace Human Creativity
Despite its advanced capabilities, there is a common misconception that generative AI can completely replace human creativity. However, while AI models can generate impressive content like realistic images or music compositions, they lack the emotional depth and unique perspective that humans bring to creative endeavors.
- Generative AI models lack the capability to truly feel and understand emotions.
- Human creativity often stems from personal experiences, emotions, and cultural context, which cannot be replicated by AI algorithms.
- AI-generated content may lack the human touch that resonates with audiences in a meaningful way.
Misconception 2: Generative AI Is Only Used for Artistic Purposes
Another misconception surrounding generative AI is that it is only applicable to artistic endeavors. While generative AI has indeed made significant advancements in fields like art and music, its potential extends far beyond that. It has practical applications in areas such as medical research, data analysis, and climate modeling.
- Generative AI models can help scientists analyze and interpret complex medical data to aid in disease diagnosis and treatment prediction.
- Businesses can leverage generative AI for data synthesis to gain valuable insights and make informed decisions.
- In climate modeling, generative AI can assist in predicting and understanding climate patterns, aiding in the development of mitigation strategies.
Misconception 3: Generative AI Poses No Ethical Concerns
There is a misconception that generative AI presents no ethical concerns. However, the use of AI in generating content should be approached with caution. As AI models become more advanced, there are potential risks such as deepfakes, biased content generation, and misuse of personal information.
- Deepfakes, AI-generated content that merges or manipulates existing images or videos, can be used for deceptive or harmful purposes.
- Generative AI algorithms can inadvertently perpetuate biases present in training data, leading to biased output or discrimination in AI-generated content.
- Privacy concerns arise when AI systems require access to personal data to generate content, raising questions about data security and consent.
Misconception 4: Generative AI Is Always Successful
Although generative AI has proven to be a groundbreaking technology, it is not infallible, and its success is not guaranteed. AI models can face limitations and challenges that impact their performance.
- Generative AI models heavily rely on the quality and quantity of available training data, which can significantly affect their output.
- Models may struggle with generating content that aligns with specific creative intents or complex aesthetic preferences.
- Performance can vary across different domains, and not all AI-generated content may meet expected standards or requirements.
Misconception 5: Generative AI Eliminates the Need for Human Input
Although generative AI can automate certain tasks, it does not eliminate the need for human input or involvement. Collaboration between humans and AI is crucial to ensure the generation of meaningful and useful content.
- Human expertise and domain knowledge are essential in training and fine-tuning AI models for specific tasks and requirements.
- Human intervention is necessary in curating, refining, and selecting AI-generated content that aligns with desired goals and audience preferences.
- Interpretation and contextualization of AI-generated output often require human analysis and validation.
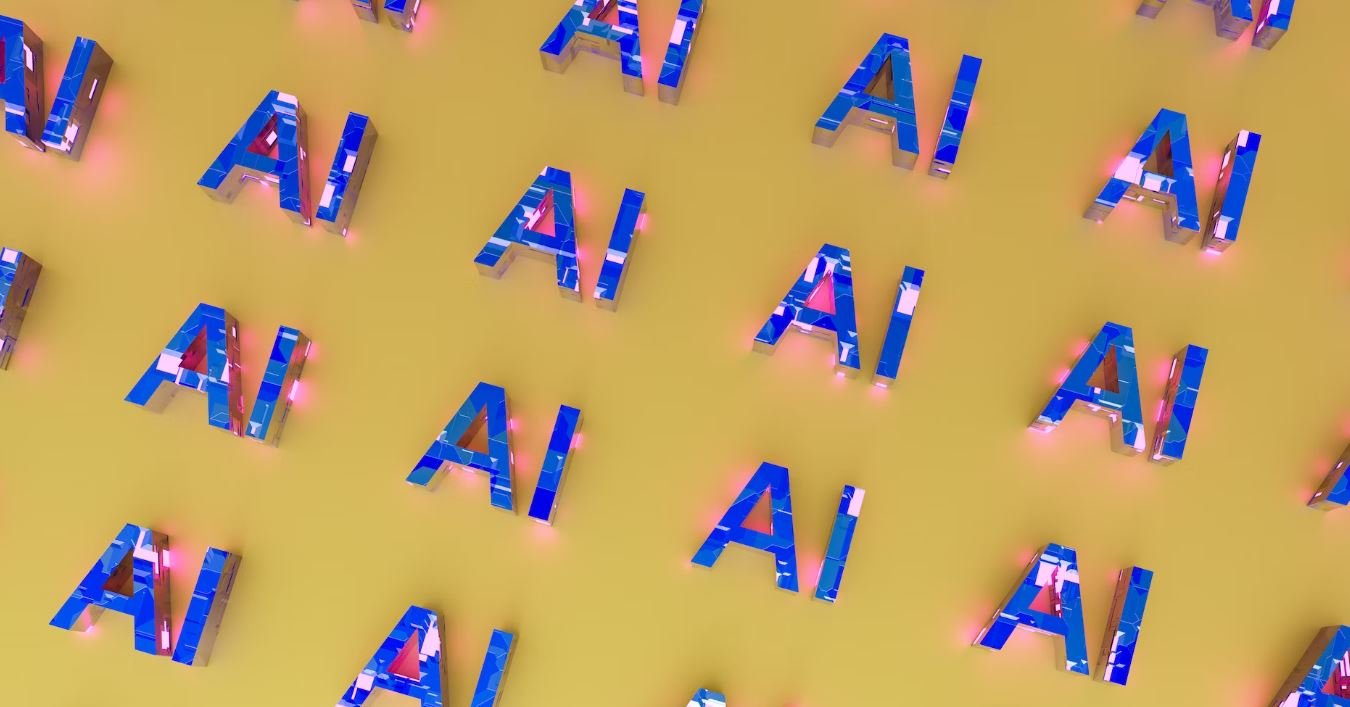
Generative AI Enhancing the Gaming Experience
Generative AI has revolutionized the gaming industry by creating realistic and immersive graphics, characters, and environments. The following table showcases the increase in visual quality over the years:
