Generative AI Medium Blog
In recent years, generative AI has become one of the most exciting and rapidly developing fields in artificial intelligence. This technology, which encompasses deep learning and neural networks, enables computers to generate content such as text, images, and even music. In this article, we will explore the concept of generative AI and its applications across various industries.
Key Takeaways
- Generative AI is a rapidly advancing field in artificial intelligence.
- It enables computers to generate content like text, images, and music.
- Generative AI has applications in various industries, including healthcare, entertainment, and marketing.
Understanding Generative AI
Generative AI refers to the ability of artificial intelligence models to generate new content that closely resembles human-created content. This is accomplished through deep learning techniques, where neural networks are trained on vast amounts of data to learn patterns and create new outputs. Generative AI models include popular algorithms like Generative Adversarial Networks (GANs) and Recurrent Neural Networks (RNNs).
Generative AI technology has garnered significant attention due to its capability to create compelling, high-quality content. An interesting aspect of generative AI is that it can create content that never existed before by imagining new possibilities. This ability opens up a world of opportunities in various industries.
Applications of Generative AI
Generative AI has found applications across a wide range of industries. Here are a few notable examples:
- Healthcare: Generative AI can aid in biomedical research by generating new molecules for drug discovery.
- Entertainment: It can be used in the entertainment industry to generate virtual characters, create realistic environments in video games, and even compose original music.
- Marketing: Generative AI can help businesses generate personalized content for marketing campaigns, improving customer engagement and brand loyalty.
Benefits of Generative AI in Healthcare
Within the healthcare industry, generative AI has several notable benefits:
- Accelerating drug discovery processes by assisting in the creation of new molecules with specific properties.
- Generative AI reduces the time and cost required for experimental testing.
- Improving the accuracy of medical imaging analysis, enabling early and more accurate diagnosis.
Generative AI in the Entertainment Industry
Entertainment has greatly benefited from generative AI technologies:
Application | Description |
---|---|
Virtual Characters | Generative AI can create realistic virtual characters in movies and video games. |
Environment Generation | It can generate complex and realistic virtual environments for various forms of media. |
Music Generation | Generative AI can compose original music pieces, providing new creative possibilities for musicians and composers. |
Marketing Opportunities with Generative AI
Generative AI has revolutionized the way businesses approach marketing:
- Creating Personalized Content: Companies can use generative AI to generate tailored content for each customer, increasing engagement and conversion rates.
- Generative AI allows for rapid content creation and iteration, enabling businesses to keep up with the demands of digital marketing.
- Enhancing User Experience: By analyzing user data, generative AI can provide personalized recommendations and suggestions to improve customer experience.
Future Developments in Generative AI
The field of generative AI continues to evolve rapidly, with constant advancements and breakthroughs. Key areas of ongoing research and development include:
- Improved Text Generation: Researchers are working on developing generative AI models capable of generating more coherent and context-aware text.
- Expanding to Other Modalities: Efforts are being made to extend generative AI capabilities to other domains such as video and speech synthesis.
- Ethics and Regulation: As generative AI becomes more powerful, it raises ethical concerns regarding manipulation and misinformation, prompting the need for regulations.
Generative AI is at the forefront of innovation, revolutionizing industries and pushing the boundaries of what computers can create. With ongoing developments, the potential applications of generative AI are only set to grow.
