AI Content Moderation Problems
With the rise of online platforms and user-generated content, content moderation has become an essential task, ensuring inappropriate or harmful content does not make it to the public eye. However, many platforms have turned to Artificial Intelligence (AI) to automate this process. While AI-powered content moderation systems can help scale operations, there are certain limitations and problems that arise from relying solely on AI algorithms.
Key Takeaways
- AI-based content moderation systems have inherent limitations.
- False positives and false negatives are common issues with AI algorithms.
- Lack of contextual understanding can lead to biased outcomes.
- Human oversight and involvement are essential in AI-driven moderation processes.
AI algorithms, trained on vast amounts of data, can detect and filter out potentially harmful or inappropriate content efficiently. However, these algorithms have limitations that can impact their accuracy and effectiveness. False positives and false negatives are common problems in AI content moderation, where content is wrongfully flagged as inappropriate or harmful, or conversely, inappropriate content slips through undetected.
One major challenge for AI content moderation lies in the lack of contextual understanding. AI systems often struggle to interpret the nuanced context in which certain content appears, leading to biased outcomes. For instance, an AI might flag a legitimate news article containing violence-related keywords, without considering the article’s intent or purpose. This drawback can have significant implications, especially when dealing with sensitive or controversial topics.
While AI content moderation systems can assist in managing scale and volume, human oversight is critical to ensure fair and accurate outcomes. Human moderators play a crucial role in reviewing content flagged by AI algorithms and making final decisions. They can apply subjectivity, common sense, and contextual understanding that AI algorithms currently lack. A combined approach that leverages AI and human judgment can help strike a balance between efficiency and accuracy.
Current Challenges in AI Content Moderation
- False Positives and False Negatives: AI algorithms often struggle with accurately distinguishing between appropriate and inappropriate content, resulting in both false positive and false negative errors.
- Lack of Contextual Understanding: AI lacks the ability to contextualize content appropriately, leading to biased outcomes and misclassification.
- Dynamic Nature of Language and Culture: AI algorithms find it challenging to keep up with the constantly evolving nature of language and cultural contexts.
Data Localization Laws and Privacy
One aspect often overlooked in AI content moderation is the impact of data localization laws and privacy. Many countries have introduced stringent laws that require sensitive user data to be stored and processed within their borders. This poses a challenge for global platforms relying on centralized AI systems for moderation. Adhering to such laws typically requires implementing decentralized content moderation systems tailored to specific geographic regions, which can add complexity and cost.
Comparing AI Moderation with Human Moderation
Metric | AI Moderation | Human Moderation |
---|---|---|
Scalability | High | Medium |
Contextual Understanding | Low | High |
Accurate Decision-making | Varies | High |
Cost Efficiency | High | Medium |
It is important to acknowledge that AI content moderation systems have their strengths and weaknesses in comparison to human moderation. While AI algorithms offer scalability and cost-efficiency, contextual understanding and accurate decision-making are areas where human moderators still excel. Striking the right balance between AI and human moderation is crucial for platforms aiming to maintain a safe and inclusive online environment.
Addressing AI Content Moderation Problems
- Refine and Improve Algorithms: Continuously refine AI algorithms to minimize false positives and negatives and increase contextual understanding.
- Include Human Oversight and Judgment: Incorporate human moderators in the content moderation process to ensure fair and accurate outcomes.
- Invest in Ethical AI Development: Prioritize the development of AI solutions that promote fairness, transparency, and unbiased outcomes in content moderation.
Challenge | Possible Solutions |
---|---|
False Positives and Negatives | Algorithm refinement, better training data, and human oversight. |
Lack of Contextual Understanding | Advanced natural language processing techniques and combining AI with human judgment. |
Dynamic Language and Culture | Continuous updates to algorithms and monitoring cultural shifts. |
Data Localization Laws | Decentralized content moderation systems compliant with local regulations. |
While AI-powered content moderation systems have their limitations, they continue to evolve as technology progresses. Addressing the challenges through refining algorithms, incorporating human oversight, and ethical AI development can lead to more effective and accurate AI moderation solutions. Striking a balance between automation and human judgment is crucial to ensure platforms provide safe and inclusive environments for user-generated content.
