AI vs ANN: Understanding the Differences
Artificial Intelligence (AI) and Artificial Neural Networks (ANN) are both technologies that have revolutionized various fields, from healthcare to finance. While they are often used interchangeably, it’s important to understand that there are key differences between the two. In this article, we will explore the distinctions between AI and ANN, their applications, and their impact on society.
Key Takeaways:
- AI and ANN are not synonymous; AI is a broad concept that encompasses ANN as a specific subset.
- AI focuses on creating intelligent systems that mimic human thinking and decision-making processes, while ANN aims to simulate the functioning of the human brain.
- ANN relies on a complex network of interconnected nodes, called neurons, to process and analyze data.
- AI has far-reaching applications, including speech recognition, computer vision, natural language processing, and autonomous vehicles.
- ANN has shown remarkable success in tasks such as image recognition, pattern detection, and prediction.
Artificial Intelligence, a term coined in the 1950s, encompasses a range of technologies that enable machines to perform tasks that typically require human intelligence. **AI encompasses both machine learning and deep learning techniques**, which leverage algorithms and statistical models to learn from data and make predictions or decisions. *Machine learning, a core component of AI, allows systems to learn from experience without being explicitly programmed*. On the other hand, **Artificial Neural Networks consist of multiple interconnected layers of nodes inspired by the structure of the human brain**. These networks are designed to recognize patterns, process complex data, and make intelligent decisions. *ANN is a specialized technique within AI that mimics the human brain’s ability to learn and adapt*.
Applications of AI and ANN:
AI has gained significant traction in various sectors due to its diverse applications. The following are some notable areas where AI is making strides:
- **Speech Recognition**: AI-driven systems, such as virtual assistants, enable voice commands and natural language interactions.
- **Computer Vision**: AI enables machines to analyze visual information, enabling applications such as image recognition and object detection.
- **Natural Language Processing**: AI techniques allow computers to understand and generate human language, facilitating tasks like text analysis and language translation.
- **Autonomous Vehicles**: AI is driving the development of self-driving cars and autonomous drones, revolutionizing the transportation industry.
- **Robotic Process Automation**: AI automates repetitive tasks, freeing up time for humans to focus on more complex and creative endeavors.
While AI encompasses a wide range of techniques, ANN has shown remarkable success in specific applications:
AI vs ANN: A Comparative Analysis
Criteria | Artificial Intelligence | Artificial Neural Networks |
---|---|---|
Processing Power | Relatively lower processing power requirement as the focus is more on algorithm design. | Requires high computational power due to deep learning architectures and complex data processing. |
Learning Capabilities | Can learn from both structured and unstructured data through supervised, unsupervised, and reinforcement learning techniques. | Capable of self-learning and feature extraction, making it excellent for pattern recognition tasks. |
Training Time | Varying training time depending on the complexity of the AI model and the size of the dataset. | Training deep neural networks can be time-consuming, especially with large-scale datasets; however, transfer learning techniques can accelerate the process. |
Interpretability | AI models can be hard to interpret due to their complex algorithms, which limits explainability. | ANN models lack explainability, making it challenging to understand the decision-making process. |
Despite these differences, both AI and ANN are transforming industries and disrupting traditional business processes. Whether it’s AI-powered intelligent conversational agents, fraud detection systems, or medical diagnostic tools based on ANN, these technologies are shaping the way we live and work.
As AI and ANN continue to evolve rapidly, further advancements and applications are on the horizon. The potential of these technologies is immense, and their impact on society cannot be overlooked. Embracing these innovations will unlock new opportunities and challenges, ultimately reshaping the future.
