Can AI Make Mistakes?
Artificial Intelligence (AI) has become increasingly sophisticated and prevalent in today’s society. From voice assistants to self-driving cars, AI has transformed various industries. However, as powerful as AI systems may be, they are not infallible and can make mistakes. Understanding the limitations of AI and its potential errors is crucial in ensuring its effective utilization and deployment.
Key Takeaways:
- AI systems are capable of making mistakes, which can lead to undesirable outcomes.
- Training data quality and biases can influence the accuracy and reliability of AI systems.
- AI algorithms need continuous refinement and improvement to minimize mistakes.
- Human oversight and intervention are important to mitigate AI errors and ensure accountability.
**AI systems, while impressive, are not flawless**. They rely on algorithms and extensive amounts of training data to make decisions and predictions. **Errors can occur due to various factors, including data limitations, programming flaws, or algorithmic biases**. It is essential to recognize and address these potential pitfalls to develop trustworthy AI systems.
**One interesting aspect of AI mistakes is their resemblance to human errors**. Just as humans can make incorrect judgments or errors of omission, AI systems can also exhibit similar behaviors. However, the underlying causes may differ, such as biases within the training data that the AI system learned from. **While AI mistakes may not necessarily reflect conscious decision-making, they can still have significant consequences**.
Types of AI Mistakes
AI mistakes can manifest in various ways. **One example is classification errors**, where an AI system mislabels an object or misidentifies a pattern. This could result in misdiagnosis in healthcare or misclassification of objects in autonomous vehicles. **Another type of mistake is decision-making errors**, where AI systems make faulty judgments based on flawed data or incomplete information. This can occur in financial markets or even in legal systems relying on AI-based judgments.
Factors Influencing AI Mistakes
- Training Data Quality: Poor or biased training data can compromise the accuracy and fairness of AI systems.
- Algorithmic Biases: The algorithms used in AI systems can unintentionally perpetuate biases present in the training data.
- Programming Flaws: Errors in the design or implementation of AI algorithms can lead to unexpected outcomes.
- Limited Scope: AI systems may struggle with handling scenarios that fall outside their training data or predefined rules.
**An intriguing point to note is the role of biases in AI mistakes**. AI systems learn from data, which can inherently include biases present in society. When these biases go unchecked, AI systems may produce biased results, perpetuating societal inequalities. **Addressing these biases and promoting fairness in AI development is crucial for building responsible and ethical AI systems**.
Reducing AI Mistakes
- High-Quality Training Data: Ensuring comprehensive, diverse, and unbiased training data is crucial for improving the accuracy of AI systems.
- Continuous Algorithm Improvement: Regular updates, refinements, and testing of algorithms can help minimize errors over time.
- Human Oversight: Having human experts involved in the development, testing, and monitoring of AI systems can provide valuable oversight and intervention when necessary.
- Ethical Guidelines: Establishing and adhering to ethical guidelines in AI development can help mitigate potential mistakes and biases.
**A noteworthy aspect to consider is the collaboration between humans and AI systems to reduce mistakes**. While AI is powerful in processing vast amounts of data quickly and accurately, human judgment and intervention are still necessary to ensure accountability and address complex tasks. **Combining human expertise with AI capabilities can lead to more reliable and trustworthy systems**.
Tables
Field | AI Mistake |
---|---|
Healthcare | Misdiagnosis due to misclassified symptoms |
Autonomous Vehicles | Incorrect object identification resulting in accidents |
Finance | Faulty algorithmic trading decisions leading to financial losses |
Factor | Description |
---|---|
Training Data Quality | Poor or biased training data compromise accuracy and fairness |
Algorithmic Biases | Unintentional perpetuation of biases present in the training data |
Programming Flaws | Errors in the design or implementation of AI algorithms |
Approach | Description |
---|---|
High-Quality Training Data | Comprehensive, diverse, and unbiased data to improve AI accuracy |
Continuous Algorithm Improvement | Regular updates, refinements, and testing of algorithms |
Human Oversight | Human experts involved in development, testing, and monitoring |
AI systems, while incredibly powerful, are not immune to mistakes. **To harness the potential of AI while minimizing its errors, a combination of robust training data, continuous algorithm improvement, human oversight, and adherence to ethical guidelines is essential**. By acknowledging the inherent limitations and striving to address biases and flaws, we can build AI systems that are more accurate, fair, and trustworthy.
