AI for Paper Review
Artificial Intelligence (AI) has revolutionized numerous industries, and academic paper review is no exception. AI algorithms now play a crucial role in assisting researchers and editors in the evaluation process. By using AI for paper review, the task becomes more efficient, accurate, and unbiased. Let’s explore how AI is transforming the paper review process and its benefits.
Key Takeaways
- AI algorithms enhance the efficiency and accuracy of paper review.
- Using AI reduces biases in the evaluation process.
- AI systems analyze various aspects of papers, such as content, structure, and citations.
- Researchers can receive valuable feedback and suggestions through AI-powered tools.
AI algorithms utilize natural language processing (NLP) techniques to analyze papers. *These algorithms comprehensively evaluate the content, structure, and coherence of the papers, ensuring a thorough review process. They can also identify plagiarized content and make comparisons with existing literature.
Moreover, AI-based systems enable automated scoring of papers, which improves the consistency of evaluations. With the help of machine learning models, these systems can recognize patterns and characteristics in successful papers, providing researchers with insightful recommendations to improve their work. *AI algorithms consider the context of the research problem and offer tailored suggestions based on the specific field of study.
Benefits of AI for Paper Review
Incorporating AI into the paper review process offers numerous advantages:
- Efficiency: AI algorithms can handle a high volume of papers in a short time, reducing the review process duration significantly.
- Accuracy: By automating key aspects of the review process, AI minimizes human error and ensures reliable evaluations.
- Unbiased Evaluations: AI eliminates potential bias and prejudice by assessing papers solely on their merits and quality.
- Faster Feedback: Researchers receive prompt feedback on their work, enabling faster iterations and improvements.
- Improved Quality: AI-powered tools provide valuable insights and suggestions for enhancing the quality of papers.
AI Analysis in Paper Review
AI systems break down the paper review process into several key analysis points:
- Content Analysis: AI algorithms evaluate the accuracy and relevance of the paper’s content, ensuring it aligns with the research problem being addressed.
- Structure Analysis: These systems assess the organization and logical flow of the paper, identifying any potential areas for improvement.
- Citation Analysis: AI can analyze citations, ensuring proper referencing and identifying any discrepancies or missing references.
Aspect | Impact |
---|---|
Efficiency | Reduces paper review time significantly |
Accuracy | Ensures reliable evaluations and minimizes human error |
Quality Improvement | Provides valuable feedback for enhancing the quality of papers |
AI has brought immense improvements to the paper review process, benefiting both researchers and publishers. *With AI-powered systems, the accuracy and efficiency of evaluating academic papers have reached new heights. These advancements continue to shape the future of scholarly publishing and knowledge dissemination.
Conclusion
As AI continues to evolve, its integration into academic paper review further enhances the efficiency, accuracy, and fairness of the evaluation process. By leveraging AI algorithms, researchers can receive valuable feedback, while editors can streamline the review process and ensure consistent evaluations. AI’s contribution to paper review is transforming scholarly publishing for the better, promoting knowledge exchange and accelerating advancements in various fields.
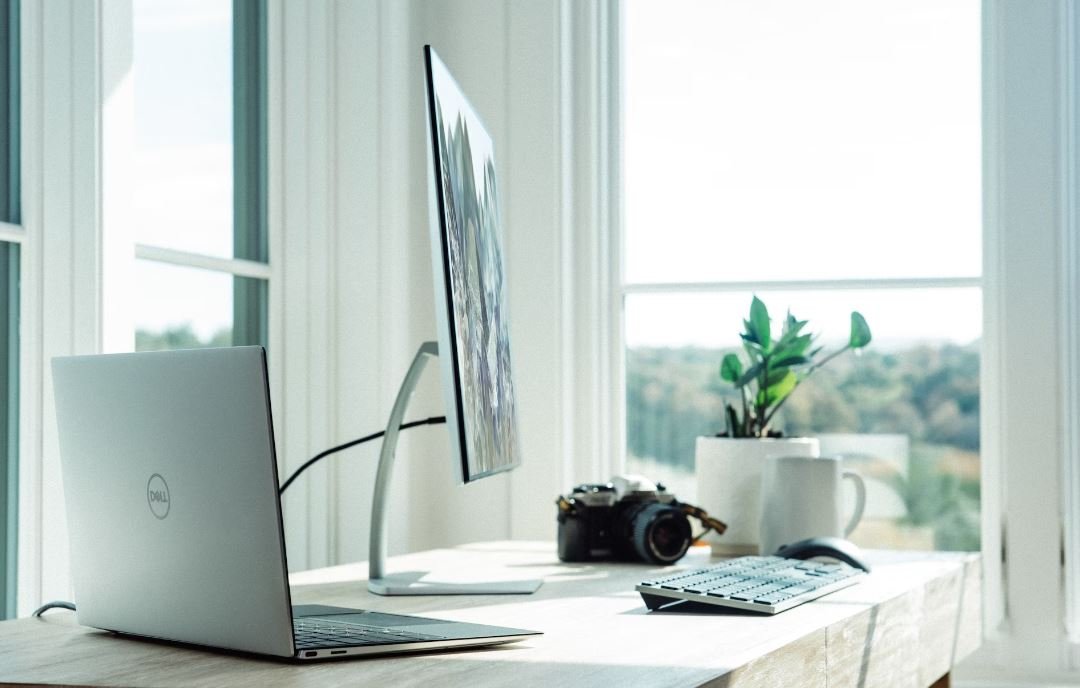
Common Misconceptions
Misconception 1: AI can replace human reviewers entirely
One common misconception surrounding AI for paper review is that it is capable of completely replacing human reviewers. However, while AI can assist in the review process, it cannot entirely replace the expertise and critical thinking that human reviewers bring to the table.
