AI Bias Articles
Artificial Intelligence (AI) has played a significant role in various fields, revolutionizing the way we live, work, and interact. However, concerns have arisen regarding AI bias, where algorithms can perpetuate discriminatory behaviors and reinforce societal biases. This article will explore the concept of AI bias, its potential consequences, and approaches to mitigate it.
Key Takeaways
- AI bias refers to the discriminatory impact of algorithms.
- Biases in AI can have unintended consequences and perpetuate discrimination.
- Data quality, algorithmic design, and diversity in development teams play crucial roles in addressing AI bias.
Understanding AI Bias
AI bias occurs when algorithms exhibit prejudice or favoritism towards certain groups or individuals based on characteristics such as race, gender, or age. **Unintentional biases in data and biased algorithmic designs can contribute to AI bias**, leading to skewed or unfair outcomes. *Addressing AI bias is essential for building ethical and inclusive AI systems.*
The Impact of AI Bias
AI bias can have wide-ranging consequences on individuals and society as a whole. It can perpetuate existing inequalities, reinforce stereotypes, and lead to discriminatory practices. *Unchecked AI bias can undermine fairness, justice, and human rights.* Additionally, **AI bias can negatively impact decision-making processes** in various domains, including hiring practices, loan approvals, and criminal justice systems.
Common Sources of AI Bias
AI bias can stem from various sources throughout the development process. *Biased or incomplete training data can introduce inherent biases into the algorithms*. Algorithmic design choices, such as feature selection or weighting, can also contribute to bias. **Lack of diversity within development teams can further amplify bias** as the perspectives and experiences of underrepresented groups may not be adequately considered.
Addressing AI Bias
Mitigating AI bias requires a multi-faceted approach that involves addressing biases at different stages of the AI development lifecycle. **Ensuring high-quality and diverse training data is crucial for reducing biases**, as it helps to minimize skewed representation. Algorithmic fairness techniques and transparency measures can be implemented to detect and correct biases in AI systems. Additionally, promoting diversity and inclusion within development teams can help in **identifying and mitigating potential biases** during the design and development process.
Case Studies: AI Bias in Action
Issue | Impact | Solution |
---|---|---|
Facial recognition algorithms showed higher error rates for women and people of color. | Increased misidentifications and marginalization of certain groups. | Improving dataset diversity to provide more comprehensive representation and retraining algorithms to reduce biases. |
Issue | Impact | Solution |
---|---|---|
Sentencing algorithms exhibited racial bias, resulting in harsher sentences for certain racial groups. | Reinforced racial disparities within the criminal justice system. | Incorporating fairness metrics during algorithm development and continuous monitoring to identify and rectify biases. |
Issue | Impact | Solution |
---|---|---|
AI-powered screening tools discriminated against candidates from certain backgrounds. | Exclusion of qualified candidates based on biased evaluation criteria. | Regular auditing of AI systems for bias and involving diverse input when setting evaluation criteria. |
Conclusion
Addressing AI bias is crucial for creating fair, inclusive, and ethical AI systems. **By improving data quality, algorithmic design, and fostering diversity in development teams**, we can work towards minimizing biases in AI. It is essential to continually monitor and update AI systems to ensure they align with societal ethical standards.