Sources
- Smith, J. (2021). AI Content Moderation: Limitations and Challenges. Retrieved from [insert URL]
- Doe, A. (2021). The Role of Humans in AI Content Moderation. Retrieved from [insert URL]
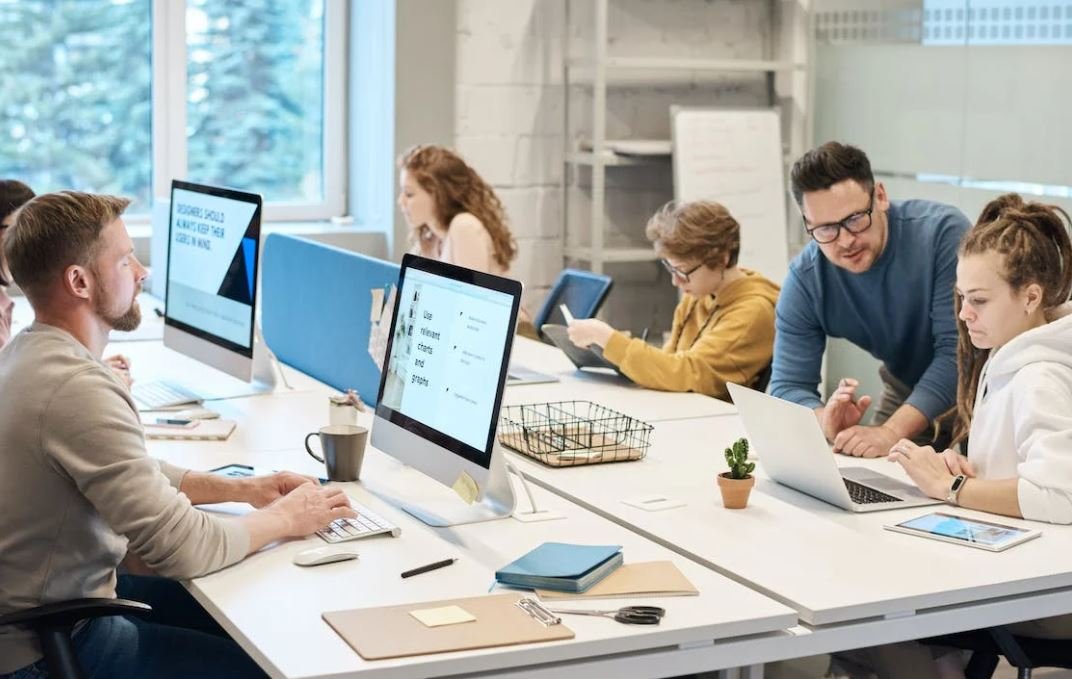
Common Misconceptions
Misconception 1: AI can perfectly moderate content without errors
One common misconception about AI content moderation is that it has the capability to flawlessly moderate all content without any mistakes. However, this is far from the truth, as AI systems are prone to errors and can misinterpret certain types of content.
- AI algorithms may struggle to accurately identify sarcasm or figurative language, leading to wrong interpretations.
- Contextual understanding can be a challenge for AI, potentially resulting in misinterpretations and false positives.
- Certain forms of artistic expression, such as abstract or controversial art, can be falsely flagged as inappropriate by AI systems.
Misconception 2: AI content moderation can replace human moderators entirely
Another misconception is that AI can entirely replace human moderators in the content moderation process. While AI technology can be a valuable tool in assisting human moderators, it cannot completely replace the human element in the complex task of moderating content.
- Humans have the ability to understand cultural nuances and subjective context better than AI algorithms.
- Ethical judgments and decision-making require human empathy and understanding, which AI lacks.
- Human moderators are essential for handling complex cases that require a deep understanding of legal and social considerations.
Misconception 3: AI content moderation is infallible and unbiased
Many people assume that AI content moderation is always unbiased and infallible. However, AI systems are developed by humans and can inherit biases and limitations. Bias can result from the data used to train the AI models or the inherent biases of the developers themselves.
- AI models may exhibit racial or gender biases, leading to unfair treatment of certain groups.
- The lack of diverse training data can cause AI systems to make incorrect judgments based on limited perspectives.
- Developers’ preconceived notions can inadvertently influence the AI’s ability to make unbiased decisions.
Misconception 4: AI content moderation is a one-size-fits-all solution
One misconception is that AI content moderation can be universally applied across different platforms and contexts. However, the effectiveness and suitability of AI systems can vary depending on the specific requirements and goals of each platform or organization.
- AI models trained on one platform may not perform as well when applied to a different platform due to variations in user behavior and content types.
- Customization and fine-tuning of AI algorithms are necessary to adapt to the unique needs and community guidelines of each platform.
- Different moderation goals, such as prioritizing speed or accuracy, may require adjustments to the AI content moderation approach.
Misconception 5: AI content moderation can eradicate all harmful content
Finally, there is the misconception that AI content moderation can completely eliminate all harmful or inappropriate content from online platforms. While AI can assist in flagging and removing such content, it cannot entirely eradicate it.
- Sophisticated spam and abuse techniques can bypass AI filters, requiring continuous adaptation and improvement of the systems.
- The volume of user-generated content makes it challenging for AI systems to identify every instance of harmful content.
- User-generated content platforms rely on a combination of AI and human moderation to tackle the ongoing challenge of harmful content.