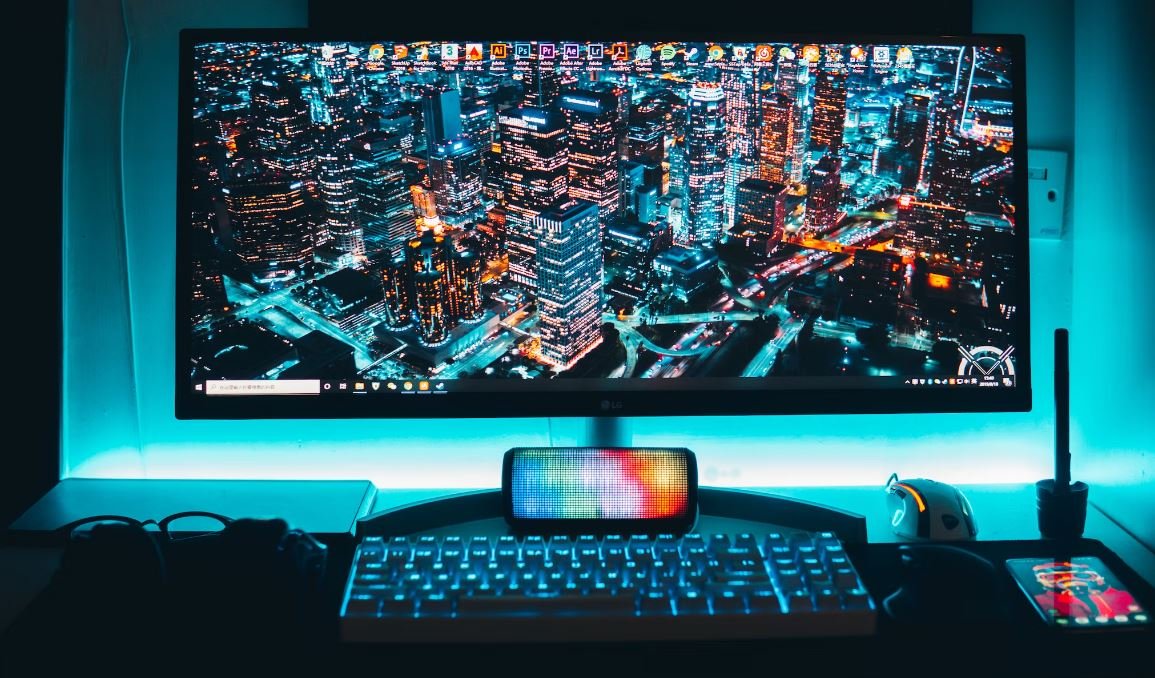
Common Misconceptions
1. Generative AI can replace human creativity
Many people mistakenly believe that generative AI can completely replace human creativity. However, while these AI models have the ability to generate content such as art, music, and writing, they lack the depth and originality that human creativity brings. Some misconceptions about generative AI include:
- Generative AI lacks the emotional and subjective elements that are inherent in human creativity.
- AI-generated content can be repetitive and lack diversity.
- The output of generative AI is limited to what it has been trained on and cannot come up with truly novel ideas.
2. Generative AI is infallible and always produces high-quality content
There is a misconception that generative AI models always produce flawless and high-quality content. However, that is not entirely true. Some common misconceptions related to the quality of generative AI content include:
- Generative AI can produce content that is factually incorrect or misleading if the training data is flawed.
- AI-generated content may still require human intervention and editing to ensure accuracy and coherence.
- Not all generated content will be of high quality, as generative AI models may produce output that is nonsensical or irrelevant.
3. Generative AI poses a significant threat to human employment
There is a widespread belief that generative AI will lead to widespread job losses and make humans obsolete in certain fields. However, while generative AI technology may automate certain tasks, it also creates new opportunities and challenges for humans. Some misconceptions associated with the threat of generative AI on employment include:
- Generative AI works best when combined with human expertise and can augment human capabilities rather than replacing them entirely.
- The rise of generative AI can also lead to the creation of new job roles, such as AI trainers and explainability specialists.
- While some repetitive tasks may be automated, there will still be a need for human creativity, critical thinking, and decision-making skills in many professional domains.
4. Generative AI can easily replicate human emotions and empathy
It is often assumed that generative AI can easily replicate human emotions and empathy in its output. However, this is a misunderstood aspect of AI technology. Some common misconceptions regarding generative AI’s ability to recreate human emotions and empathy are:
- Generative AI lacks the contextual understanding and life experiences that shape human emotions, making it difficult to replicate them accurately.
- The emotional aspects of generative AI-generated content may feel forced or artificial, lacking the genuine empathy and connection that humans can provide.
- While generative AI can mimic certain emotional responses based on data it has been trained on, it does not possess true emotional intelligence or consciousness.
5. Generative AI is inherently biased and unethical
One misconception surrounding generative AI is that it is inherently biased and unethical due to the potential biases contained in its training data. However, biases in AI are a result of human input, not an inherent flaw of the technology itself. Some common misconceptions in this regard include:
- The biases present in generative AI are a reflection of the biases within the data it has been trained on, which can perpetuate existing societal biases.
- Efforts are being made to address biases in training data and ensure fairness and ethical considerations are included in the development and deployment of generative AI models.
- Generative AI can actually help uncover and mitigate biases by enabling analysis and recognition of patterns in vast amounts of data that may be difficult for humans to process.