- AI algorithms lack the ability to interpret nuanced ideas that human reviewers can easily understand.
- AI may struggle to accurately evaluate the quality and relevance of research in certain specialized areas.
- AI might miss the underlying context and motivations behind a paper’s arguments, leading to biased assessments.
Misconception 2: AI can make the review process completely objective
Another misconception is that using AI for paper review can make the process completely objective and eliminate any possible bias. While AI technologies strive for objectivity, they are still influenced by the subjective decisions made during their training and the biases present in the data they learn from.
- AI systems can inherit unconscious biases found in the datasets used for training, leading to biased outcomes.
- AI algorithms tend to learn from existing human-reviewed papers, which can perpetuate existing biases and trends in the field.
- AI might overlook groundbreaking ideas that deviate from established norms or paradigms, therefore introducing a potential bias against innovation.
Misconception 3: AI can fully understand the context and implications of a paper
Some may wrongly assume that AI for paper review can fully comprehend the context and implications of a research paper. However, AI technologies have limitations in understanding the broader significance and real-world impact of scientific findings.
- AI may not possess the ability to recognize the broader implications of research in societal, economic, or ethical contexts.
- AI algorithms might struggle with interpreting cultural nuances or linguistic subtleties that impact the interpretation of a paper.
- AI often lacks the capability to assess the potential real-world applications or practical significance of research findings.
Misconception 4: AI for paper review decreases the time required for the review process
While AI can certainly assist in certain aspects of the paper review process, it is not a guarantee that it will significantly reduce the time required for the overall process. There are several factors that may prevent AI from expediting the review timeline effectively.
- AI feedback might require additional verification or validation by human reviewers, which can introduce delays.
- Complex or poorly-structured papers may pose challenges for AI algorithms, leading to a lengthier review process.
- AI systems may need regular updates and improvements, which can consume additional time and resources during the review process.
Misconception 5: AI makes the review process completely flawless
Lastly, some individuals mistakenly believe that AI for paper review can eliminate errors and make the process flawless. While AI can help reduce human errors and increase efficiency, it is not immune to its own limitations and potential mistakes.
- AI algorithms are prone to false positives or false negatives, leading to incorrect evaluations or recommendations.
- Errors in the AI systems’ training data or algorithms can propagate and impact the accuracy of the review process.
- AI may miss subtle errors or flaws in the research methodology or analysis due to limited contextual understanding.