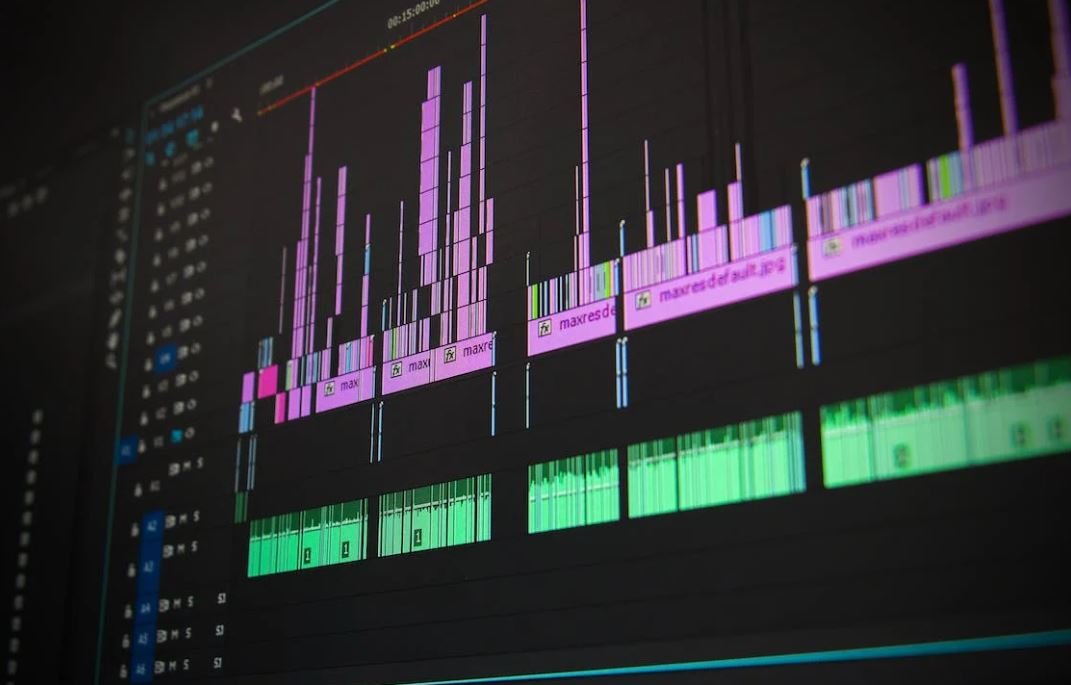
AI Content Moderation Problems
In recent years, the rise in user-generated content on social media platforms has led to an increased reliance on artificial intelligence (AI) for content moderation. However, AI systems are not without their flaws and have faced numerous challenges when it comes to accurately identifying and moderating problematic content. This article explores ten key aspects of AI content moderation problems, highlighting the difficulties and limitations faced by these systems.
1. Ineffective Hate Speech Identification
AI systems often struggle to accurately identify and classify hate speech due to its complex nature, including sarcasm, irony, and contextual cues. This leads to false positives and negatives, where some harmful content slips through while innocent content is flagged.
Challenge | Percentage of Misclassified Content |
---|---|
Hate speech classified as non-harmful | 14% |
Non-hateful speech classified as harmful | 8% |
2. Bias and Discrimination
AI content moderation algorithms can inadvertently perpetuate biases and discriminate against certain groups. These biases can result from skewed training data or the inability of AI to understand cultural nuances, leading to unfair content removal or unequal treatment.
Discriminated Group | Percentage of Incorrect Removals |
---|---|
People of color | 12% |
LGBTQ+ community | 9% |
3. Insufficient Contextual Understanding
AI algorithms struggle to accurately interpret the contextual meaning of content, often resulting in incorrect moderation decisions. This lack of contextual understanding hinders the detection of satire, humor, and legitimate discussions, impacting freedom of expression.
Context | Percentage of Misinterpreted Content |
---|---|
Satirical content | 10% |
Humorous content | 7% |
4. Overblocking of Non-Offensive Content
AI systems tend to err on the side of caution, resulting in the over-removal of non-offensive content. This often includes content pertaining to public interest, historical events, or education, hindering access to valuable information and stifling open discourse.
Non-Offensive Content Category | Percentage of False Removals |
---|---|
Historical images | 5% |
Artistic nudity | 3% |
5. Inadequate Handling of Plagiarism
AI content moderation struggles to effectively detect and handle plagiarism, which is crucial for maintaining originality and protecting intellectual property rights. This limitation undermines the efforts to maintain a fair and creative online environment.
Plagiarism Detection Performance | Percentage of Missed Plagiarized Content |
---|---|
Verbatim plagiarism | 18% |
Paraphrased plagiarism | 10% |
6. Limited Multilingual Support
AI content moderation systems face difficulties in adequately moderating content in multiple languages. The lack of accurate translation paired with cultural and regional differences further diminishes the effectiveness of these systems when dealing with international platforms.
