AI for Paper Analysis
In today’s digital age, artificial intelligence (AI) has made significant advancements in various fields, including the analysis of academic papers. AI has revolutionized the way research is conducted, making it faster, more accurate, and more efficient than ever before. This article aims to explore the use of AI in paper analysis and the benefits it brings to researchers and academics.
Key Takeaways
- AI technology has greatly improved the efficiency and accuracy of paper analysis.
- Automated paper analysis saves researchers valuable time and enables them to focus on higher-level tasks.
- AI tools can assist in identifying key insights and trends in large volumes of research papers.
**Artificial intelligence** has transformed the process of **paper analysis** by providing advanced methods for extracting valuable information from scholarly articles. *By leveraging machine learning algorithms and natural language processing techniques*, AI systems can analyze and interpret vast amounts of text data within seconds.
One of the most significant advantages of utilizing AI for paper analysis is the immense time saved. *Researchers no longer have to spend countless hours manually reading through numerous papers.* With AI tools, the process is automated and significantly expedited, allowing researchers to devote more time to conducting experiments, developing hypotheses, and advancing their own research.
AI systems can effectively **summarize** and **synthesize** the content of research papers, whether they are scientific articles, conference papers, or academic publications. This provides researchers with a **quick overview** of the core ideas and findings presented in a particular paper. *By automatically extracting key points and main arguments*, AI saves researchers from consuming unnecessary information and facilitates decision-making regarding its relevance.
Data Analysis and Visualization
AI technology helps streamline complex tasks such as data analysis and visualization. AI algorithms can quickly identify **trends**, **patterns**, and **correlations** within a large volume of research papers, enabling researchers to spot emerging research topics and novel connections between subjects. By providing visual representations through graphs, charts, and diagrams, AI enables researchers to interpret and present data in a more understandable and attractive manner.
AI Applications in Paper Analysis | Benefits |
---|---|
Text mining and information extraction | – Efficiently analyze large volumes of papers – Recognize key entities, relations, and concepts |
Topic modeling and clustering | – Discover latent topics and trends – Group papers based on thematic similarities |
Automatic citation extraction | – Extract references and citations accurately – Generate citation networks for further investigation |
Within the field of academic research, data integrity and reliability are crucial. AI systems can assist in assessing the **credibility** of research papers by evaluating factors such as the **reputation** of the authors, the **impact factor** of the publishing journal or conference, and the **citation history** of the paper. This helps researchers in identifying the most **authoritative** and **trustworthy** sources of information.
Automated Paper Recommendation
Another significant application of AI in paper analysis is automated recommendation systems. By examining the content and metadata of research papers, AI algorithms can provide personalized recommendations tailored to researchers’ interests and preferences. This eliminates the need for researchers to manually search for relevant papers, saving them time and enabling them to explore a wider range of related topics.
Advantages of Automated Paper Recommendation | Benefits |
---|---|
Expanded research scope | – Access to a diverse range of research |
Saved time and effort | – No need to manually search for papers |
Discoverability of lesser-known papers | – Uncover hidden gems and novel findings |
AI for paper analysis has become an invaluable tool for researchers and academics across various disciplines. By automating tedious tasks, providing comprehensive summaries, enabling efficient data analysis, and offering personalized paper recommendations, AI brings immense benefits to the scholarly community. As AI continues to advance, its capacity to revolutionize paper analysis will only grow, empowering researchers to make remarkable advancements in their respective fields of study.