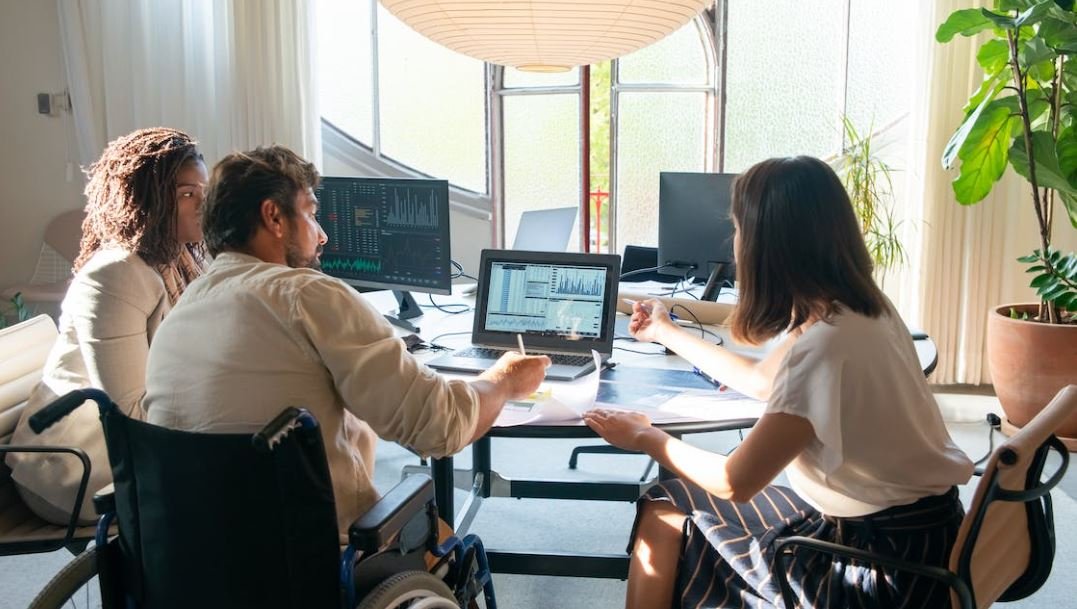
Common Misconceptions
Misconception 1: AI and ANN are the same
One common misconception people have is that Artificial Intelligence (AI) and Artificial Neural Networks (ANN) are the same thing. While ANN is a subset of AI, AI is a broader concept that encompasses various techniques and algorithms that enable machines to simulate human intelligence and perform tasks. ANN, on the other hand, refers specifically to a type of machine learning model inspired by the biological neural network of the human brain.
- AI is not limited to neural networks.
- Several other AI techniques exist, such as genetic algorithms and expert systems.
- ANN is just one approach within the larger field of AI.
Misconception 2: AI and ANN can think and reason like humans
Another misconception is that AI and ANN can achieve human-like thinking and reasoning. While AI systems can process vast amounts of data and perform complex tasks, they do not possess the same level of cognitive abilities as humans. AI systems rely on predefined rules and patterns to make decisions, while human thinking and reasoning are influenced by emotions, intuition, and prior knowledge that is difficult to replicate in machines.
- AI systems lack human-like consciousness and self-awareness.
- Human cognition incorporates subjective experiences and emotions.
- ANN lacks the capacity for creativity and abstract reasoning seen in human intelligence.
Misconception 3: AI and ANN will take over human jobs
There is a prevailing fear that AI and ANN will replace human workers, leading to higher unemployment rates. While it is true that AI has the potential to automate certain tasks and roles, it is unlikely to fully replace human workers across all industries. AI technologies are better suited for augmenting human capabilities, enhancing productivity, and streamlining processes rather than completely substituting human labor.
- AI can empower humans by automating repetitive and mundane tasks.
- New jobs and roles will emerge in the field of AI and related technologies.
- Human skills such as creativity, empathy, and critical thinking remain crucial and cannot be replicated by AI systems.
Misconception 4: AI and ANN are infallible
Many believe that AI and ANN are flawless and always provide accurate results. However, these technologies are not free from errors and limitations. AI models heavily rely on the quality of data they are trained on, and biases or errors in the data can lead to biased or inaccurate outcomes. Additionally, AI models may struggle in novel or ambiguous situations where they lack sufficient training data.
- AI models can exhibit biases, reflecting the biases in the training data.
- High-quality and diverse training data is essential for accurate AI models.
- AI models may struggle in adapting to unforeseen or unique scenarios.
Misconception 5: AI and ANN are only for tech companies
Some people believe that AI and ANN are exclusively relevant to the tech industry. While AI has significant applications in the tech sector, its potential extends to a variety of other industries as well. AI can be utilized in healthcare to improve diagnostics, in finance for fraud detection, in transportation for autonomous vehicles, and in many other sectors where complex data analysis and decision-making are required.
- AI has the potential to transform diverse industries beyond tech.
- Healthcare, finance, transportation, and manufacturing are among the sectors benefitting from AI.
- AI has the capability to revolutionize various aspects of our daily lives.
