AI Content Analysis
AI content analysis, also known as AI text analysis or AI semantic analysis, is a powerful technology that uses artificial intelligence to analyze and understand written content. It involves the use of algorithms and machine learning to extract meaningful insights from text, allowing businesses and individuals to gain valuable information from large amounts of unstructured data. This technology has the potential to revolutionize various industries, from marketing and customer service to legal and healthcare.
Key Takeaways:
- AI content analysis uses artificial intelligence to analyze written content.
- It extracts valuable insights from unstructured data.
- This technology has diverse applications across multiple industries.
**AI content analysis has the ability to process and analyze large volumes of text in a matter of seconds**, something that would take humans a considerable amount of time and effort to accomplish. By utilizing natural language processing (NLP) techniques and machine learning algorithms, AI systems can understand the context, sentiment, and semantic meaning of textual data. This enables organizations to automate tasks related to content analysis, such as sentiment analysis, topic detection, keyword extraction, and more.
AI content analysis is not limited to a specific industry or sector. Its applications are widespread and can benefit organizations in multiple ways. In marketing, for example, businesses can use AI content analysis to gain insights into customer preferences, sentiment analysis of reviews and feedback, and analyze social media interactions. Legal professionals can leverage this technology to review and analyze vast amounts of legal documents or contracts, saving time and reducing the risk of human error. Healthcare organizations can use AI content analysis to mine medical literature and research papers to support evidence-based decision making.
**One interesting aspect of AI content analysis is its ability to detect and understand emotions expressed in text**. Sentiment analysis, a key component of AI content analysis, can identify the sentiment or emotion behind a piece of text, such as positive, negative, or neutral. This information can be invaluable for companies to monitor customer satisfaction, track brand sentiment, and identify potential issues or opportunities.
How AI Content Analysis Works
AI content analysis involves several steps and processes to analyze and extract meaningful insights from text. Here’s a high-level overview:
- **Text Preprocessing**: In this step, the text is prepared for analysis by removing noise, such as punctuation, stopwords, and special characters. It may also involve stemming or lemmatization to reduce words to their root form.
- **Feature Extraction**: The next step is to extract relevant features or information from the preprocessed text. This can include identifying keywords, entities, or topics.
- **Machine Learning**: AI content analysis often harnesses the power of machine learning algorithms. These algorithms learn from training data to make predictions or classifications based on patterns and statistical models.
- **Semantic Analysis**: The text is analyzed for its contextual and semantic meaning, including sentiment analysis, topic modeling, or understanding relationships between words.
- **Insight Generation**: After the analysis, insights and actionable information are generated, allowing organizations to make data-driven decisions and take appropriate actions.
**Table 1: Applications of AI Content Analysis in Different Industries**
Industry | Applications |
---|---|
Marketing | Sentiment analysis of customer feedback, social media monitoring, content optimization |
Legal | Contract analysis, eDiscovery, legal document review |
Healthcare | Medical research analysis, patient sentiment analysis, disease tracking |
AI content analysis relies on the vast amount of data available to train and improve its accuracy and effectiveness. The more data an AI system is exposed to, the better it becomes at understanding and analyzing text. Furthermore, AI content analysis can be integrated with other AI technologies such as chatbots or virtual assistants, enhancing their capabilities and providing personalized interactions to users.
**One interesting trend in AI content analysis is the integration of visual analysis**. By combining image recognition and text analysis, businesses can gain deeper insights by analyzing both textual and visual data. This can be particularly useful in analyzing social media posts or customer reviews that include images or videos, allowing for a more comprehensive understanding of customer sentiment and preferences.
Table 2: Benefits of AI Content Analysis
Benefits | Explanation |
---|---|
Time-saving | AI can analyze large volumes of text in seconds, reducing manual effort and increasing efficiency. |
Improved accuracy | AI systems can consistently and accurately extract insights from text, minimizing errors and biases. |
Enhanced decision-making | By providing actionable insights, AI content analysis helps organizations make data-driven decisions. |
AI content analysis is continuously evolving and improving, with new techniques and algorithms being developed regularly. As AI technology advances, AI content analysis will become more sophisticated and capable of understanding and analyzing text with increasing accuracy. It presents significant opportunities for businesses to gain valuable insights from their textual data and make informed decisions based on data-driven analysis.
