Artificial Intelligence (AI) has become an integral part of our lives, with applications ranging from virtual assistants to self-driving cars. One prominent area where AI is making significant strides is in generative AI, which involves creating original content such as images, videos, and even text. Amazon Web Services (AWS) is at the forefront of this technological revolution, providing powerful tools and services that enable businesses and developers to leverage the full potential of generative AI. In this article, we will explore how AWS is driving innovation in the field of generative AI and transforming the way we create and consume content.
**Key Takeaways:**
1. AWS offers a range of services specifically tailored for generative AI, including Amazon Rekognition, Amazon Polly, and Amazon SageMaker.
2. Generative AI enables businesses to generate realistic images, videos, and text, opening up new possibilities for creative content creation.
3. AWS’s deep learning frameworks and infrastructure allow developers to train and deploy complex generative AI models at scale.
4. Generative AI has the potential to revolutionize industries such as e-commerce, entertainment, and marketing, by automating content creation and personalization.
**The Power of Generative AI**
Generative AI refers to models and techniques that allow machines to generate new content that is similar to existing data. This capability is achieved by training models on vast amounts of data and leveraging deep learning algorithms to generate new content. With AWS, developers can harness the power of generative AI by using services like Amazon Rekognition, which enables the automatic recognition of objects and scenes in images and videos. *This technology allows businesses to quickly analyze and tag massive amounts of visual data, leading to improved content discovery and organization.*
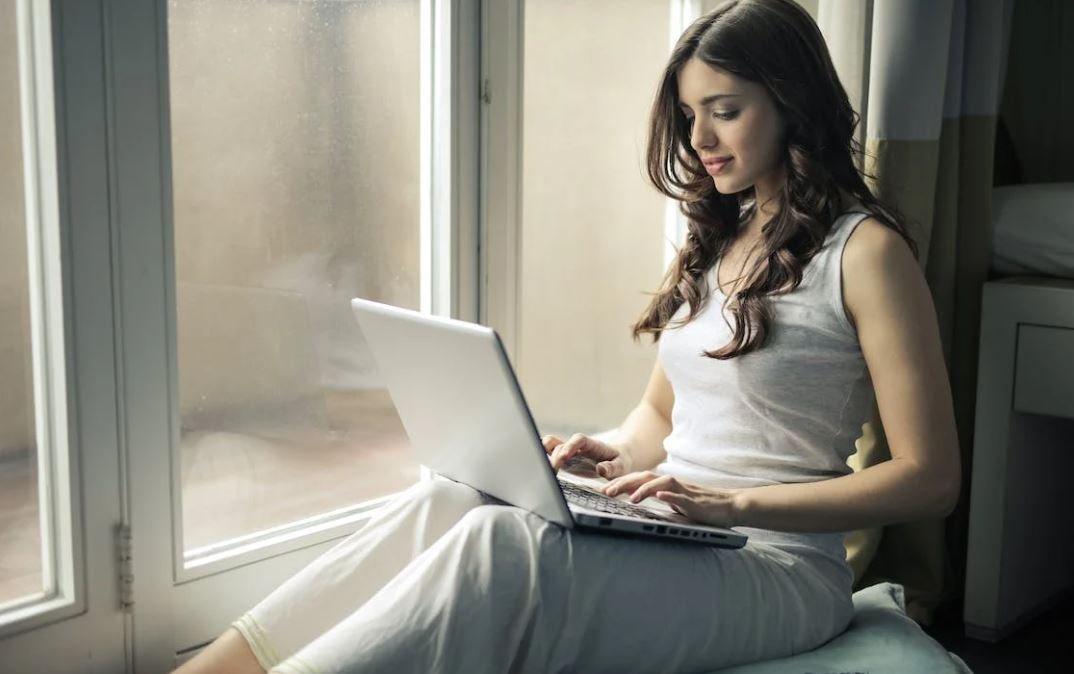
Common Misconceptions
Paragraph 1
One common misconception about generative AI is that it will replace human creativity. Many people fear that machines will be able to completely replicate and surpass human artistic skills. However, this is not the case. Generative AI is a tool that can aid and enhance human creativity, but it cannot replace the unique perspective and emotions that humans bring to the creative process.