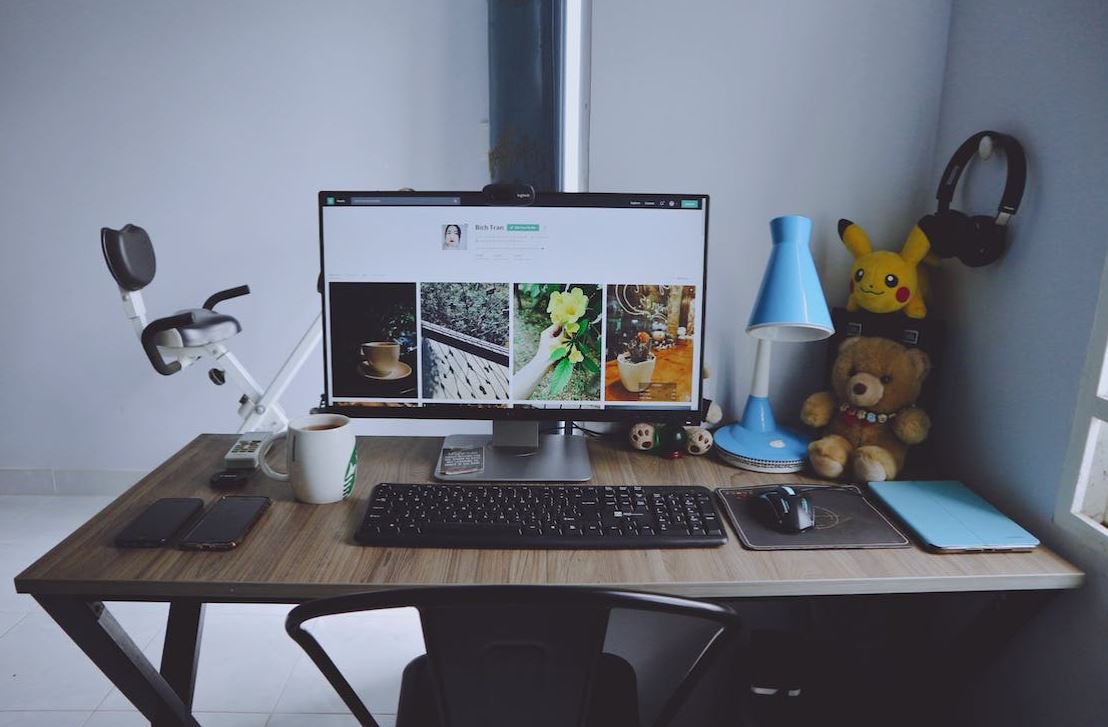
Introduction
In recent years, the fields of Artificial Intelligence (AI) and Artificial Neural Networks (ANN) have gained significant attention due to their ability to mimic human intelligence and solve complex problems. While AI refers to the broad concept of creating machines that can perform tasks requiring human-like intelligence, ANN is a specific algorithmic model of AI inspired by the structure and functioning of the human brain. This article explores various aspects of AI and ANN and highlights their similarities and differences through a series of engaging tables.
Table 1: Applications of AI
Artificial Intelligence has found applications in numerous fields, from healthcare to finance and entertainment. Below are some noteworthy examples:
| Field | Application |
|—————-|———————————————————————————————————|
| Healthcare | AI is used to predict diseases, diagnose patients, and develop personalized treatment plans. |
| Finance | AI algorithms assist with fraud detection, trading analytics, and credit scoring. |
| Sports | AI is utilized for performance analysis, injury prevention, and providing real-time game intelligence. |
| Transportation | Self-driving cars, traffic prediction, and optimized route planning are few applications of AI in this domain. |
Table 2: Advantages of AI
AI brings numerous benefits to various industries. Here are some advantages:
| Advantages | Explanation |
|————————————————-|——————————————————————————————–|
| Efficiency | AI systems can perform repetitive tasks faster and without fatigue, increasing productivity. |
| Decision-making | AI algorithms can analyze vast amounts of data and make more accurate and informed decisions.|
| Personalization | AI enables the customization of products/services based on individual preferences and needs.|
| Safety and risk reduction | AI can be used to detect potential dangers, prevent accidents, and manage risks effectively. |
Table 3: Examples of ANN
Artificial Neural Networks are a type of AI model that utilize interconnected nodes resembling neurons. They have been successfully applied to various tasks:
| Task | Examples |
|————————|———————————————————————————————————|
| Image Recognition | ANN models such as Convolutional Neural Networks (CNNs) are used for object and facial recognition. |
| Natural Language Processing | Recurrent Neural Networks (RNNs) enable language translation, sentiment analysis, and chatbots. |
| Financial Forecasting | ANN can predict stock market trends, analyze trading patterns, and optimize investment portfolios. |
| Medical Diagnosis | ANN assists in diagnosing diseases, analyzing medical images, and predicting treatment outcomes. |
Table 4: Similarities between AI and ANN
Although AI is a broad concept and ANN is a specific model within AI, there are several similarities between the two:
| Similarities | Explanation |
|—————————————-|——————————————————————————————–|
| Machine Learning Algorithms | Both AI and ANN involve the use of machine learning algorithms to extract patterns from data. |
| Ability to Learn and Adapt | AI and ANN can learn from experience, adapt to new scenarios, and improve their performance. |
| Problem-solving Capabilities | Both AI and ANN possess problem-solving abilities that can tackle complex and diverse tasks. |
| Data-driven Decision Making | AI and ANN base their decisions and predictions on the analysis of large volumes of data. |
Table 5: Differences between AI and ANN
While AI incorporates various subfields and techniques, ANN represents a specific approach. Here are some differences:
| Differences | Explanation |
|———————————————|—————————————————————————————————|
| Breadth of Applications | AI has a broader scope and can include various techniques beyond ANN, such as expert systems or NLP.|