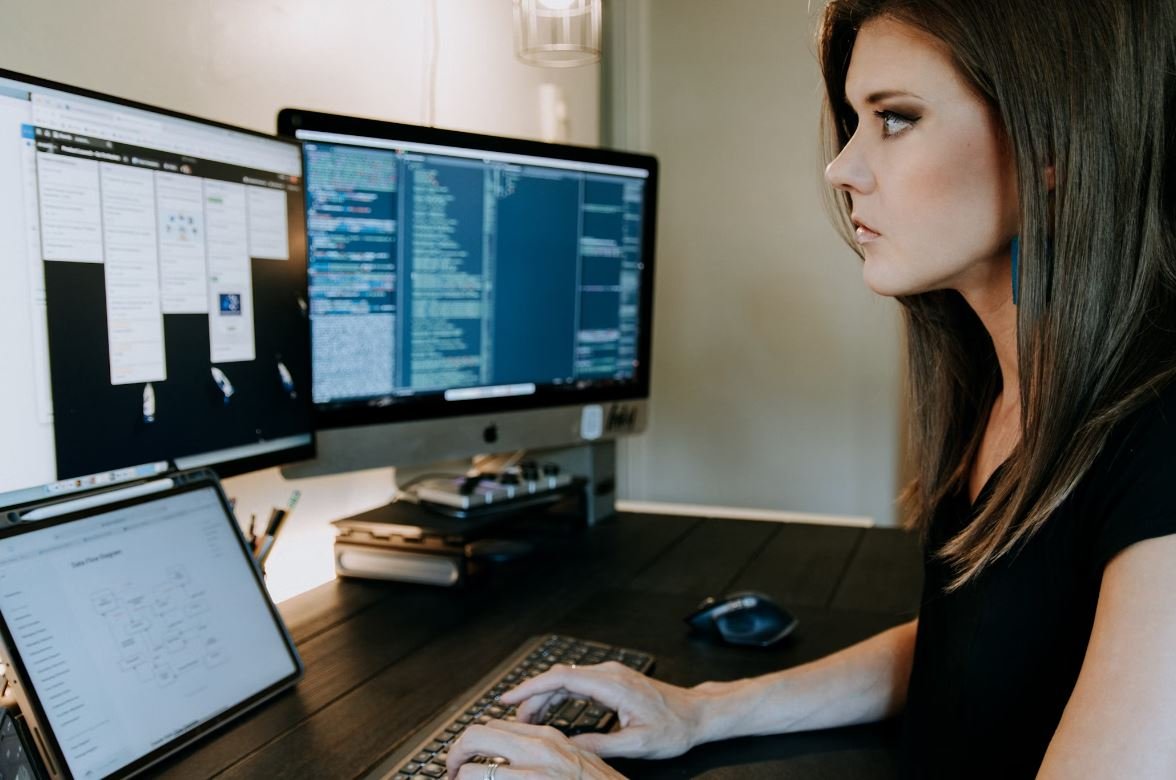
Common Misconceptions
Misconception 1: AI can perfectly analyze papers without human intervention
One common misconception about AI for paper analysis is that it is capable of perfectly analyzing papers without the need for human intervention. While AI has made significant advancements in natural language processing and machine learning algorithms, it is still not foolproof.
- AI algorithms may struggle with certain language nuances and subtleties that humans can easily understand.
- AI may misinterpret ambiguous or context-dependent statements in papers, leading to inaccurate analysis.
- Human involvement is crucial to correct any mistakes or misinterpretations made by AI algorithms.
Misconception 2: AI can replace human researchers and their expertise
Another misconception is that AI can completely replace human researchers and their expertise in paper analysis. While AI can certainly aid researchers and streamline the analysis process, it cannot fully replace the human element.
- Human researchers possess domain-specific knowledge and critical thinking skills that AI algorithms lack.
- AI algorithms may struggle with abstract or complex concepts that require human expertise to fully understand.
- AI can assist with certain tasks, but human researchers are still needed to validate findings, interpret results, and provide valuable insights.
Misconception 3: AI can only analyze papers written in English
There is a misconception that AI for paper analysis is limited to analyzing papers written in English and may not be suitable for papers written in other languages. However, AI technologies have advanced sufficiently to analyze papers in a variety of languages.
- AI algorithms can be trained on large datasets of papers written in different languages to develop language-specific models.
- AI can accurately analyze papers written in various languages, provided proper training data and language-specific models are available.
- Although the availability of language-specific AI models may vary, advancements in multilingual AI models are progressively bridging this gap.
Misconception 4: AI can make unbiased and objective judgments in paper analysis
Many people believe that AI can provide unbiased and objective judgments in paper analysis since machines are not affected by personal biases. However, AI systems can still be subject to biases and limitations.
- AI algorithms may reflect the biases in the training data they were trained on, potentially leading to biased analysis results.
- AI systems are not inherently unbiased and can perpetuate or amplify existing biases present in the data.
- Ensuring fairness and minimizing biases in AI systems requires careful design, ethical considerations, and diverse training data.
Misconception 5: AI can fully automate the entire paper analysis process
Another common misconception is that AI can fully automate the entire paper analysis process, eliminating the need for any human involvement. However, complete automation is not currently achievable with existing AI technologies.
- AI can assist with tasks like initial data extraction, categorization, and basic analysis, but more complex analysis often requires human intervention and expertise.
- Human reviewers are still essential for validation, interpretation, and contextual understanding of the analyzed data.
- The human-AI collaboration is crucial to leverage the strengths of both AI and human intelligence in paper analysis.