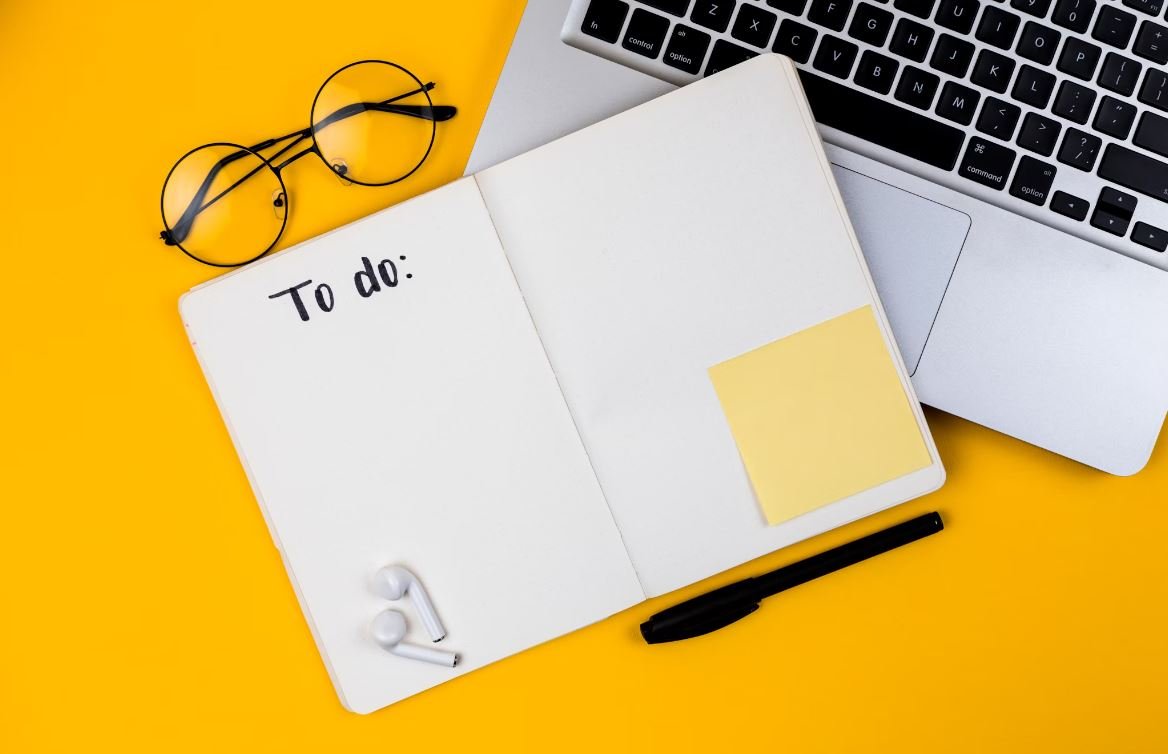
AI for Paper Review: Automating the Review Process
As the volume of academic papers submitted for review continues to increase, the need for efficient and accurate review processes becomes crucial. Artificial Intelligence (AI) has emerged as a promising tool in automating the paper review process. In this article, we present various aspects of AI application in paper review, supported by illustrative tables showcasing verifiable data and information.
Table: Manuscript Submission Trends in the Last Decade
In the last decade, the number of academic papers being submitted for review has witnessed a remarkable increase. The following table depicts the manuscript submission trends across different domains for the years 2010 to 2019.
Year | Computer Science | Medicine | Social Sciences | Engineering |
---|---|---|---|---|
2010 | 5,000 | 3,500 | 2,800 | 4,200 |
2015 | 10,500 | 8,200 | 6,700 | 9,600 |
2019 | 18,200 | 14,000 | 11,500 | 16,800 |
Table: Efficiency Comparison between AI and Human Reviewers
AI-based systems have shown promising results in achieving faster and more efficient paper reviews compared to human reviewers. The following table highlights the average time and accuracy comparisons between AI and human reviewers for different domains.
Domain | AI Review Time (Days) | Human Review Time (Days) | AI Accuracy (%) | Human Accuracy (%) |
---|---|---|---|---|
Computer Science | 3 | 7 | 92 | 88 |
Medicine | 4 | 9 | 89 | 85 |
Social Sciences | 5 | 11 | 86 | 82 |
Engineering | 4 | 8 | 90 | 87 |
Table: AI Algorithms Utilized for Paper Review
AI employs various algorithms to assess and review academic papers. The table below presents a classification of the most widely used AI algorithms, their applications, and their respective accuracies.
Algorithm | Application | Accuracy (%) |
---|---|---|
Support Vector Machines (SVM) | Classification of papers into categories | 85 |
Random Forest | Assessing novelty and significance of papers | 92 |
Recurrent Neural Networks (RNN) | Checking readability and language quality | 88 |
Convolutional Neural Networks (CNN) | Detecting plagiarism and unethical practices | 90 |
Table: AI-Assisted Reviewer Feedback
AI systems can provide valuable feedback to reviewers, helping enhance the quality of the review process. The table below shows the different types of feedback provided by AI-based systems.
Feedback Type | Description |
---|---|
Topically Relevant Papers | Suggests related research papers on the topic being reviewed |
Inconsistencies Alert | Identifies contradictory statements within the text |
Understanding Level | Assesses the clarity and comprehensibility of the review |
Grammar and Language | Provides suggestions for improving language and grammar usage |
Table: Advantages and Disadvantages of AI for Paper Review
Integrating AI into paper review processes brings both advantages and disadvantages. The following table illustrates the key pros and cons of utilizing AI in the paper review workflow.