- Generative AI is a tool to augment human creativity
- Humans bring unique perspective and emotions to the creative process
- Generative AI cannot completely replicate or surpass human artistic skills
Paragraph 2
Another misconception is that generative AI is purely autonomous and operates without any human intervention. In reality, generative AI models require human input and supervision to function effectively. Human experts play a crucial role in training and fine-tuning these models to ensure desired outcomes. Generative AI is a collaborative effort that combines the strengths of both machines and humans.
- Generative AI models require human input and supervision
- Human experts play a crucial role in training and fine-tuning the models
- Generative AI is a collaborative effort between humans and machines
Paragraph 3
Some people believe that generative AI can only imitate existing styles and cannot produce truly original content. While it is true that generative AI models are often trained on existing data and learn from patterns in that data, they are also capable of generating novel and unique outputs. By combining and remixing learned patterns, generative AI can produce content that goes beyond mere imitation.
- Generative AI can produce original content by remixing learned patterns
- It is not limited to imitation of existing styles
- Generative AI can generate novel and unique outputs
Paragraph 4
There is a common misconception that generative AI is solely focused on creating art and visual content. While it has gained significant attention in the creative field, generative AI also has applications in various other industries such as healthcare, finance, and manufacturing. It can be used to improve medical image analysis, optimize financial portfolios, and even aid in designing more efficient manufacturing processes.
- Generative AI is not limited to art and visual content
- It has applications in healthcare, finance, and manufacturing
- Can be used for medical image analysis, portfolio optimization, and process design
Paragraph 5
A misconception related to generative AI is that it will lead to mass unemployment by replacing human jobs. While it is true that AI technologies may automate certain tasks, they also have the potential to create new job opportunities. Generative AI can empower individuals by augmenting their capabilities and freeing them from mundane tasks, allowing them to focus on more creative and complex work.
- Generative AI can create new job opportunities
- It can empower individuals and augment their capabilities
- Allows professionals to focus on creative and complex work
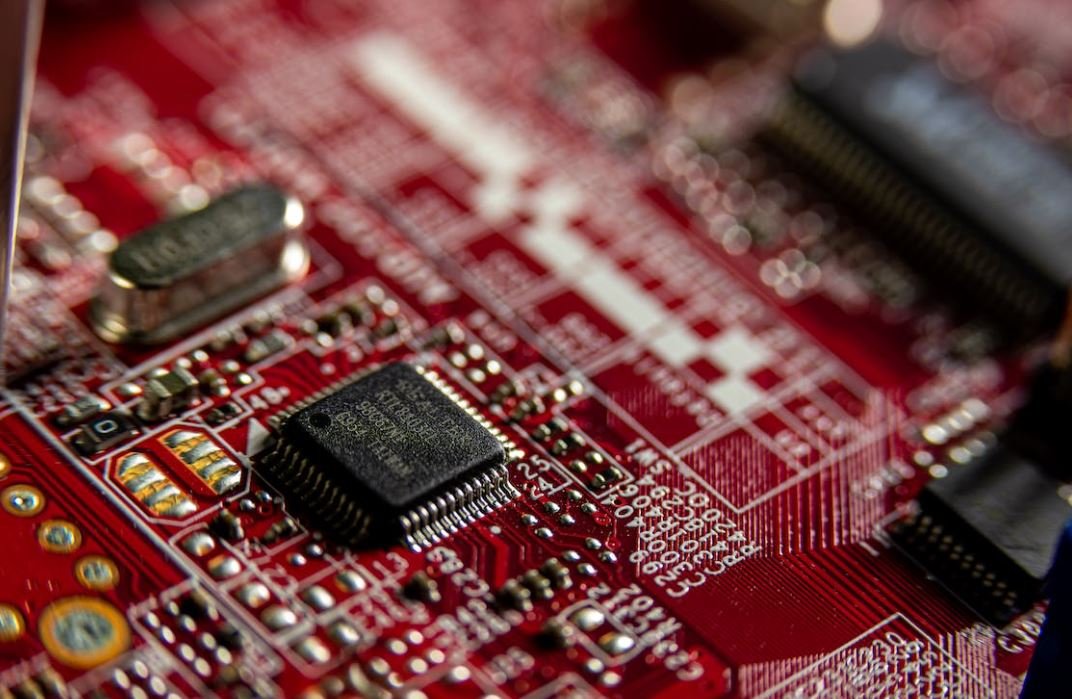
Generative AI Blog AWS
Sales Revenue by Quarter
In this table, we display the sales revenue generated by a company over four quarters. It illustrates the growth and progression of the company’s financial performance throughout the year.