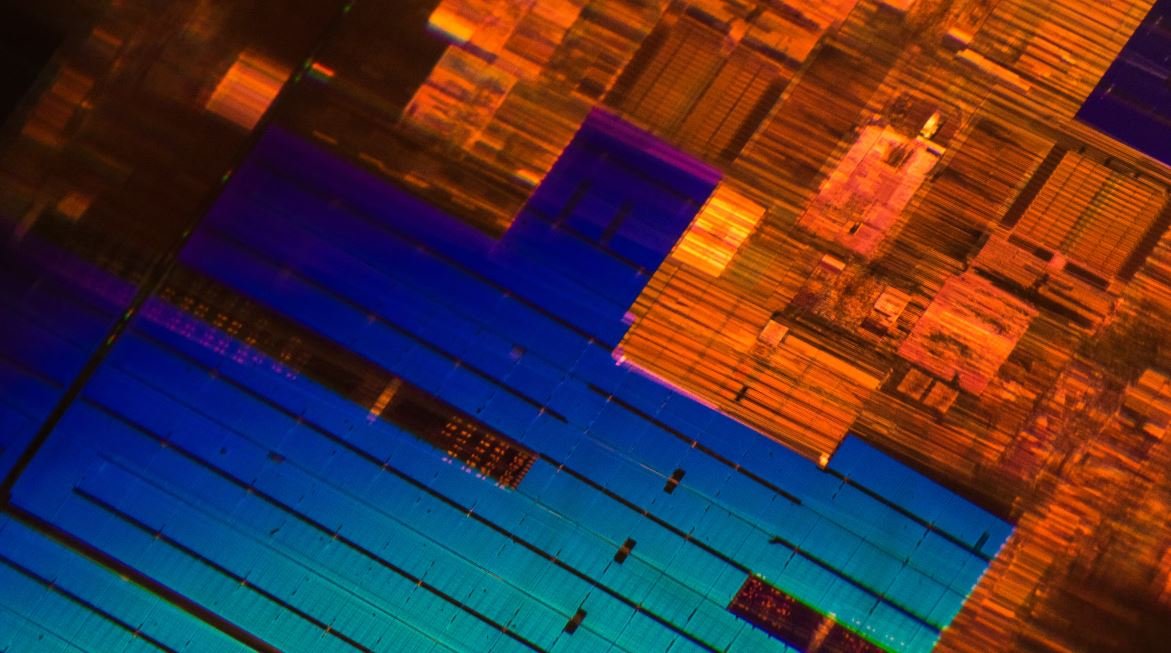
The Rise of Generative AI: A Game-Changer in the Digital World
In recent years, the field of generative AI has witnessed remarkable advancements, revolutionizing various industries. From creating realistic images and videos to generating human-like speech, this cutting-edge technology has opened up new possibilities. The following tables highlight some intriguing facts and applications of generative AI.
The Impact of Generative AI on Industries
Generative AI has significantly disrupted several industries, transforming the way we work and interact. The table below showcases the profound impact of generative AI in three sectors: healthcare, finance, and entertainment.
Industry | Applications | Effects |
---|---|---|
Healthcare | Drug Discovery, Medical Imaging Analysis | Accelerated innovation, improved diagnoses |
Finance | Algorithmic Trading, Fraud Detection | Enhanced financial decision-making, reduced risks |
Entertainment | Virtual Reality, Game Development | Immersive experiences, realistic simulations |
Generative AI Applications in Creative Industries
The creative realm has been greatly influenced by generative AI, empowering artists and designers to break traditional boundaries. Explore the table showcasing how generative AI is reshaping music, art, and fashion.
Creative Domain | Generative AI Applications | Results |
---|---|---|
Music | Composition, Virtual Instruments | Innovative soundscapes, new musical styles |
Art | Procedural Art, DeepArt | Unique artworks, blended human-machine creativity |
Fashion | Design, Customization | Tailored clothing, personalized fashion |
Generative AI’s Ethical Considerations
As generative AI advances, it raises important ethical dilemmas that must be addressed. The table below highlights some key concerns and questions surrounding the deployment of generative AI.
Ethical Considerations | Questions |
---|---|
Authenticity of Generated Content | How do we ensure transparency and prevent misinformation? |
Intellectual Property Rights | Who owns the creative output generated by AI systems? |
Unintended Bias | How can we mitigate biases encoded in AI algorithms? |
Generative AI in Film Industry: Beyond Special Effects
Generative AI has been a game-changer in the film industry, revolutionizing not only visual effects but also the entire creative process. The following table sheds light on the diverse applications of generative AI in filmmaking.
Application | Examples | Impact |
---|---|---|
Character Generation | Virtual actors, creature design | Expands creative possibilities, reduces costs |
Scene Design | Automatic set generation, detailed landscapes | Efficient production, limitless imagination |
Post-production | Automated video editing, visual effects | Time-saving, seamless integration |
Advancements in Natural Language Processing
Natural Language Processing (NLP) powered by generative AI offers sophisticated language understanding and generation capabilities. The table below showcases key milestones in NLP advancements.
