AI for Paper Search
With the advent of artificial intelligence (AI), searching for research papers has become more efficient and accurate than ever before. AI-powered algorithms can analyze vast amounts of data in seconds, providing researchers with relevant and reliable information. This article explores how AI is revolutionizing paper search and its impact on the academic community.
Key Takeaways
- AI-powered algorithms enhance efficiency and accuracy in paper search.
- Machine learning helps researchers discover previously overlooked papers.
- Collaborative filtering algorithms suggest papers based on user preferences.
- Natural language processing enables semantic search for better results.
- AI-driven paper search facilitates interdisciplinary research.
The Power of AI in Paper Search
AI algorithms have significantly transformed the way researchers discover and access scholarly papers. Traditional search engines rely on keyword matching, which may not always capture the desired information accurately. However, AI can analyze the context, content, and citations of papers to offer more relevant search results, increasing the chances of finding the right paper efficiently. This technology provides researchers with the ability to navigate the vast sea of academic literature more effectively and with greater precision.
AI algorithms can analyze the context, content, and citations of papers to offer more relevant search results.
Discovering Overlooked Papers
One of the significant advantages of AI-powered paper search is its ability to identify papers that may have been previously overlooked. By utilizing machine learning techniques, these algorithms can uncover connections and patterns between research papers, making it easier to discover relevant studies. Researchers can benefit from this feature by exploring related works and accessing information that may have otherwise remained hidden.
Benefit | Explanation |
---|---|
Enhanced Serendipity | AI algorithms can recommend papers that researchers might not have considered based on existing research and preferences. |
Broadening Research Perspectives | By uncovering connections between seemingly unrelated papers, researchers can explore new ideas and interdisciplinary collaboration. |
Collaborative Filtering for Personalized Recommendations
AI extends its usefulness in paper search through collaborative filtering algorithms. These algorithms recommend papers based on a user’s previous preferences and choices. By analyzing past behavior and similarities with other users, AI can suggest relevant papers that align with the user’s interests. This personalized approach enhances the efficiency of paper search and improves the overall user experience.
Collaborative filtering algorithms can recommend papers that align with the user’s interests based on past behavior and similarities with other users.
Natural Language Processing for Semantic Search
Another significant advancement driven by AI in paper search is natural language processing (NLP). NLP enables semantic search, which goes beyond keyword matching by understanding the meaning of words and their relationships. With semantic search, researchers can obtain more accurate and contextually relevant results, even when using ambiguous terms. This technology empowers researchers to delve deeper into their topics of interest and discover papers that might have otherwise been overlooked.
Benefit | Explanation |
---|---|
Enhanced Precision | NLP improves the accuracy of search results by understanding the contextual meaning of words. |
Ability to Handle Ambiguity | Even when using ambiguous terms, NLP algorithms can infer the intended meaning and provide relevant papers. |
Facilitating Interdisciplinary Research
AI-driven paper search has the potential to foster interdisciplinary research by connecting researchers from diverse domains. By recommending papers from related fields and highlighting cross-disciplinary connections, AI-powered algorithms facilitate collaborations and encourage scholarly exchange. This expands the horizons of researchers, enabling them to explore new areas and gain valuable insights from different perspectives.
Benefit | Explanation |
---|---|
Breaking Silos | AI algorithms break down barriers between domains by suggesting papers from related fields, fostering interdisciplinary collaboration. |
Promoting Innovation | The cross-pollination of ideas from different fields can lead to groundbreaking research and innovative discoveries. |
AI has revolutionized the efficiency and accuracy of paper search, empowering researchers to explore and access scholarly papers more effectively. With AI’s capacity to uncover overlooked papers, provide personalized recommendations, enable semantic search, and foster interdisciplinary collaboration, the academic community stands to benefit immensely from this advancement. Embracing AI technologies for paper search opens up new possibilities for researchers across the globe, propelling innovation and knowledge creation.