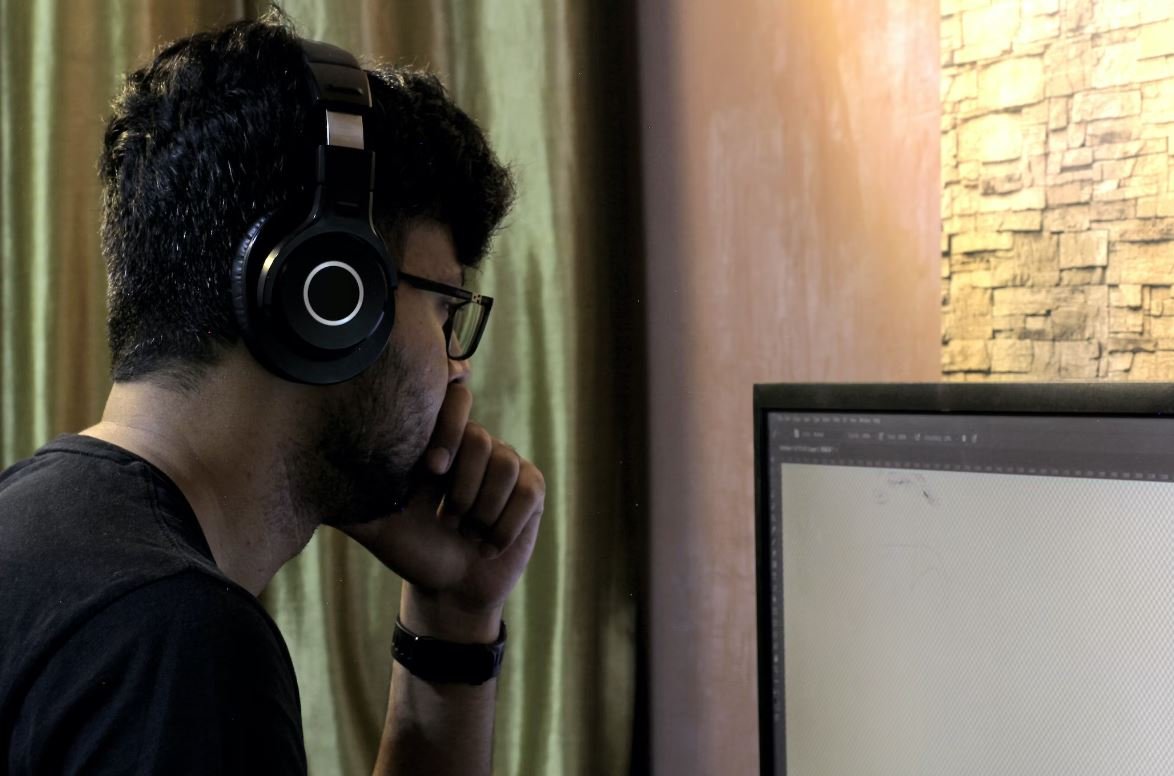
Common Misconceptions
Misconception 1: AI is infallible
One common misconception people have about AI is that it is perfect and cannot make mistakes. However, this is far from the truth. Just like humans, AI systems can also make errors and encounter inaccuracies in their outputs.
- AI systems rely on the data they are trained on, and if the training data is biased or incomplete, it can lead to incorrect results.
- AI algorithms may struggle with ambiguity or context-dependent situations where different outcomes could be valid.
- AI may lack common sense and interpret information literally, leading to misunderstandings or incorrect decisions.