| Neural Network Structure | ANN is structured as interconnected layers of nodes, whereas not all AI models follow this setup. |
| Training and Learning Methods | ANN relies on backpropagation and supervised learning, whereas AI encompasses different learning approaches and algorithms.|
| Complexity and Compute Requirements | ANN can be computationally intensive depending on the complexity of the network and training data, while AI models may have varying levels of complexity. |
Table 6: AI in Popular Media
AI has fascinated people for decades, explored widely in various forms of media, from movies to literature:
| Medium | Popular Examples |
|————-|—————————————————————|
| Movies | Blade Runner, Ex Machina, The Matrix, Her |
| Literature | 1984, Brave New World, Do Androids Dream of Electric Sheep? |
| Television | Westworld, Black Mirror, Humans, Person of Interest |
| Video Games | Portal, Deus Ex, Detroit: Become Human, The Last of Us Part II |
Table 7: Ethical Concerns in AI
As AI continues to advance, ethical considerations become critical. Here are some key concerns:
| Ethical Concerns | Explanation |
|———————————————-|————————————————————————————————|
| Bias in Algorithmic Decision-Making | AI systems can unintentionally embed biases, leading to unfair outcomes and reinforced inequalities. |
| Privacy and Data Protection | The collection and use of personal data raise concerns about privacy, consent, and data security. |
| Autonomous Weapons and Warfare | AI-powered weapons possess risks related to accountability, human control, and potential misuse. |
| Impact on Employment and Workforce | AI automation may disrupt job markets and require strategies to ensure a just transition for workers. |
Table 8: Industry Leaders in AI
Several companies and organizations have become pioneers and leaders in AI research and implementation:
| Organization | Notable Contributions |
|————————|———————————————————————————————————–|
| Google | Develops advanced AI models, such as AlphaGo, and integrates AI technologies into various products/services.|
| OpenAI | Conducts research to ensure safe and beneficial AI advances while releasing models like GPT-3 and DALL-E. |
| Microsoft | Utilizes AI across its products, focusing on areas like cloud services, personal assistants, and gaming. |
| DeepMind (acquired by Google) | Known for creating AI systems that achieve remarkable performance in chess, Go, and other complex games. |
Table 9: AI and ANN Career Opportunities
A career in AI and ANN offers diverse opportunities across multiple sectors:
| Industry | Career Opportunities |
|————————-|——————————————————————————————-|
| Healthcare | AI-powered medical imaging, precision medicine, telemedicine, and health chatbot development.|
| Finance | Algorithmic trading, risk assessment, fraud detection, and automated customer service. |
| Robotics | Development of autonomous robots, industrial automation, and unmanned aerial vehicles. |
| Gaming and Entertainment| Game AI programming, virtual reality experiences, character animation, and speech recognition.|
Conclusion
Artificial Intelligence and Artificial Neural Networks represent captivating fields with tremendous potential for innovation and transformation. While AI encompasses a broader concept of creating intelligent machines, ANN stands as a specific model within AI that mimics the neural structure of the human brain. Both AI and ANN have brought significant advancements in various domains, allowing us to solve intricate problems, enhance decision-making, and expand the boundaries of human capability. As the world continues to embrace AI and ANN, appropriately addressing ethical concerns and creating a collaborative environment between humans and machines will be crucial for a beneficial and inclusive future.