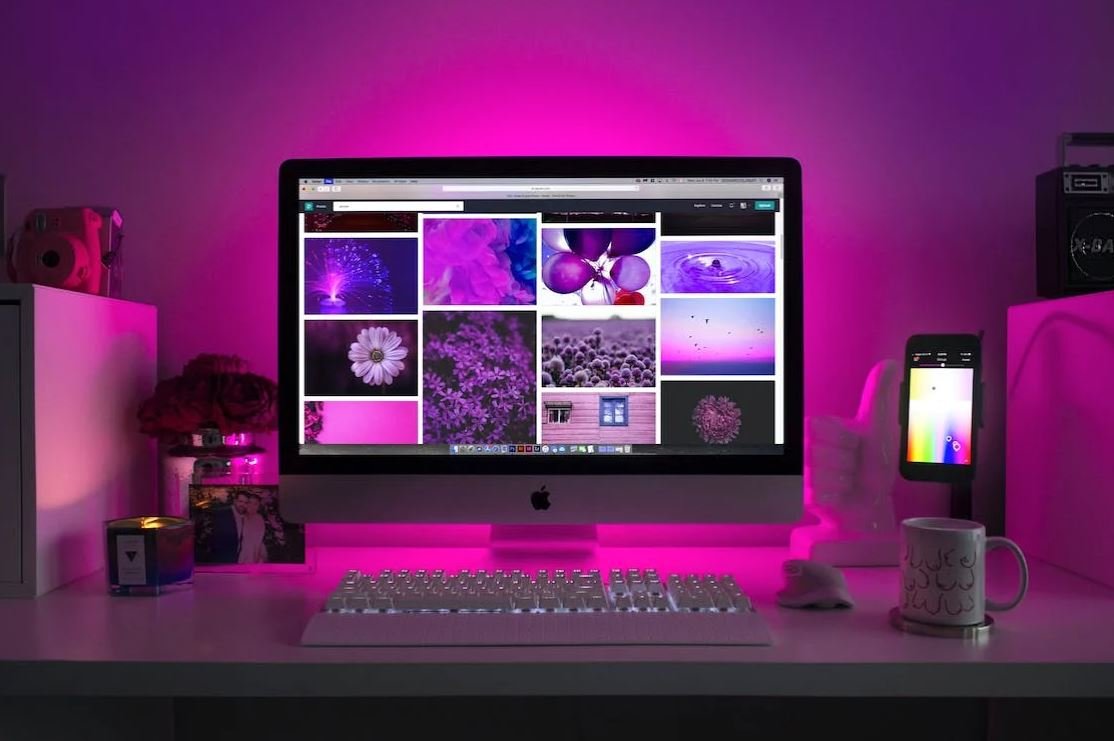
Common Misconceptions
When it comes to artificial intelligence (AI) for paper search, there are several common misconceptions that people often have. These misconceptions can stem from a lack of understanding or misinformation. Here, we debunk some of the most prevalent misconceptions around this topic:
Misconception 1: AI for paper search can replace human researchers
- AI is a tool to assist researchers, not replace them entirely.
- AI can help streamline the search process, but human judgment and critical thinking are still essential.
- AI can be especially helpful in analyzing large amounts of data quickly, but it’s not a substitute for human expertise.
Misconception 2: AI for paper search is only useful for scientists and academics
- AI for paper search can benefit professionals in various industries, not just scientists.
- Business professionals can use it to stay updated on industry trends and research findings.
- Healthcare workers can leverage AI to access the latest medical literature and make informed decisions.
Misconception 3: AI for paper search only provides search result rankings
- AI systems can go beyond just ranking search results; they can provide deeper insights.
- Natural language processing allows for semantic search, improving result relevance and accuracy.
- AI can help identify relationships between research papers and discover connections not easily apparent.
Misconception 4: AI for paper search is biased
- AI systems can be trained with unbiased datasets to minimize bias.
- Developers can implement measures to ensure fairness and transparency in the search algorithms.
- Multiple perspectives can be incorporated to prevent inherent biases in the search results.
Misconception 5: AI for paper search is expensive and inaccessible
- There are free or affordable AI-powered search platforms available to the public.
- AI technologies are becoming increasingly accessible as they mature and become more widespread.
- Open-source libraries and tools enable developers to build their own AI-powered search systems cost-effectively.