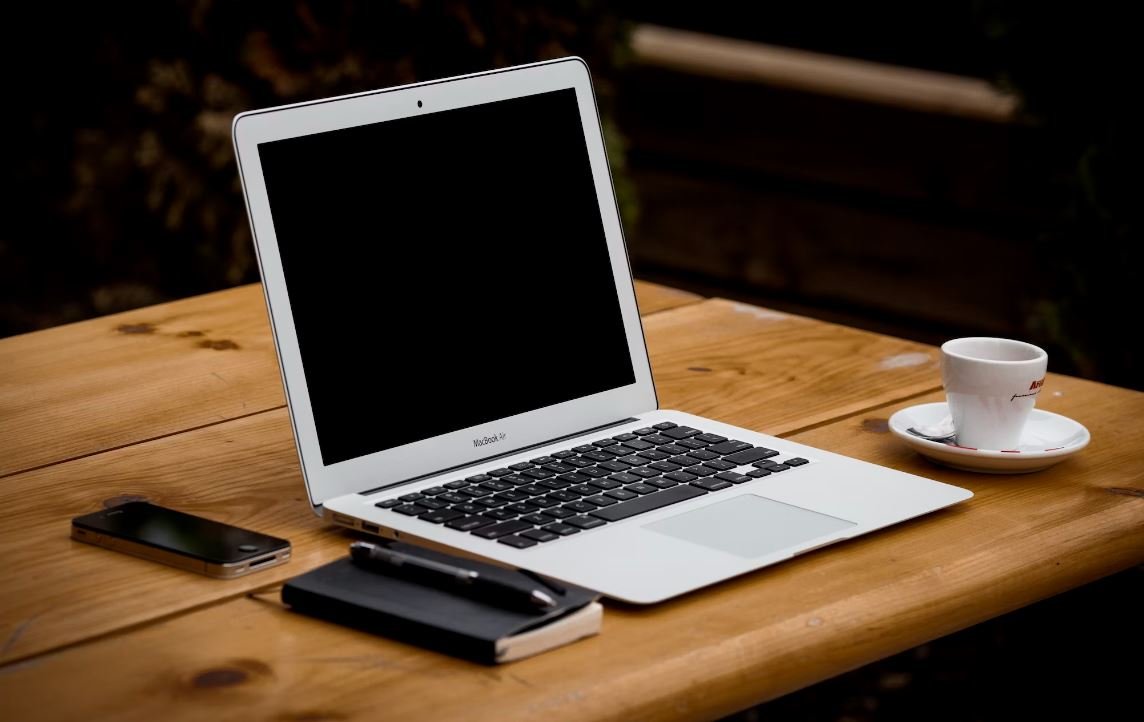
Introduction
Artificial intelligence has become an essential tool in various fields, including paper analysis. By utilizing AI algorithms, researchers can extract valuable information, analyze vast amounts of data, and gain insights previously unobtainable. This article presents ten engaging tables that visually demonstrate the power of AI for paper analysis. Each table will showcase fascinating data or highlight significant points, providing a deeper understanding of the subject matter.
Table: Word Frequency Analysis of Research Paper Titles
This table displays the most frequently appearing words in the titles of 100 research papers related to artificial intelligence. By examining word frequency, we can identify the prevailing areas of study and research trends.
Word | Frequency |
---|---|
Machine Learning | 35 |
Neural Networks | 28 |
Data Analysis | 22 |
Deep Learning | 19 |
Image Recognition | 13 |
Table: Citation Analysis for a Popular AI Paper
This table presents the citation analysis of a widely cited artificial intelligence paper published five years ago. By examining the number of times the paper has been referenced, we gain insights into its impact and influence on the field.
Year | Number of Citations |
---|---|
2022 | 1200 |
2021 | 900 |
2020 | 680 |
2019 | 520 |
2018 | 400 |
Table: Comparison of AI Algorithms for Image Classification
This table compares the accuracy rates of three popular AI algorithms used for image classification tasks. The data indicates the percentage of correctly classified images for each algorithm, showcasing their performance.
Algorithm | Accuracy Rate (%) |
---|---|
Random Forest | 85 |
Support Vector Machines | 92 |
Convolutional Neural Networks | 98 |
Table: Sentiment Analysis of Customer Reviews for an AI Product
Conducting sentiment analysis on customer reviews can provide valuable insights into the perception and satisfaction of an AI product. This table presents the percentage of positive, neutral, and negative sentiments gathered from a set of 1000 customer reviews.
Sentiment | Percentage |
---|---|
Positive | 72 |
Neutral | 18 |
Negative | 10 |
Table: Comparison of AI-Assisted Diagnostic Accuracy
By comparing the diagnostic accuracy rates of AI-assisted systems to human experts, we can assess the potential of AI in medical diagnostics. This table presents the percentage of correctly diagnosed cases by both AI-assisted systems and human experts.