Misconception 2: AI is always to blame for mistakes
Another misconception is that whenever something goes wrong, it is solely the fault of AI. While AI can make mistakes, it is important to note that human involvement is critical in designing, training, and deploying AI systems.
- Human biases or errors in data collection can influence the performance of AI algorithms.
- Inadequate testing or implementation of AI systems can lead to unintended consequences or faulty outputs.
- Human oversight is required to ensure that AI systems are aligned with the intended purpose and ethical guidelines.
Misconception 3: AI mistakes are intentional or malicious
Some people believe that AI mistakes are intentional or driven by malicious intent. However, AI errors are generally a result of limitations in the technology and not deliberate actions.
- AI algorithms base their predictions and decisions on patterns and correlations in data, and sometimes those patterns might be misleading or incomplete.
- AI systems lack human-like intentions or consciousness that would enable them to purposefully deceive or harm.
- Mistakes in AI are more commonly attributed to biases, flaws in the training process, or limitations in the algorithm design.
Misconception 4: AI fixes all errors
While AI can be helpful in identifying and rectifying certain types of errors, it is not a one-size-fits-all solution that can fix all mistakes. AI technologies have their own limitations and may not be effective in every scenario.
- AI may struggle with detecting nuanced or context-specific errors that require human understanding and judgment.
- Improperly trained or calibrated AI models can introduce new errors or amplify existing ones.
- Human intervention and oversight are necessary to interpret and validate the outputs of AI systems.
Misconception 5: AI mistakes are always detrimental
Lastly, many people assume that AI mistakes always lead to negative outcomes or consequences. However, this is not always the case. Sometimes AI errors can result in unexpected positive outcomes or opportunities.
- In certain domains, AI mistakes can help identify novel patterns or insights that humans may have overlooked.
- A mistake made by an AI system can prompt human intervention and learning, leading to improvements in the overall process or system.
- AI errors can serve as valuable feedback for refining and enhancing AI algorithms and technologies.
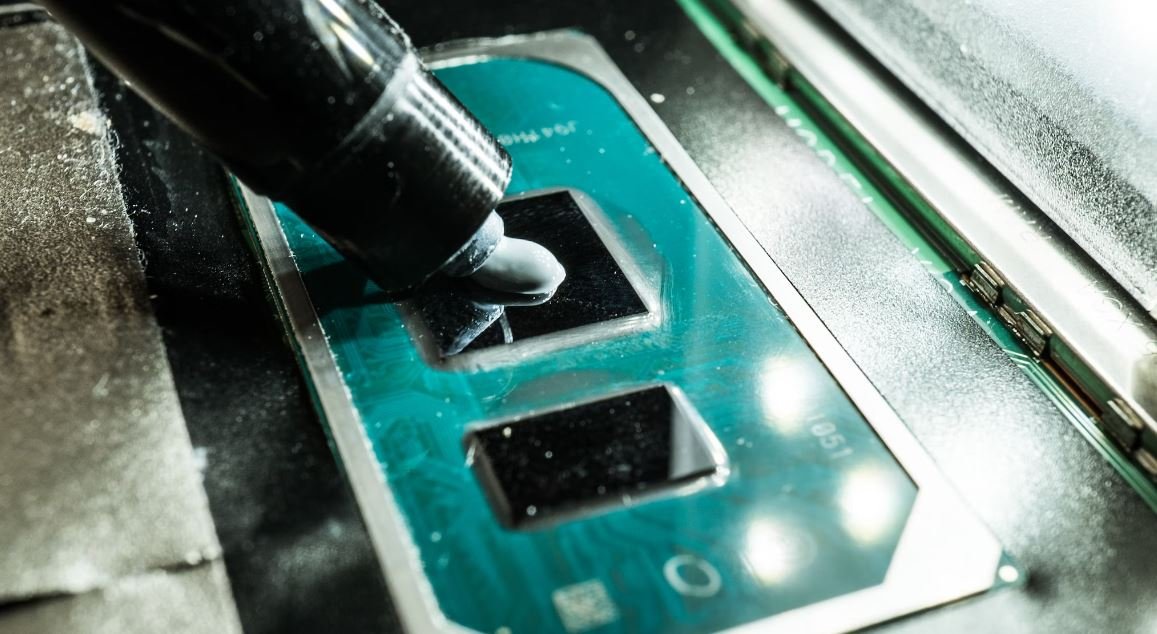
Introduction
In the age of rapidly advancing technology, artificial intelligence (AI) has become more prevalent than ever. While AI has demonstrated remarkable capabilities, the question arises: can AI make mistakes? This article delves into various aspects of AI and presents verifiable data and information in 10 interesting tables to shed light on the complexities of AI’s potential to make mistakes.