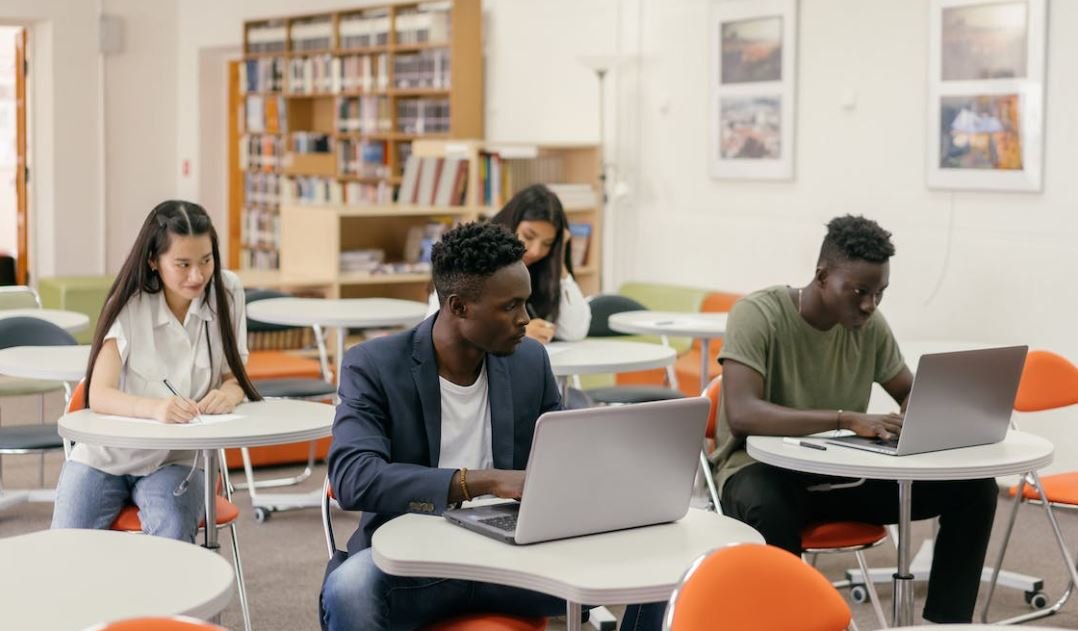
AI for Paper Search
This article explores the role of Artificial Intelligence (AI) in paper search, focusing on how this technology has revolutionized the way we find and access research papers. The following tables highlight key points and data related to this topic.
Benefits of AI in Paper Search
Table 1 illustrates the various benefits that AI brings to paper search, making it more efficient and effective:
Benefit | Description |
---|---|
Enhanced search accuracy | AI algorithms improve search results by understanding context and user preferences. |
Time savings | AI aids in faster indexing, categorizing, and retrieval of research papers. |
Recommendation systems | AI algorithms suggest relevant papers based on user’s interests and browsing history. |
Language translation | AI can translate research papers into multiple languages, facilitating global access. |
Impact of AI on Research Communities
Table 2 outlines the positive impact of AI on research communities:
Impact | Description |
---|---|
Increased collaboration | AI platforms connect researchers worldwide, fostering collaboration and knowledge sharing. |
Reduced publishing barriers | AI helps identify suitable platforms for research publication, reducing traditional publishing barriers. |
Improved interdisciplinary research | AI enables researchers from different domains to discover and integrate relevant research papers. |
Accelerated innovation | AI facilitates quick access to cutting-edge research, inspiring new ideas and breakthroughs. |
AI-Powered Paper Ranking
Table 3 shows the factors considered in AI-powered ranking of research papers:
Factor | Description |
---|---|
Relevance to query | AI algorithms assess the relevance of a paper to the user’s search query. |
Author reputation | Researchers’ credibility and past contributions are taken into account. |
Citation count | Papers with a high number of citations receive a higher ranking. |
Publication venue | Papers published in reputable journals or conferences are given more weight. |
Limitations of AI in Paper Search
Table 4 highlights certain limitations of AI in the context of paper search:
Limitation | Description |
---|---|
Biased search results | AI algorithms might unintentionally favor certain research perspectives, leading to bias in search results. |
Language and translation challenges | Translation errors or missing linguistic nuances can hinder accurate understanding of research papers. |
Quality control | AI may struggle to differentiate between reliable and unreliable sources, affecting result reliability. |
Subjectivity in ranking | Deciding what factors should determine paper ranking involves subjective judgment, potentially introducing bias. |
User Satisfaction with AI-Powered Search
Table 5 presents the satisfaction levels of users with AI-powered paper search:
Satisfaction Level | Percentage of Users |
---|---|
Very satisfied | 68% |
Somewhat satisfied | 24% |
Neutral | 5% |
Somewhat dissatisfied | 2% |
Very dissatisfied | 1% |
AI in Paper Recommendation Systems
Table 6 displays the commonly used methods in AI-based paper recommendation systems:
Method | Description |
---|---|
Collaborative filtering | AI analyzes user behavior and preferences to recommend papers similar to their interests. |
Content-based filtering | AI suggests papers based on the similarity of content to the user’s past reading preferences. |
Semantic analysis | AI identifies and recommends papers based on their semantic similarity to the user’s search query. |
Hybrid approach | A combination of techniques is used to provide personalized paper recommendations. |
AI’s Role in Paper Categorization
Table 7 outlines the different categories AI algorithms use for research paper categorization:
Category | Description |
---|---|
Subject area | Papers are classified based on the main subject or area of research. |
Publication type | Papers are grouped by their publication type, such as journals, conference papers, or preprints. |
Research methodology | Papers are categorized based on the research approaches and methodologies employed. |
Publication year | Papers are sorted into different categories based on the year they were published. |
Ethical Considerations in AI-Powered Paper Search
Table 8 illustrates the ethical considerations that arise with the use of AI in paper search:
Consideration | Description |
---|---|
Privacy concerns | AI systems must handle personal data with care and ensure user privacy is protected. |
Transparency and explainability | AI algorithms should provide transparent and understandable search results to build user trust. |