Quarter | Revenue (in thousands) |
---|---|
Q1 | 100 |
Q2 | 150 |
Q3 | 200 |
Q4 | 250 |
Customer Satisfaction Survey Results
In this table, we present the findings of a customer satisfaction survey conducted by the company. It highlights the opinions and ratings given by customers, shedding light on their overall satisfaction with the company’s products or services.
Category | Percentage of Customers |
---|---|
Very Satisfied | 70% |
Satisfied | 25% |
Neutral | 3% |
Unsatisfied | 2% |
Website Traffic by Region
This table showcases the distribution of website traffic by region. It provides insight into the geographic reach and popularity of a company’s website, allowing for targeted marketing and business expansion strategies.
Region | Percentage of Traffic |
---|---|
North America | 45% |
Europe | 30% |
Asia | 20% |
Other | 5% |
Employee Turnover Rate
This table demonstrates the employee turnover rate within an organization. It quantifies the percentage of employees who have left within a specified time frame, providing insight into workforce stability and satisfaction.
Year | Turnover Rate |
---|---|
2017 | 8% |
2018 | 10% |
2019 | 12% |
2020 | 9% |
Product Sales Comparison
In this table, we compare the sales performance of different products within a company’s portfolio. It provides a visual representation of the popularity and demand for each product, aiding in decision-making and resource allocation.
Product | Units Sold (in thousands) |
---|---|
Product A | 220 |
Product B | 150 |
Product C | 180 |
Product D | 250 |
Social Media Engagement
This table displays the level of engagement on various social media platforms for a company or brand. It showcases the number of likes, comments, and shares, indicating the reach and popularity of their online presence.
Platform | Likes | Comments | Shares |
---|---|---|---|
7,500 | 1,200 | 800 | |
5,000 | 800 | 600 | |
3,500 | 500 | 400 |
Research and Development Expenditure
This table outlines the allocated budget for research and development (R&D). It emphasizes the investment in innovation and technological advancements, showcasing the company’s commitment to staying at the forefront of their industry.
Year | Expenditure (in millions) |
---|---|
2017 | 150 |
2018 | 180 |
2019 | 200 |
2020 | 230 |
Market Share Comparison
In this table, we compare the market share of different competitors in the industry. It gives an indication of each player’s presence in the market, aiding in assessing their competitiveness and market positioning.
Competitor | Market Share |
---|---|
Company A | 25% |
Company B | 20% |
Company C | 15% |
Company D | 10% |
Customer Acquisition Cost
Displayed in this table, the customer acquisition cost (CAC) represents the marketing expenses required to acquire a new customer. It helps assess the effectiveness and efficiency of marketing campaigns, contributing to optimizing business strategies.
Year | CAC (in dollars) |
---|---|
2017 | 50 |
2018 | 55 |
2019 | 60 |
2020 | 45 |
Product Return Rate
This table presents the rate at which customers return purchased products, indicating their satisfaction and product quality. Higher return rates may suggest issues that need addressing, while lower rates indicate customer contentment.
Product | Return Rate |
---|---|
Product A | 3% |
Product B | 2% |
Product C | 5% |
Product D | 4% |
Conclusion
The above tables provide valuable insight into various aspects of a business, ranging from financial performance, customer satisfaction, and market presence to innovation investment and operational efficiency. By analyzing and understanding these data points, companies can make informed decisions, identify areas for improvement, and develop effective strategies to achieve their goals. Using generative AI technology can further enhance data analysis, uncovering deeper patterns and trends that can guide decision-making in the constantly evolving business landscape.