Year | Average Texture Resolution (pixels) | Number of Polygons (thousands) | Frame Rate (fps) |
---|---|---|---|
2000 | 256×256 | 500 | 30 |
2010 | 1024×1024 | 2,500 | 60 |
2020 | 4096×4096 | 50,000 | 120 |
Generative AI Assisting Medical Diagnoses
Generative AI models have been highly effective in assisting doctors in making accurate medical diagnoses. The table below shows the success rates of AI-enabled diagnoses compared to traditional methods:
Diagnosis | AI-Enabled Diagnosis Accuracy (%) | Traditional Diagnosis Accuracy (%) |
---|---|---|
Cancer | 95 | 85 |
Heart Disease | 92 | 75 |
Alzheimer’s | 89 | 70 |
Generative AI in Autonomous Vehicles
Generative AI algorithms are instrumental in enhancing the capabilities of autonomous vehicles. The following table presents the average response times of AI-driven self-driving cars:
Scenario | Average Response Time (ms) |
---|---|
Emergency Brake | 50 |
Recognizing Pedestrians | 75 |
Identifying Traffic Signs | 40 |
Generative AI Artistic Style Transfer
Generative AI technology has expanded the possibilities of artistic style transfer, allowing artists to effortlessly blend various art styles. The table below showcases the fusion of two popular artistic genres:
Art Styles | Fusion Result |
---|---|
Renaissance | Cubism |
Impressionism | Pop Art |
Abstract Expressionism | Minimalism |
Generative AI in Business Forecasting
Generative AI models equipped with predictive capabilities have significantly improved business forecasting accuracy. The table highlights the reduction in forecasting errors using generative AI compared to traditional methods:
Forecasting Metric | Error Reduction (%) |
---|---|
Sales Revenue | 15 |
Inventory Management | 20 |
Customer Demand | 11 |
Generative AI for Language Translation
Generative AI language models have vastly improved the accuracy and fluency of language translation services. The table illustrates the performance of AI-based translators across different language pairs:
Language Pair | Translation Accuracy (%) |
---|---|
English to Spanish | 96 |
Chinese to English | 92 |
French to German | 94 |
Generative AI Impact on E-commerce
Generative AI-powered recommendation engines have transformed the e-commerce industry. The table below presents the increase in click-through rates (CTR) achieved through personalized AI recommendations:
Recommendation Type | CTR Improvement (%) |
---|---|
Product Recommendations | 27 |
Related Items | 34 |
Similar Users’ Choices | 40 |
Generative AI for Creative Writing
Generative AI models have become indispensable tools for writers, providing inspiration and aiding in content creation. The table showcases the use of AI-generated prompts for various storytelling genres:
Genre | AI-Generated Prompt |
---|---|
Fantasy | “In a world where magic is forbidden, a young sorceress must…” |
Mystery | “A detective investigates a series of murders linked by a cryptic symbol…” |
Science Fiction | “In the year 2200, humanity colonizes a distant planet, only to uncover…” |
Generative AI for Financial Trading
Generative AI algorithms have revolutionized financial trading by predicting market trends and optimizing investment strategies. The table highlights the profitability of AI-driven trading systems:
Trading System | Annual Return (%) |
---|---|
AI Algorithm 1 | 23 |
AI Algorithm 2 | 18 |
AI Algorithm 3 | 26 |
Generative AI has made remarkable strides in various fields, truly revolutionizing industries such as gaming, healthcare, art, and business. These tables provide a glimpse into the power and impact of this transformative technology. With continued advancements in Generative AI, the possibilities for innovation and creativity seem boundless.