**One exciting direction for AI content analysis is the integration with voice recognition technology**. As voice assistants and voice-enabled devices become more prevalent, AI content analysis has the potential to analyze and understand spoken language, enabling businesses to extract insights from customer interactions and provide personalized recommendations or responses.
Table 3: Challenges in AI Content Analysis
Challenges | Explanation |
---|---|
Data privacy and security | Handling sensitive or confidential information, ensuring data protection and compliance. |
Domain-specific understanding | Understanding industry-specific jargon and terminology to accurately analyze domain-specific content. |
Cultural and contextual sensitivity | Understanding nuances, sarcasm, or humor in text that can vary across cultures and contexts. |
AI content analysis is an exciting and rapidly growing field that holds immense potential for various industries. With its ability to analyze large volumes of text, understand sentiments, and generate actionable insights, AI content analysis offers businesses and individuals a valuable tool to make informed decisions, improve customer satisfaction, and stay ahead of the competition.

Common Misconceptions
AI Content Analysis
Misconception 1: AI Content Analysis will replace human creativity
One common misconception about AI Content Analysis is that it will replace human creativity in the content creation process. However, this is not true. AI can assist and enhance human creativity by providing valuable insights and suggestions, but it cannot replicate the unique human perspective and intuition.
- AI can generate data-driven ideas, but human creativity adds the personal touch.
- AI can automate repetitive tasks, freeing up time for creative thinking.
- A combination of AI and human creativity leads to better content outcomes.
Misconception 2: AI Content Analysis is infallible
Another misconception is that AI Content Analysis is infallible and always produces accurate results. While AI algorithms continue to improve, they are not devoid of errors or biases. It is crucial to critically evaluate and validate the outputs of AI Content Analysis, ensuring their alignment with human judgment and expectations.
- AI algorithms may have limitations and biases in their training data.
- Human oversight is necessary to analyze and verify the AI-generated insights.
- Regular updates and refinement are essential to improve the accuracy of AI Content Analysis.
Misconception 3: AI Content Analysis is only for large businesses
Many people believe that AI Content Analysis is only suitable for large businesses with abundant resources. However, AI tools and platforms have become increasingly accessible and affordable, making them relevant for businesses of all sizes. Small and medium-sized enterprises can also leverage AI Content Analysis to optimize their content strategies.
- AI Content Analysis tools come in various price ranges, catering to different budgets.
- Small businesses can benefit from AI Content Analysis to stay competitive in the digital landscape.
- Accessibility to AI tools is expanding, enabling wider adoption among businesses.
Misconception 4: AI Content Analysis threatens job security
One fear surrounding AI Content Analysis is that it will threaten job security for content creators and marketers. While AI technology may automate certain tasks, it also creates new opportunities and enhances job roles. Content creators can focus on leveraging AI insights to improve content quality and engage with the audience more effectively.
- AI can take over tedious, time-consuming tasks, allowing humans to focus on higher-level responsibilities.
- Content creators can collaborate with AI to enhance their efficiency and productivity.
- New roles, such as AI trainers, evaluators, and strategists, are emerging in content-related fields.
Misconception 5: AI Content Analysis compromises privacy
Concerns about privacy often arise when discussing AI Content Analysis. While AI tools analyze vast amounts of data, they do not necessarily compromise personal privacy. Responsible AI implementations prioritize data privacy and protection, ensuring that individuals’ personal information remains secure and anonymous.
- AI platforms adhere to privacy regulations, safeguarding user data.
- User consent and transparency are vital in AI Content Analysis implementations.
- Data anonymization techniques minimize the risk of identifying individuals through AI analysis.