Advancement | Description |
---|---|
ChatGPT | Conversational AI model capable of generating human-like text |
BERT | Applying bidirectional training to enhance language understanding |
GPT-3 | Massive language model capable of diverse tasks and creative writing |
Generative AI for Personalized Recommendations
Generative AI enables personalized recommendations, enhancing user experiences and driving engagement. The table below highlights how generative AI algorithms power recommendation systems.
Platform | Generative AI Recommendations | Features |
---|---|---|
Streaming Service | Customized music and video suggestions | Discover new content, tailored playlists |
Online Retailer | Personalized product recommendations | Better shopping experience, increased sales |
Social Media | Targeted content, relevant ads | Improved engagement, monetization |
Generative AI’s Potential in Data Synthesis
Generative AI facilitates data synthesis, generating realistic datasets that accelerate research and development. The table below presents use cases of generative AI in data synthesis.
Use Case | Applications | Benefits |
---|---|---|
Astronomy | Simulating celestial bodies, generating telescope images | Enables astronomical research, reduces observing time |
Autonomous Driving | Generating diverse traffic scenarios, virtual sensor data | Enhances testing, improves safety |
Medical Imaging | Synthesizing realistic MRI and CT scan images | Augments training data, aids diagnosis |
Exploring Generative AI’s Limits: Challenges and Opportunities
While generative AI has made remarkable strides, it faces certain challenges and holds exciting opportunities for future exploration. The table below sheds light on these factors.
Challenges | Opportunities |
---|---|
Limited Dataset Quality | Developing techniques for effective data augmentation |
Uncertainty in Output | Exploring ensemble models and probabilistic approaches |
Ethical Implications | Designing guidelines and frameworks for responsible AI |
Conclusion
Generative AI has revolutionized numerous industries and creative domains, offering unprecedented possibilities for human-machine collaboration. From personalized recommendations to transforming the film industry, it has proven to be a game-changer in the digital world. However, ethical considerations and challenges in data quality and output uncertainty remain pivotal areas to address. As generative AI advances, continued research and responsible development will shape its future trajectory, unlocking even greater potential for innovation and creativity.
Frequently Asked Questions
What is Generative AI?
Generative AI refers to the use of artificial intelligence techniques to create or generate new content, such as images, text, or music, that imitates or resembles human-created content. It involves training models on large amounts of data and utilizing deep learning algorithms to generate creative and original outputs.
How does Generative AI work?
Generative AI utilizes frameworks like neural networks, deep learning algorithms, and machine learning techniques to learn patterns and generate new content. It involves training a model on a dataset and then using the trained model to produce novel outputs based on the learned patterns and insights from the data.
What are the applications of Generative AI?
Generative AI has various applications, including generating realistic images, creating natural language text, composing music, improving data augmentation, and enhancing data synthesis. It can also be used for video synthesis, virtual reality, and content creation in various fields like marketing, entertainment, and design.
What are some popular algorithms used in Generative AI?
Some popular algorithms used in Generative AI include Generative Adversarial Networks (GANs), Variational Autoencoders (VAEs), Recurrent Neural Networks (RNNs), and Transformers. These algorithms are capable of generating realistic and creative outputs based on the training data.
What are the challenges in Generative AI?
Generative AI faces challenges such as mode collapse (limited diversity in generated outputs), training instability, ethical implications, bias in generated content, and the need for high-quality and diverse training data. Addressing these challenges is crucial to ensure the reliability and ethical use of generative models.
Is Generative AI capable of replacing human creativity?
No, Generative AI is not meant to replace human creativity but rather to assist and enhance human creativity. It can provide inspiration and generate novel ideas, but the creative judgment and decision-making still depend on human involvement. Generative AI serves as a tool to stimulate and amplify human creativity.
Are there any ethical concerns associated with Generative AI?
Yes, Generative AI raises ethical concerns related to the potential misuse or generation of manipulated content, deepfakes, copyright infringement, and privacy violations. There is a need for responsible use and regulation to ensure that generative models are not used in harmful or deceptive ways.
What are the future prospects of Generative AI?
The future prospects of Generative AI are vast and exciting. It can amplify creativity, facilitate content generation, enable personalized experiences, and improve data synthesis. It may also find applications in fields like healthcare, virtual reality, and scientific research, contributing to advancements in various domains.
How can one get started with Generative AI?
To get started with Generative AI, one can begin by learning the basics of machine learning and neural networks. Familiarize yourself with popular generative models like GANs and VAEs. Then, experiment and practice by working on small-scale generative projects using frameworks like TensorFlow or PyTorch.
Where can I find more resources to learn about Generative AI?
There are several online resources available to learn more about Generative AI. Websites like Medium, Towards Data Science, and GitHub provide tutorials, articles, and open-source code repositories. Online courses and academic papers are also valuable sources to delve deeper into this field.