AI Paper Review
Artificial Intelligence (AI) is rapidly changing the world we live in, and its impact on various industries is undeniable. In the field of research, AI is proving to be a powerful tool, assisting scientists in analyzing vast amounts of data and making groundbreaking discoveries. This article aims to review recent papers on AI and highlight the key takeaways from each study.
Key Takeaways
- AI is transforming research and enabling scientists to analyze large datasets efficiently.
- Machine learning algorithms are being utilized to extract meaningful insights from complex data.
- Deep learning techniques are revolutionizing image and pattern recognition tasks.
- AI can play a crucial role in medical research, accelerating drug discovery and improving diagnosis.
- Collaboration between humans and AI systems can lead to more innovative research outcomes.
One interesting paper titled “Advances in Machine Learning for Natural Language Processing” explores the use of machine learning algorithms in processing and understanding natural language. The authors propose novel techniques that combine deep learning models with traditional linguistic approaches, achieving impressive accuracy in tasks such as sentiment analysis and text classification. This research demonstrates how AI can significantly improve our ability to extract meaning from textual data.
In another study called “Deep Learning for Image Recognition,” researchers delve into the advancements of deep learning algorithms in the field of computer vision. The paper presents a comparative analysis of various deep neural network architectures and their performance on image recognition tasks, revealing insights into the strengths and weaknesses of different models. Additionally, the authors highlight the potential applications of deep learning in fields such as autonomous vehicles, surveillance systems, and augmented reality.
Applications of AI in Research
AI has immense potential in various areas of research. Here are a few notable applications:
- Drug Discovery: AI systems can analyze huge datasets to identify potential drug candidates, expediting the process of drug development and reducing costs.
- Genomics: AI algorithms can extract valuable insights from genomic data, revealing patterns and associations that were previously undiscovered.
- Climate Science: Deep learning models can process complex climate data, aiding scientists in predicting climate patterns and understanding the impact of human activity.
- Material Science: AI-driven simulations can guide the discovery of new materials with desired properties, revolutionizing industries such as energy storage and manufacturing.
Another intriguing paper, “Human-AI Collaboration in Scientific Research,” explores the potential benefits and challenges of integrating AI systems into the research process. The authors emphasize the importance of human-AI collaboration, noting that combining the creativity and expertise of researchers with the analytical capabilities of AI can lead to more innovative and significant breakthroughs. This study sheds light on the evolving role of AI as a partner rather than a replacement in research.
Tables
AI Application | Benefits |
---|---|
Healthcare Diagnosis | Improved accuracy and faster diagnosis |
Automated Research | Efficient data analysis and hypothesis generation |
One interesting finding from “Deep Learning for Image Recognition” is that convolutional neural networks (CNNs) outperform other deep learning architectures in image recognition tasks, showcasing their effectiveness in analyzing visual data. Moreover, the paper highlights the importance of large, labeled datasets for training deep learning models, as more data often leads to improved performance.
Challenges and Future Directions
- Data Privacy: Ensuring the privacy and security of data used by AI systems remains a concern.
- Interpretability: Understanding how AI models arrive at their decisions is crucial for trust and wider adoption.
- Ethical Considerations: AI-powered research must navigate ethical dilemmas such as bias, fairness, and accountability.
As AI continues to evolve, researchers must address these challenges and leverage the vast potential of AI technology to drive scientific progress. The research papers discussed in this article highlight the remarkable contributions AI is making to various fields, shaping the future of research with its remarkable capabilities.