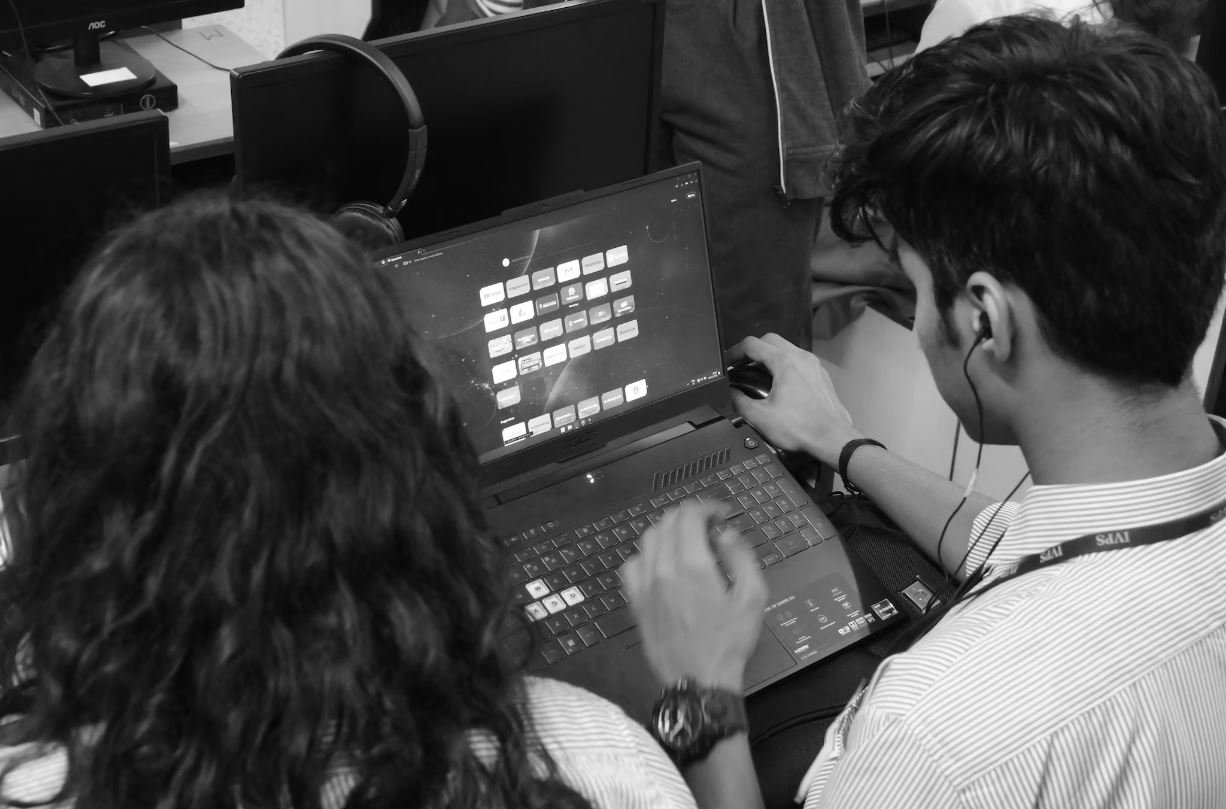
Common Misconceptions
Misconception 1: AI is completely objective and unbiased
One common misconception about artificial intelligence is that it is completely objective and unbiased. While AI systems are designed to make decisions based on data, they are not immune to biases. Here are three relevant points:
- AI algorithms are created by human programmers who can have their own unconscious biases.
- Data sets used to train AI models may already contain biases inherent in human society.
- AI systems can also perpetuate existing biases if not carefully monitored and corrected.
Misconception 2: AI can replace human judgment without bias
Another common misconception is that AI can replace human judgment without any bias. While AI can process vast amounts of data and make predictions or recommendations, it still needs human input to ensure fairness and ethical decision-making. Here are three relevant points:
- AI algorithms may not take into account the larger societal context necessary to make fair decisions.
- AI systems can only make decisions based on the data they are trained on, which may not capture all relevant factors.
- Human oversight is crucial to identify and address bias in AI systems and their outcomes.
Misconception 3: AI bias is intentional
Many people mistakenly believe that AI bias is intentionally programmed by developers. However, bias in AI systems is often unintentional and may result from flaws in the design or implementation process. Here are three relevant points:
- Developers may unintentionally introduce bias through the choice of training data or algorithms.
- Biases can arise due to inadequate diversity within the development teams working on AI projects.
- Addressing unintentional biases requires ongoing evaluation and iteration of AI systems.
Misconception 4: AI can eliminate all human biases
While AI has the potential to mitigate human biases, it cannot eliminate them entirely. AI systems learn from historical data, which can contain the biases present in human decision-making. Here are three relevant points:
- AI systems may inadvertently learn and perpetuate existing biases present in the training data.