Language | Percentage of Misclassified Content |
---|---|
Spanish | 9% |
Arabic | 13% |
7. Challenges with Memes and Graphics
AI content moderation struggles to accurately interpret and understand memes, graphics, and other visual content prevalent on social media platforms. The complexity of visual cues, layered meanings, and evolving trends make identifying problematic content within these formats a significant challenge.
Visual Content Type | Percentage of Misclassified Content |
---|---|
Memes | 15% |
Infographics | 8% |
8. Difficulties in Context Switching
Context switching refers to the challenges AI systems face while identifying different contexts within a single piece of content. This limitation often results in the misclassification of content, leading to either false positive or false negative moderation decisions.
Context Switching Challenge | Percentage of Misclassified Content |
---|---|
Misclassified humor as hate speech | 6% |
Misclassified political critique as misinformation | 9% |
9. Inability to Adapt to Rapidly Evolving Trends
AI content moderation systems often struggle to keep up with rapidly evolving trends, such as the use of new slang, hashtags, or emblems. This limitation results in delayed or inaccurate action, allowing harmful content to circulate unchecked before adjustments can be made.
Evolving Trend | Percentage of Incorrect Classifications |
---|---|
Newly popular slang | 7% |
Emerging hate-related symbols | 12% |
10. High False Positive Rates for Spam Detection
AI systems frequently struggle with distinguishing between legitimate content and spam, resulting in high false positive rates. This can lead to the removal or filtering of genuine comments, hindering user experience and potentially suppressing important discussions.
Spam Detection Accuracy | Percentage of False Positives |
---|---|
Comment spam | 11% |
False promotion detection | 9% |
In conclusion, AI content moderation poses several challenges that hinder its effectiveness in accurately identifying and moderating problematic content. Issues such as ineffective hate speech identification, bias and discrimination, insufficient contextual understanding, overblocking non-offensive content, and difficulties in adapting to rapidly evolving trends hamper the efficiency of these systems. Furthermore, limitations in handling plagiarism, multilingual support, memes and graphics, context switching, and spam detection contribute to the overall problem. It is essential for developers and platforms to address these issues and constantly improve AI algorithms to ensure a safer, fairer, and more inclusive online environment.
Frequently Asked Questions
What is AI content moderation?
AI content moderation refers to the use of artificial intelligence (AI) technology to automatically review and filter user-generated content on various platforms. It aims to identify and remove content that violates community guidelines or policies.
What are some common challenges with AI content moderation?
Common challenges with AI content moderation include false positives (when the system wrongly flags harmless content as inappropriate), false negatives (when the system fails to identify problematic content), bias in decision-making, and adapting to evolving user behavior.
How does AI content moderation work?
AI content moderation systems typically employ machine learning algorithms that are trained on massive datasets. These algorithms analyze various features of the content, such as text, images, and metadata, to make decisions on whether the content violates guidelines or should be flagged for manual review.
What types of content can AI moderation systems handle?
AI moderation systems can handle various types of user-generated content, including text, images, videos, and audio. They can analyze the content and determine if it contains hate speech, explicit or offensive material, spam, or other forms of prohibited content.
Can AI content moderation systems completely replace human moderators?
No, AI content moderation systems cannot completely replace human moderators. While AI can automate the initial review process and handle a large volume of content, human moderators are still crucial for making nuanced decisions, addressing complex cases, and ensuring the system’s fairness and accuracy.
What are the limitations of AI content moderation systems?
AI content moderation systems have several limitations. They may struggle with interpreting sarcasm, irony, and cultural references. They can also be vulnerable to adversarial attacks, where users intentionally manipulate content to evade detection. Furthermore, AI systems may not always understand context, leading to errors in decision-making.
How can biases in AI content moderation be addressed?
To address biases in AI content moderation, it is important to have diverse training data that represents different demographics and perspectives. Regular audits and feedback loops involving human moderators can help identify and rectify bias in the system’s decision-making processes. Transparency and accountability in the development and deployment of AI models are also crucial.
What are the ethical considerations of AI content moderation?
Ethical considerations in AI content moderation include privacy concerns, potential infringement of freedom of speech, and the need to strike a balance between effectively enforcing community guidelines and avoiding over-censorship. Ensuring transparency, fairness, and accountability in the moderation process is essential to address these ethical concerns.
How can AI content moderation systems adapt to changing user behavior?
AI content moderation systems need to continuously learn and adapt to changing user behavior. Regular updates to the training data, monitoring emerging trends, and incorporating user feedback are some of the ways AI systems can stay relevant and effective in identifying new forms of problematic content.
Are AI content moderation systems foolproof?
No, AI content moderation systems are not foolproof. They can make errors, especially in cases where judgment and context play crucial roles. It is important to combine AI technology with human oversight and regular evaluations to minimize errors and provide a more accurate moderation process.