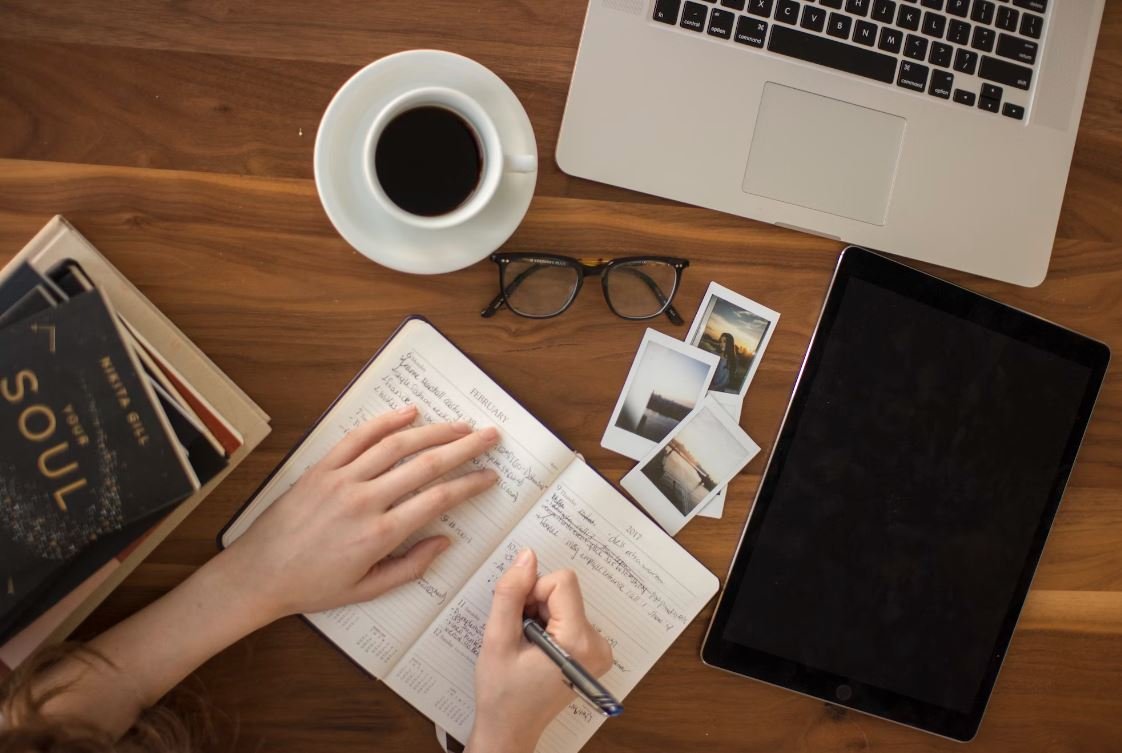
Common Misconceptions
Misconception 1: AI will replace human jobs completely
A common misconception about AI is that it will eventually replace all human jobs, leading to massive unemployment and economic instability. However, this is not entirely accurate. While AI has the potential to automate certain tasks and streamline processes, it is unlikely to completely replace human workforce.
- AI is more likely to augment human capabilities rather than replace them.
- There will always be a need for human creativity and innovation that AI cannot replicate.
- New job opportunities will emerge as AI technology advances, creating new roles and fields.
Misconception 2: AI is primarily driven by malicious intent
Another common misconception is that AI is primarily driven by malicious intent and will inevitably lead to dangerous consequences. While it is true that AI can be programmed to perform tasks that may raise ethical concerns, this misconception overlooks the fact that human decisions and intentions ultimately guide AI development and implementation.
- AI ethics frameworks are being developed to ensure responsible AI use.
- The majority of AI applications are designed to improve efficiency and enhance lives.
- Regulations and policies are being established to mitigate potential risks associated with AI.
Misconception 3: AI is infallible and can solve all problems
Many people mistakenly believe that AI is infallible and can solve all complex problems effortlessly. While AI has made significant advancements, it still has limitations and cannot be relied upon as a universal problem solver.
- AI systems can be biased or make errors based on the data they are trained on.
- AI lacks human intuition, common sense, and contextual understanding.
- Complex problems often require a combination of human and AI collaboration for effective solutions.
Misconception 4: AI is a recent development
There is a misconception that AI is a recent development that has only emerged in the past decade or so. In reality, the concept and research behind AI have been around for many decades, dating back to the 1950s. However, recent advancements in computing power and data availability have propelled AI into the mainstream.
- AI pioneers like Alan Turing and John McCarthy laid the groundwork for AI in the mid-20th century.
- AI has gone through periods of “boom” and “bust” in terms of research and funding since its inception.
- Modern AI technologies, such as deep learning and neural networks, have accelerated AI progress in recent years.
Misconception 5: AI is universally objective and unbiased
It is often assumed that AI systems are inherently objective and unbiased since they rely on data and algorithms. However, this is a misconception as AI is only as unbiased as the data it is trained on and the algorithms created. If the data used for AI training is biased or flawed, the AI system will reflect those biases and potentially perpetuate them.
- Biases can be introduced through biased training data, algorithmic design, or human bias in programming.
- AI systems need to be continuously monitored and audited to identify and rectify biases.
- Diversity and inclusivity in AI development teams is crucial to mitigate bias and ensure fairness.