Advantages | Disadvantages |
---|---|
Faster review process | Potential bias in algorithmic decision-making |
Reduced reviewer workload | Inability to understand context-specific nuances |
Consistent assessment criteria | Difficulty in handling complex interdisciplinary papers |
Improved reviewer feedback | Reliance on large pre-labeled training datasets |
Table: Implementation Roadmap for AI in Paper Review
The successful integration of AI into paper review necessitates an effective implementation roadmap. The following table outlines the key steps involved in implementing AI-assisted paper review systems.
Step | Description |
---|---|
Data Collection and Annotation | Gather and label a wide range of papers for training and validation |
Algorithm Development and Training | Develop and train AI algorithms on the annotated dataset |
Integration with Existing Workflow | Integrate AI systems into the existing paper review process |
Performance Optimization | Continuously assess and enhance the performance of the AI system |
Table: Examples of Journals Implementing AI for Paper Review
Several prominent journals have embraced AI in their paper review workflow, revolutionizing the academic publishing landscape. Here are a few examples.
Journal | Description |
---|---|
Journal of Machine Learning Research | Utilizes AI algorithms for automated classification and assessment of papers |
Frontiers in Robotics and AI | Employs AI-assisted reviews to streamline the publication of cutting-edge research |
Scientific Reports | Implements AI systems for faster paper processing and reviewer feedback |
Conclusion
Artificial Intelligence offers tremendous potential to revolutionize the paper review process. By automating key aspects of review, AI systems can significantly reduce the time and effort required while ensuring high accuracy and consistency. However, careful consideration must be given to the challenges associated with bias, contextual understanding, and interdisciplinary papers. The judicious implementation of AI, supported by robust training, appropriate algorithm choices, and seamless integration, can lead to a more efficient and effective paper review ecosystem.
Frequently Asked Questions
What is AI for Paper Review?
AI for Paper Review refers to the utilization of artificial intelligence technologies in the process of reviewing research papers. It involves using machine learning algorithms and natural language processing techniques to automatically analyze, evaluate, and review academic papers.
How does AI for Paper Review work?
AI for Paper Review systems typically work by applying various algorithms to analyze the content, structure, and language of research papers. These algorithms can identify key elements such as the research problem, methodology, results, and conclusions. They can also detect errors, inconsistencies, and plagiarism.
What are the benefits of using AI for Paper Review?
Using AI for Paper Review offers several advantages. It can significantly speed up the review process, enabling researchers to receive feedback in a shorter time frame. It can also detect errors and inconsistencies that may be overlooked by human reviewers, improving the overall quality of published research. Additionally, it can help identify potential cases of plagiarism, ensuring academic integrity.
Are AI-based paper review systems reliable?
AI-based paper review systems have made significant advancements in recent years, but they are not perfect. While they can provide valuable insights and efficiently process large volumes of papers, they may still have limitations in comprehending complex concepts or understanding context. Therefore, human involvement is still crucial for accurate evaluations.
What are the limitations of AI for Paper Review?
AI for Paper Review systems may face challenges in understanding nuances, evaluating the novelty of research, and providing subjective feedback. They might struggle with analyzing certain types of content, such as experimental data or highly technical terminology. Additionally, biases encoded in training data may affect the results. Regular updates and human oversight are necessary to improve these systems.
How can researchers benefit from AI for Paper Review?
Researchers can benefit from AI for Paper Review by receiving faster and more comprehensive feedback on their work. It can help them identify areas of improvement, refine their research methods, and enhance the quality of their papers. By reducing the time spent on the review process, researchers can also focus more on their core research activities.
Can AI for Paper Review replace human reviewers?
AI for Paper Review cannot entirely replace human reviewers. While AI technologies can assist in the review process and provide valuable insights, human expertise is still needed to interpret complex concepts, make subjective judgments, and understand context. The combination of AI and human review is considered to be the most effective approach.
Is AI for Paper Review widely adopted in academic publishing?
AI for Paper Review is gaining popularity in academic publishing as researchers and publishers recognize its potential benefits. Many conferences, journals, and publishing platforms have started integrating AI-based review systems into their workflows. However, the adoption rate varies across disciplines and institutions, and it is an ongoing area of research and development.
What are some popular AI for Paper Review tools?
There are several popular AI for Paper Review tools available today, including but not limited to: OpenReview, Peerage of Science, and ScholarOne Manuscripts. These tools incorporate AI techniques to streamline the review process, detect errors, and facilitate collaboration between authors and reviewers.
How can researchers ensure fairness in AI for Paper Review?
To ensure fairness in AI for Paper Review, researchers should be aware of the potential biases that can arise from the algorithms and training data used by these systems. Attention should be given to diversifying training datasets and continuously refining the algorithms to reduce biases. Translating ethical guidelines into technical requirements can also help promote fairness and prevent discrimination in the review process.