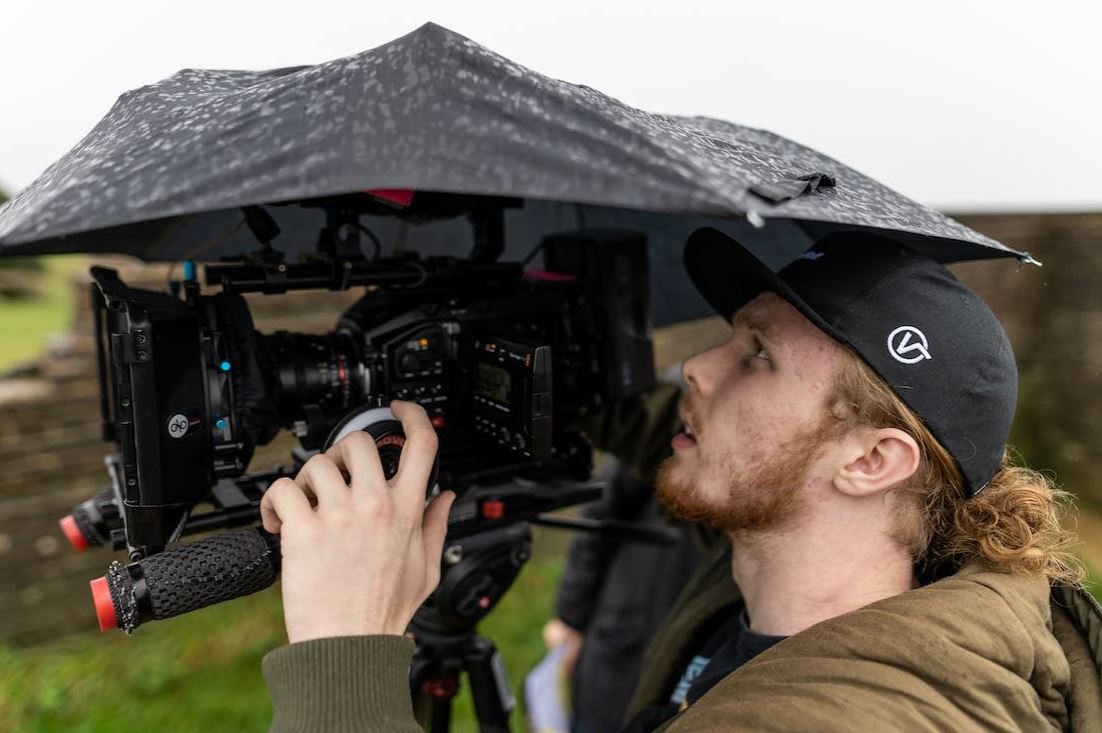
Introduction:
The emergence of AI technology has revolutionized content analysis by providing in-depth and data-driven insights. This article explores various aspects of AI-driven content analysis through ten captivating tables, highlighting its potential to transform industries, enhance decision-making, and optimize user experiences.
1. Social Media Engagement by Industry
Social media platforms play a crucial role in content consumption and engagement. This table categorizes different industries based on their average engagement rates to showcase which sectors benefit the most from AI-powered content analysis.
2. Sentiment Analysis of Customer Reviews
Understanding customers’ sentiments towards products or services is invaluable for businesses. This table displays sentiment analysis results for various products, indicating the percentage of positive, negative, and neutral customer reviews, enabling companies to identify areas for improvement.
3. Fake News Classification Rates
Controlling the spread of fake news is a pressing issue. This table compares the accuracy rates of different AI algorithms in classifying news articles as accurate or fake, emphasizing the role of AI content analysis in combating misinformation.
4. Keyword Frequency in Blog Posts
Optimizing content for search engines is critical for increasing visibility. This table showcases the frequency of keywords used in blog posts, providing insights into popular topics and helping content creators tailor their strategies accordingly.
5. Content Relevance to User Preferences
Personalizing content is essential for enhancing user experiences. This table exhibits the relevance of content recommendations to users based on their preferences, showcasing the effectiveness of AI content analysis in delivering tailored suggestions.
6. Trend Analysis of Social Media Hashtags
Monitoring trending hashtags on social media facilitates understanding popular topics. This table highlights the frequency of hashtags across different platforms, unveiling emerging trends, and enabling businesses to align their strategies with the latest discussions.
7. User Engagement with Email Campaigns
Analyzing user engagement with email campaigns is crucial for evaluating their effectiveness. This table presents click-through rates and conversion rates, indicating the impact of AI content analysis in optimizing email campaign strategies.