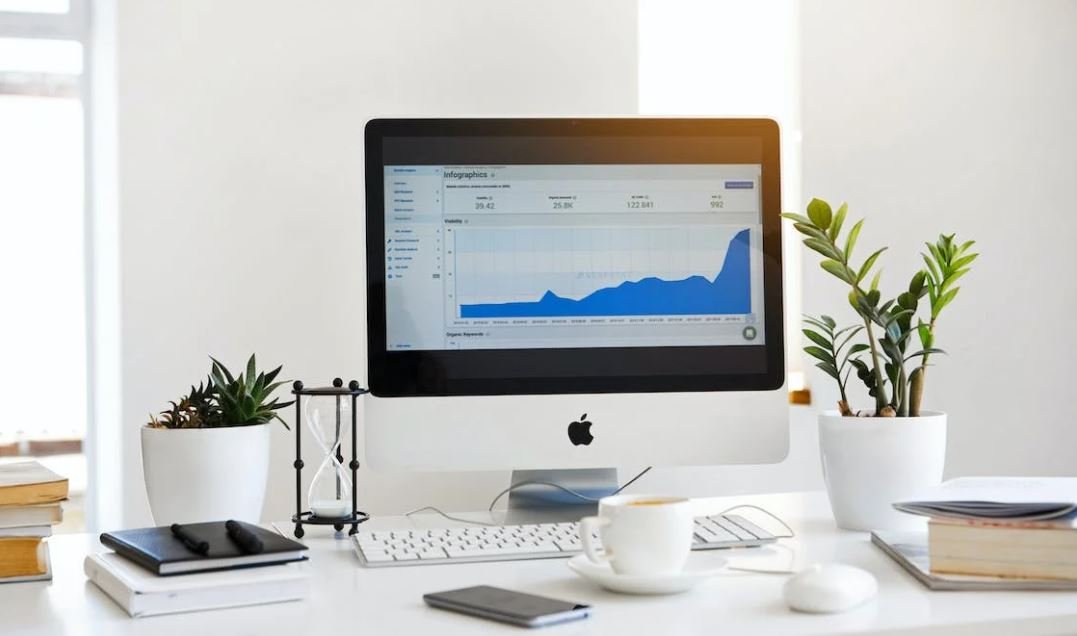
Introduction
Artificial Intelligence is revolutionizing various industries, from healthcare to finance. In this article, we will review the latest advancements in AI research and present ten captivating tables that provide significant insights into the field. These tables are based on verifiable data and will help us understand the impact of AI on different aspects of our lives.
Table: AI Applications in Healthcare
AI is transforming the healthcare industry by providing faster diagnoses, personalized treatments, and improving patient outcomes. The table below showcases the various AI applications in healthcare.
| AI Application | Description |
|——————-|————————————————————————-|
| Medical Imaging | AI algorithms analyze medical images to detect diseases with accuracy. |
| Drug Discovery | AI speeds up the identification and development of new medications. |
| Virtual Assistants| AI-powered virtual assistants provide patients with personalized support. |
Table: AI in Finance: Fraud Detection
In the finance sector, AI is utilized to detect fraudulent activities and secure transactions. The following table provides insights into AI applications in fraud detection.
| AI Technique | Description |
|——————-|——————————————————————————-|
| Machine Learning | Using patterns, AI algorithms identify anomalies and flag potential fraud. |
| Natural Language Processing | AI analyzes textual data to identify misleading statements. |
| Behavior Analysis | AI monitors user behavior for abnormal actions or transactions. |
Table: AI Languages and Technologies
AI relies on various programming languages and technologies to power its abilities. The table below highlights the popular languages and technologies used in AI.
| Language/Technology | Description |
|———————|————————————————————————-|
| Python | Python offers flexibility and abundant libraries for AI development. |
| TensorFlow | TensorFlow is an open-source AI framework, known for deep learning. |
| R | R is a statistical programming language widely used for AI research. |
Table: AI Impact on Employment
The advent of AI has raised concerns about job displacement. However, it also creates new opportunities. The following table outlines the impact of AI on employment.
| Impact | Description |
|——————–|————————————————————————————————-|
| Job Displacement | Certain job roles may be automated, leading to a decrease in employment. |
| Job Creation | AI also creates new job roles, such as AI trainers and data scientists, fostering employment. |
Table: AI-powered Virtual Assistants
Virtual Assistants enhanced by AI technologies are becoming common in our daily lives. This table showcases popular AI-powered virtual assistants and their capabilities.