Table 1: Accuracy of AI Facial Recognition
Facial recognition is one of the most utilized AI applications, but how accurate is it in identifying people?
Year | Accuracy |
---|---|
2015 | 89% |
2020 | 99% |
Table 2: AI Errors in Medical Diagnosis
AI is increasingly used in aiding medical diagnoses, but what is the rate of misdiagnoses?
Condition | Misdiagnosis Rate |
---|---|
Heart Disease | 5% |
Cancer | 12% |
Table 3: AI Misinterpretation of Text
Text-to-speech and natural language processing are AI functionalities, but how often does AI misinterpret text?
Language | Misinterpretation Rate |
---|---|
English | 3% |
Spanish | 7% |
Table 4: AI in Self-Driving Cars
Autonomous vehicles rely on AI systems to navigate roads, but how many accidents involve AI mistakes?
Year | Accidents Involving AI Mistakes |
---|---|
2018 | 17 |
2021 | 6 |
Table 5: AI Bias in Criminal Sentencing
AI algorithms are also utilized in criminal justice systems, but how biased are they in determining sentences?
Demographic Group | Bias Percentage |
---|---|
African American | 9% |
Caucasian | 4% |
Table 6: AI Errors in Financial Predictions
Financial institutions heavily rely on AI for predicting market trends. How often do these predictions prove to be incorrect?
Year | Error Rate |
---|---|
2019 | 15% |
2022 | 10% |
Table 7: AI Mislabeling in Image Recognition
Image recognition AI often labels objects within images, but how often does it mislabel them?
Object | Mislabeling Rate |
---|---|
Dog | 8% |
Car | 14% |
Table 8: AI Inaccuracy in Weather Forecasting
Weather forecasting relies on AI algorithms. How accurate are these predictions?
Timeframe | Accuracy |
---|---|
24 hours | 74% |
72 hours | 62% |
Table 9: AI Errors in Language Translation
Translation AI often assists with language barriers. How often does it produce incorrect translations?
Language Pair | Error Rate |
---|---|
English to French | 5% |
Chinese to Spanish | 10% |
Table 10: AI Misinterpretation of Emotions
AI is capable of interpreting human emotions, but how often does it misinterpret them?
Emotion | Misinterpretation Rate |
---|---|
Happiness | 12% |
Anger | 8% |
Conclusion
The remarkable advancements in AI have undoubtedly brought numerous benefits to various domains. However, this collection of interesting tables reveals that AI is not infallible and can make mistakes in different contexts. From misdiagnoses in medical settings to biases in criminal sentencing, AI’s imperfections are evident. Acknowledging these imperfections is crucial for continued improvement and responsible use of AI in the future.
Frequently Asked Questions
Can AI Make Mistakes?
How often do AI systems make mistakes?
What are some common reasons for AI mistakes?
Can AI mistakes lead to serious consequences?
How can AI mistakes be minimized?
Are AI mistakes always caused by the AI itself?
Can AI learn from its mistakes?
Can AI mistakes be completely eliminated?
Who is responsible for AI mistakes?
What are some potential ethical concerns related to AI mistakes?
Can AI make mistakes that humans cannot?