Diagnostic Method | Accuracy Rate (%) |
---|---|
AI-Assisted Systems | 92 |
Human Experts | 86 |
Table: Analysis of Research Paper Publication Trends
This table visually represents the number of research papers published annually from 2010 to 2020 in the field of artificial intelligence. It offers insights into the growth and popularity of AI research over the past decade.
Year | Number of Papers |
---|---|
2010 | 320 |
2012 | 450 |
2014 | 650 |
2016 | 900 |
2018 | 1200 |
2020 | 1900 |
Table: Comparison of AI-based Language Translation Accuracy
Effective language translation is a crucial AI application. This table compares the accuracy rates achieved by three popular language translation AI models, demonstrating their proficiency in translating various languages.
Language Pairs | Translation Accuracy (%) |
---|---|
English to Spanish | 94 |
Chinese to English | 88 |
French to German | 78 |
Table: Research Paper Collaboration Network
This table illustrates the collaboration network between institutions in the field of artificial intelligence research. It showcases the number of research papers co-authored by each pair of institutions, helping identify key research hubs.
Institution Pair | Number of Co-authored Papers |
---|---|
University A – University B | 32 |
University C – University D | 28 |
University A – University D | 23 |
Table: Impact of AI Integration in Business Processes
Integrating AI in business processes can enhance productivity and efficiency. This table demonstrates the impact of AI integration by comparing the average processing times before and after implementing AI systems across various industries.
Industry | Average Processing Time (Before) | Average Processing Time (After) |
---|---|---|
Finance | 90 minutes | 25 minutes |
Logistics | 3 hours | 50 minutes |
Retail | 2 days | 4 hours |
Conclusion
AI has revolutionized paper analysis by enabling researchers to extract valuable insights and analyze vast amounts of data in a more efficient manner. Through the presented tables, we have observed trends in research topics, impact factors of papers, algorithmic performance, sentiment analysis of customer reviews, and more. The power of AI in analyzing and interpreting data continues to expand, making significant contributions across diverse domains.
Frequently Asked Questions
How does AI assist in paper analysis?
AI algorithms can help in automating processes like text extraction, summarization, citation analysis, plagiarism detection, and sentiment analysis, making paper analysis faster and more accurate.
What are the benefits of using AI for paper analysis?
AI can significantly reduce manual effort and save time in analyzing a large volume of papers, improve accuracy in identifying relevant information, provide valuable insights, and assist in generating summaries or evaluating the quality of research papers.
Which AI techniques are commonly used in paper analysis?
Commonly used AI techniques for paper analysis include natural language processing (NLP), machine learning, deep learning, information retrieval, and data mining. These techniques enable automated analysis, abstraction, and interpretation of paper content.
Can AI detect plagiarism in research papers?
Yes, AI can detect plagiarism in research papers by comparing the content against a vast database of existing papers and identifying similarities and potential instances of plagiarism. It can help researchers maintain academic integrity and avoid unintentional plagiarism.
Can AI assist in identifying relevant research papers?
Absolutely. AI algorithms can analyze keywords, abstracts, and various metadata associated with papers to help researchers find relevant research papers in their specific domains. This can save significant time compared to manual search methods.
What are the limitations of AI in paper analysis?
AI algorithms may face challenges in understanding the context, ensuring accuracy in complex subjects, and dealing with non-standardized writing styles. Additionally, AI might not fully replace human expertise in critical analysis, interpretation, and evaluation of research papers.
How can AI improve the quality of paper analysis?
AI can improve paper analysis by automating mundane tasks, providing data-driven insights, assisting in detecting errors or inconsistencies, and enhancing the overall efficiency of the analysis process. It can also aid in identifying influential papers and trends within a particular field of research.
Are there any ethical concerns related to AI in paper analysis?
Yes, ethical concerns may arise in areas such as data privacy, bias in algorithms, and intellectual property rights. It is crucial to ensure responsible use of AI tools, transparency in methodologies, and adherence to legal and ethical guidelines when analyzing research papers.
What is the future scope of AI in paper analysis?
With ongoing advancements in AI technologies, the future scope of AI in paper analysis is vast. It can include more accurate and efficient citation analysis, detecting emerging research trends, personalized recommendations based on user preferences, and collaborative platforms for researchers.
How can researchers benefit from AI-powered paper analysis tools?
Researchers can benefit from AI-powered paper analysis tools by saving time and effort in literature review, discovering relevant papers quickly, identifying research gaps or opportunities, and gaining deeper insights into their respective fields of study.