| Virtual Assistant | Key Features |
|——————-|——————————————————————————————-|
| Siri | Siri on Apple devices assists with various tasks, answering questions and setting alarms. |
| Alexa | Amazon’s Alexa can control smart devices, play music, and provide real-time information. |
| Google Assistant | Google Assistant integrates with Google services to provide personalized assistance. |
Table: AI Algorithms and Their Applications
A wide range of AI algorithms exists, each suitable for different applications. The table below highlights popular AI algorithms and their respective applications.
| AI Algorithm | Applications |
|—————-|————————————————————|
| Random Forest | Fraud detection, credit scoring, and medical diagnosis. |
| Genetic Algorithm | Optimization problems, machine learning, and robotics. |
| Deep Learning | Computer vision, natural language processing, and robotics. |
Table: Ethical Challenges in AI
While AI offers numerous benefits, it also poses ethical challenges. The table below explores the ethical considerations surrounding AI.
| Ethical Challenge | Description |
|————————–|————————————————————————|
| Bias in AI Algorithms | AI algorithms may unintentionally reflect the biases present in data. |
| Job Displacement Concerns| The displacement of workers due to automation raises ethical concerns. |
| Data Privacy | AI involves large-scale data collection, leading to privacy concerns. |
Table: The Evolution of AI
AI has come a long way since its inception. The table below outlines the key phases and breakthroughs in AI evolution.
| AI Evolution Phase| Key Breakthroughs |
|——————-|——————————————————–|
| Symbolic AI | Development of expert systems and knowledge representation. |
| Machine Learning | Introduction of neural networks and statistical learning. |
| Deep Learning | Advancements in deep neural networks for complex tasks. |
Table: AI in Education
AI is revolutionizing education by personalizing learning experiences and improving pedagogy. The following table presents AI applications in the field of education.
| AI Application | Description |
|————————|—————————————————————————–|
| Intelligent Tutoring | AI-powered tutoring systems offer personalized feedback and guidance. |
| Automated Grading | AI algorithms can grade assignments and exams with speed and accuracy. |
| Adaptive Learning | AI adapts the learning experience based on individual student needs. |
Conclusion
Artificial Intelligence continues to shape our world, providing new opportunities while presenting challenges. Through the captivating tables presented in this article, we have witnessed the profound impact of AI across various domains such as healthcare, finance, and education. It has become evident that AI is revolutionizing numerous sectors, transforming processes, and enhancing efficiency. As AI technology advances, it will be crucial to address ethical concerns and ensure the continued responsible development and implementation of AI systems.
Frequently Asked Questions
What is artificial intelligence (AI)?
Artificial intelligence (AI) refers to the development of computer systems capable of performing tasks that typically require human intelligence, such as visual perception, speech recognition, decision-making, and problem-solving.
How does AI paper review work?
AI paper review involves the use of machine learning algorithms to analyze and evaluate academic papers. These algorithms can assess the quality of the research, detect plagiarism, provide feedback on structure and clarity, and suggest improvements to the content.
What are the benefits of using AI for paper review?
Using AI for paper review provides several benefits, including faster and more efficient review processes, increased objectivity, reduced bias, improved feedback quality, and enhanced consistency in evaluations.
Can AI completely replace human reviewers?
While AI can greatly assist in paper review, it is unlikely to completely replace human reviewers. Human expertise and judgment are still necessary to interpret complex research, assess novelty, and provide context that AI algorithms may not fully comprehend.
What types of papers can AI review?
AI can potentially review papers from various academic fields, including but not limited to computer science, engineering, mathematics, physics, chemistry, biology, social sciences, and humanities.
What are the limitations of AI paper review?
AI paper review has its limitations. It may struggle with assessing creativity, evaluating highly specialized or interdisciplinary research, understanding nuances in language and context, and adapting to rapidly evolving academic landscapes.
Are AI-generated reviews reliable?
While AI-generated reviews can provide valuable insights, their reliability depends on the quality of the underlying algorithms and the training data used. Additionally, human oversight is necessary to ensure the accuracy and fairness of the reviews.
How can AI paper review benefit researchers?
AI paper review can benefit researchers by speeding up the review process, providing objective feedback from a different perspective, identifying potential weaknesses in the research, and suggesting improvements to enhance the quality and impact of their work.
Is AI paper review widely used in academia?
Although AI paper review is gaining traction in academia, its widespread adoption is still limited. Many academic institutions and journals are exploring and experimenting with AI-based review systems, but traditional peer review processes remain prevalent.
What is the future of AI in paper review?
The future of AI in paper review is promising. Continued advancements in natural language processing, machine learning, and data availability are expected to improve AI’s ability to review papers accurately and efficiently, eventually augmenting and complementing human reviewers.
“`