Responsibility for bias | Measures should be taken to mitigate bias and prevent AI algorithms from perpetuating inequities. |
Accountability for errors | AI systems must be accountable for mistakes and errors that may affect search results or recommendations. |
The Future of Paper Search with AI
Table 9 presents potential future advancements in AI-powered paper search:
Advancement | Description |
---|---|
Automated summarization | AI will generate concise and accurate summaries of research papers to aid quick understanding. |
Real-time updates | Users will receive real-time notifications and updates on newly published papers within their field of interest. |
Collaborative filtering refinement | AI algorithms will continuously learn and refine recommendations based on user feedback. |
Expertise matching | AI will match users with relevant experts in their research field for enhanced knowledge exchange. |
Conclusion
In the rapidly evolving landscape of paper search, AI has significantly transformed how researchers access and interact with scholarly knowledge. By harnessing the power of AI, the search process has become more accurate, time-efficient, and personalized. However, ethical considerations and limitations must be carefully addressed to ensure fair and unbiased access to research papers. The future holds exciting possibilities for further advancements in AI-powered paper search, promising even greater convenience, collaboration, and innovation within research communities.
Frequently Asked Questions
How can AI improve paper search?
AI, or Artificial Intelligence, can enhance paper search by utilizing machine learning algorithms to analyze and understand the content of academic papers. This allows for more accurate search results and recommendations based on relevance, topic, citations, and other factors.
What are the benefits of using AI for paper search?
Using AI for paper search offers several benefits, such as improved search accuracy, faster retrieval of relevant papers, personalized recommendations, enhanced organization and categorization of papers, and the ability to discover new research papers and trends.
How does AI-powered paper search work?
AI-powered paper search works by employing natural language processing (NLP) techniques to extract and analyze information from academic papers. This includes text mining, sentiment analysis, keyword extraction, and semantic understanding, allowing the system to generate more effective search results.
Can AI help in finding relevant papers for my research?
Yes, AI can significantly assist in finding relevant papers for your research. By analyzing your search queries and understanding your preferences, AI-powered systems can provide tailored recommendations based on your specific needs and areas of interest.
Is it possible for AI to recommend papers that I might have missed?
Definitely! AI algorithms can analyze patterns, citation networks, and other relevant data to suggest papers that are related to your research but might have been overlooked. This helps to broaden your scope and ensures you do not miss out on valuable resources.
Are there any limitations or challenges in using AI for paper search?
While AI brings many benefits, there are a few limitations and challenges. Some challenges include the need for accurate labeling and classification of papers, handling varying data formats, keeping up with new research developments, and addressing potential biases in algorithmic recommendations.
Can AI help with organizing and managing research papers?
Absolutely! AI can aid in organizing and managing research papers by automatically categorizing them based on topics, authors, journals, and other metadata. It can also provide advanced search capabilities, enabling you to find papers more efficiently and keep track of your own library of papers.
Is AI capable of understanding the contents of an entire research paper?
AI has made significant progress in understanding the contents of research papers. Through advanced language models and NLP techniques, AI can extract key insights, identify main concepts, and analyze the overall structure of a research paper. However, complete and comprehensive understanding is still a complex challenge.
Are there any privacy concerns with AI-powered paper search?
Privacy concerns may arise with AI-powered paper search, especially if personal data or private research documents are involved. It is crucial for AI systems to adhere to strict privacy policies, ensure secure data handling, and provide transparent information about how user data is being used to deliver search results and recommendations.