Frequently Asked Questions
FAQ 1: What is the difference between AI and ANN?
AI, or Artificial Intelligence, refers to the broader field of computer science that aims to create intelligent machines capable of simulating human-like behavior. ANN, or Artificial Neural Network, is a specific type of AI model inspired by the neural networks in the human brain. While AI encompasses various methodologies, ANN specifically focuses on mimicking the way neurons interconnect and process information.
FAQ 2: How does an Artificial Neural Network work?
An Artificial Neural Network is composed of multiple interconnected nodes, known as artificial neurons or units. These units receive inputs, apply weights to them, perform calculations, and produce outputs. The network then uses these outputs as inputs for subsequent layers, leading to the final desired output. Through a process called training, where the network adjusts the weights based on known inputs and desired outputs, an ANN can learn and generalize patterns.
FAQ 3: What are the advantages of using AI?
AI brings several advantages, such as automation of repetitive tasks, increased efficiency and productivity, improved decision-making based on data analysis, the ability to handle complex and large-scale data, and the potential for breakthroughs in various fields, including healthcare, finance, transportation, and more. AI has the ability to enhance processes, discover insights, and offer innovative solutions.
FAQ 4: What are the limitations of Artificial Neural Networks?
While ANNs are powerful, they also have some limitations. Training an ANN can be time-consuming and computationally intensive, especially for large and complex architectures. Overfitting, where the network performs well on training data but fails to generalize to new data, is another challenge. ANNs may also require a significant amount of labeled data for effective training, and their inner workings can be difficult to interpret or explain, leading to a lack of transparency.
FAQ 5: Can AI replace human intelligence?
No, AI is designed to augment human intelligence rather than replace it. While AI systems can automate tasks, analyze data, and make decisions, they still rely on human input for training, fine-tuning, and oversight. AI systems lack the creativity, emotions, and ethical considerations that humans possess. The goal of AI is to assist humans in solving problems, enhancing productivity, and improving decision-making processes.
FAQ 6: Are all Artificial Neural Networks deep learning models?
No, not all ANNs are deep learning models. Deep learning is a subset of machine learning that utilizes deep neural networks with multiple hidden layers to learn and extract patterns from data. While deep learning has revolutionized AI applications in areas like computer vision, speech recognition, and natural language processing, ANNs can be shallow with just one or a few hidden layers.
FAQ 7: Can Artificial Neural Networks be used for time series forecasting?
Yes, Artificial Neural Networks can be effectively used for time series forecasting. By feeding historical data into an ANN, the network can learn patterns, trends, and associations in the data, enabling it to predict future values. Time series forecasting using ANNs has been successfully applied in various domains, including finance, weather prediction, stock market analysis, and demand forecasting.
FAQ 8: How are Artificial Neural Networks trained?
Artificial Neural Networks are trained using various algorithms, the most common of which is the backpropagation algorithm. In backpropagation, the network iteratively adjusts the weights based on the difference between the predicted output and the desired output. This process involves calculating the error gradient and propagating it backward through the network, updating the weights to minimize the error. Other techniques like gradient descent, stochastic gradient descent, and evolutionary algorithms can also be used for training.
FAQ 9: Are there any risks associated with AI and Artificial Neural Networks?
AI and Artificial Neural Networks come with potential risks and ethical implications. Biases present in training data can be reflected in AI systems, leading to discriminatory outcomes. The use of AI in autonomous systems raises concerns regarding safety, accountability, and decision-making transparency. Additionally, the possibility of AI being misused or manipulated for malicious purposes, such as deepfake technology or algorithmic discrimination, requires careful regulation and ethical considerations.
FAQ 10: What is the future of AI and Artificial Neural Networks?
The future of AI and Artificial Neural Networks looks promising. Continued advancements in hardware, algorithms, and data availability will further enhance the capabilities of AI systems. Deep learning techniques will likely continue to evolve, enabling higher accuracy and performance in various applications. Additionally, there will be an increased focus on ethical AI, explainability, and responsible deployment to ensure the positive impact of AI on society.