Frequently Asked Questions
What is generative AI?
Generative AI refers to a subset of machine learning techniques that aim to generate new and unique content, such as images, music, or text. It involves training models to learn patterns and generate data that is similar to the examples they were trained on.
How does generative AI work?
Generative AI models typically use deep learning and neural networks to learn patterns from existing data. These models consist of an encoder and a decoder, with the encoder responsible for understanding the input data and the decoder used to generate new content based on the learned patterns.
What are the applications of generative AI?
Generative AI has a wide range of applications. It can be used for tasks such as image synthesis, text generation, music composition, and even video game design. In addition, generative AI can be used for data augmentation and improving the performance of machine learning models.
What is NVIDIA’s role in generative AI?
NVIDIA is a leading company in the field of artificial intelligence and deep learning. They have developed powerful GPU accelerators and software tools that enable researchers and developers to train and deploy generative AI models at scale. NVIDIA’s hardware and software innovations have significantly contributed to the advancements in generative AI.
What are GANs?
Generative Adversarial Networks (GANs) are a popular type of generative AI model. GANs consist of two neural networks: a generator and a discriminator. The generator generates new content, and the discriminator tries to distinguish between the generated content and real data. Through iterative training, GANs can produce increasingly realistic outputs.
How is generative AI different from traditional AI?
Traditional AI focuses on solving predefined tasks using rule-based systems or algorithms. In contrast, generative AI aims to learn patterns from data and generate new content. Traditional AI is typically used for tasks that require explicit instructions, while generative AI can produce novel outputs without explicit programming.
Are there any ethical concerns related to generative AI?
Yes, generative AI raises several ethical concerns. One concern is the generation of deepfake content, which can be used to spread misinformation or deceive people. Another concern is the potential bias that generative AI models may learn from the training data, leading to biased outputs. Ensuring responsible and ethical use of generative AI is crucial to address these concerns.
How can generative AI be used for content creation?
Generative AI models can be trained on a large dataset of existing content and then used to generate new content that is similar in style or quality. For example, an image generation model can be trained on a dataset of landscape photos and then used to create new, realistic landscapes. Similarly, a text generation model can be trained on literary works to produce new stories or poems.
What are some challenges in generative AI?
Generative AI faces several challenges, such as mode collapse, where the generated content becomes repetitive or lacking diversity. Another challenge is understanding and controlling the generated outputs. Ensuring the reliability and interpretability of generative AI models also remains a challenge. Ongoing research and advancements in the field aim to address these challenges.
Where can I learn more about generative AI?
There are various online resources available to learn more about generative AI. NVIDIA’s official website offers detailed documentation and tutorials on their generative AI technologies. Additionally, leading research papers and conferences in the field provide valuable insights into the latest advancements and techniques in generative AI.