8. Web Page Loading Times by Content Type
Web page loading times significantly impact user experience. This table highlights the loading times of different content types (text, images, videos) on web pages, guiding content creators to minimize loading delays for a seamless browsing experience.
9. Advertisement Click-through Rates by Target Audience
Precise targeting is vital for effective advertising campaigns. This table compares click-through rates of advertisements across different target audience segments, demonstrating the role of AI content analysis in enhancing advertising strategies.
10. Content Performance on Search Engine Rankings
Achieving higher rankings on search engine result pages is paramount for content visibility. This table presents the search ranking positions of various content pieces, emphasizing the impact of AI content analysis in improving search engine optimization practices.
Conclusion:
AI-driven content analysis harbors immense potential for transforming how businesses understand, create, and deliver content. The illustrations provided through the ten captivating tables underscore the power of AI in analyzing social media engagement, sentiment analysis, fake news detection, keyword optimization, personalized recommendations, web performance, advertising effectiveness, and search engine rankings. By harnessing these data-driven insights, organizations can augment decision-making processes and ultimately optimize user experiences, fueling continued growth and success in the digital landscape.
Frequently Asked Questions
How does AI content analysis work?
AI content analysis utilizes machine learning algorithms to analyze and understand the content present in text documents or other forms of data. It involves the extraction of meaningful insights, identifying patterns, and categorizing the information based on predefined criteria, all done by the AI models trained on vast amounts of data.
What can be analyzed using AI content analysis?
AI content analysis can be used to analyze various forms of content, such as text documents, emails, social media posts, surveys, customer feedback, and more. It can help in sentiment analysis, topic categorization, entity recognition, identifying key phrases, and understanding the overall context of the content.
What are the benefits of using AI content analysis?
AI content analysis offers numerous benefits. It can help businesses gain valuable insights from large volumes of unstructured data quickly and accurately. It improves decision-making processes, aids in customer feedback analysis, enhances content categorization and organization, identifies emerging trends, and automates repetitive tasks, saving time and resources.
How accurate is AI content analysis?
The accuracy of AI content analysis depends on several factors, including the quality of the training data, the complexity of the content being analyzed, and the chosen AI model. While modern AI models can achieve high accuracy rates, it is essential to continuously fine-tune and validate the results to ensure optimal accuracy for specific use cases.
Is AI content analysis capable of understanding context?
Yes, AI content analysis models are designed to understand the context of the content they analyze. They can take into account language nuances, syntax, and semantic meaning to provide accurate insights. However, it is important to note that perfect contextual understanding is an ongoing area of research and may vary based on the specific use case and AI model used.
What industries can benefit from AI content analysis?
AI content analysis can benefit various industries such as marketing, customer service, finance, healthcare, e-commerce, social media monitoring, news organizations, and more. Any industry that deals with significant volumes of textual data can leverage AI content analysis to gain insights and automate processes.
Is AI content analysis capable of multilingual analysis?
Yes, AI content analysis can handle multiple languages. Many AI models are trained on multilingual datasets to understand and analyze content in different languages accurately. This enables businesses with international operations or a diverse customer base to extract insights and analyze multilingual content effectively.
Is AI content analysis completely automated?
AI content analysis is generally automated, using pre-trained models and algorithms to process the content. However, some level of human oversight and validation is often required, especially for critical tasks and to ensure the accuracy of the results. Human involvement may include fine-tuning models, validating outputs, and making subjective judgments based on domain-specific knowledge.
Can AI content analysis be customized for specific needs?
Yes, AI content analysis can be customized to meet specific needs. By using tailored training data, identifying relevant features, and fine-tuning the AI models, it is possible to enhance the accuracy and performance of content analysis for individual businesses and unique use cases. Customization allows for addressing specific industry jargon, domain-specific nuances, or unique data sources.
What are the limitations of AI content analysis?
While AI content analysis has numerous benefits, it also has limitations. Accuracy can be impacted by the quality of the training data, complexity of the content, and contextual understanding. AI models may struggle with sarcasm, irony, or cultural references, requiring additional fine-tuning. Privacy concerns and ethical considerations related to data usage and biases in training data also need to be addressed.