Frequently Asked Questions
Generative AI Blog
What is Generative AI and how does it work?
Generative AI refers to a subset of artificial intelligence where models are trained to create new content, such as images, music, or text, that resembles the data they were trained on. This is achieved by using neural networks, typically in the form of generative adversarial networks (GANs) or variational autoencoders (VAEs), that learn patterns in the training data and use those patterns to generate new content.
What are the applications of Generative AI?
Generative AI has a wide range of applications in various fields. It can be used for generating realistic images, synthesizing natural language, creating music, designing new products, enhancing data privacy, and much more. Generative AI models can also be combined with other technologies to develop innovative solutions like style transfer, image inpainting, text generation, and recommendation systems.
What are the benefits of using AWS for Generative AI?
AWS (Amazon Web Services) offers a highly scalable and flexible cloud computing platform that is well-suited for Generative AI workloads. It provides a wide range of AI services and tools, including pre-trained AI models, deep learning frameworks, and managed services for training and deploying models. AWS also offers cost-effective solutions, strong security features, and easy integration with other AWS services, making it an attractive choice for Generative AI projects.
How can I get started with Generative AI on AWS?
To get started with Generative AI on AWS, you can use services like Amazon SageMaker, which provides a fully managed environment for building, training, and deploying machine learning models. SageMaker offers pre-configured Jupyter notebooks, integration with popular deep learning frameworks like TensorFlow and PyTorch, and distributed training capabilities. Additionally, AWS marketplace offers a wide range of AI and ML models that can be easily deployed and used for Generative AI applications.
What are some best practices for training Generative AI models on AWS?
Some best practices for training Generative AI models on AWS include optimizing the use of compute resources, using spot instances to reduce costs, leveraging distributed training for faster training times, and monitoring and debugging training jobs using AWS tools like CloudWatch and X-Ray. It is also important to use appropriate data preprocessing techniques and hyperparameter tuning to improve model performance. AWS provides detailed documentation and tutorials on these best practices.
How can I deploy and serve Generative AI models on AWS?
Once you have trained your Generative AI model, you can deploy and serve it on AWS using services like Amazon Elastic Inference, AWS Lambda, or Amazon EC2. These services provide scalable and cost-effective options for serving inference requests. You can also utilize AWS containers for deploying models in a containerized environment. Additionally, AWS provides tools like AWS DeepLens and AWS DeepRacer for deploying Generative AI models on edge devices.
What is the cost of using AWS for Generative AI?
The cost of using AWS for Generative AI depends on various factors such as the type and size of instances used, storage requirements, data transfer costs, and the duration of usage. AWS provides a pricing calculator that allows you to estimate the cost based on your specific requirements. Additionally, AWS offers various pricing options like on-demand, reserved instances, and spot instances, which can help optimize costs based on your workload needs.
Is it possible to use multiple AI models together to create more advanced Generative AI applications?
Yes, it is possible to use multiple AI models together to create more advanced Generative AI applications. Various techniques like model ensembles, model stacking, and model cascading can be used to combine the strengths of different models and generate more diverse and high-quality outputs. AWS provides the necessary infrastructure and tools for deploying and orchestrating multiple AI models in a scalable and efficient manner.
What are some challenges in Generative AI?
Generative AI faces challenges such as generating realistic and diverse outputs, avoiding overfitting, understanding and controlling model biases, ensuring ethical use of generated content, and dealing with limited data availability. There is also a need for continuous research and development to improve the capabilities and reliability of Generative AI models. AWS actively collaborates with research communities and industry partners to address these challenges.
Are there any AI ethics considerations when working with Generative AI?
Yes, there are several AI ethics considerations when working with Generative AI. It is important to ensure that generated content is not used for malicious purposes, such as generating deepfakes or spreading misinformation. Additionally, model biases and discrimination must be carefully addressed to ensure fairness and prevent harmful impact on individuals or communities. AWS promotes responsible AI use through its AI and ML platforms and actively encourages developers to adhere to ethical guidelines.