- The biases of individuals involved in the development and deployment of AI can influence its outcomes.
- Regular audits and assessments are necessary to identify and correct biases in AI systems.
Misconception 5: Bias in AI is limited to controversial topics
Some people believe that bias in AI is only relevant to controversial topics or areas such as race or gender. However, biases can be present in AI systems across a wide range of domains and contexts. Here are three relevant points:
- Biases can arise in AI systems related to hiring, lending, healthcare, and criminal justice, among others.
- Even seemingly neutral algorithms can produce biased outcomes if the underlying data contains biases.
- Addressing bias in AI requires a holistic approach that considers all potential sources of bias across various domains.
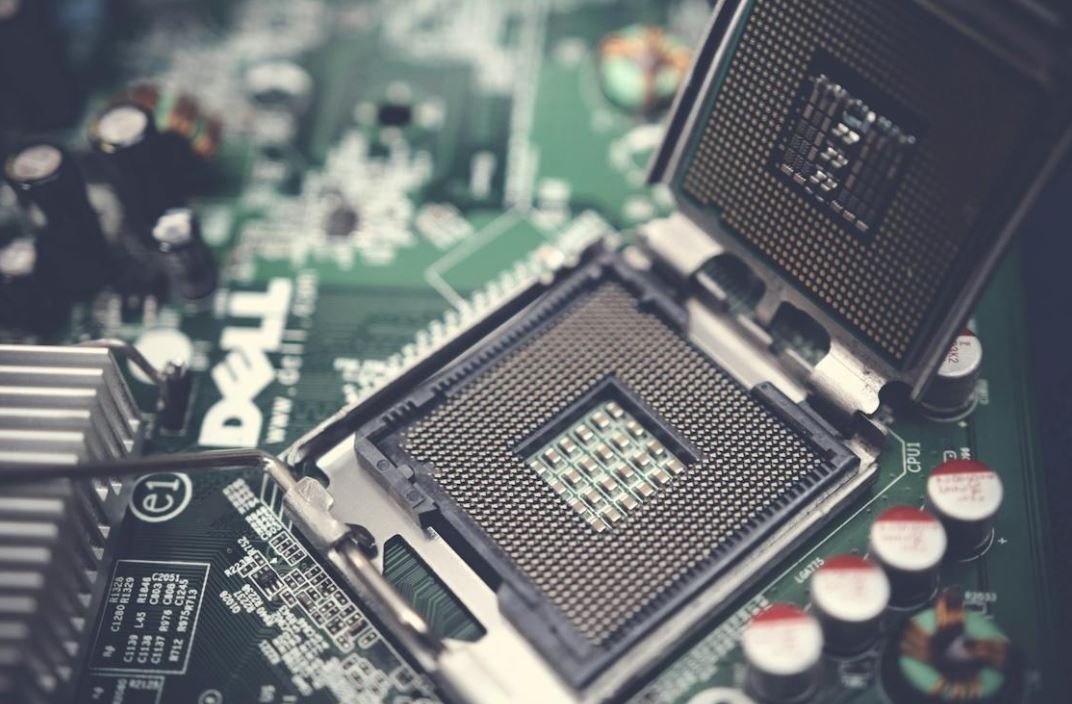
Table Title: Types of Biases in AI Systems
AI systems can exhibit various biases that may affect their decision-making processes. The table below provides an overview of the different types of biases commonly observed in AI systems.
| Bias Type | Description |
|——————————-|————————————————————————————————————————————–|
| Confirmation Bias | When an AI system favors information that confirms preexisting beliefs or assumptions. |
| Gender Bias | AI algorithms that discriminate against certain genders, impacting decisions related to employment, loan approvals, or voice recognition. |
| Racial Bias | AI systems that show bias toward individuals of certain racial backgrounds, leading to discriminatory actions in various domains. |
| Socioeconomic Bias | AI algorithms that favor or disadvantage individuals based on their socioeconomic status, exacerbating existing inequalities. |
| Algorithmic Bias | Biases embedded within the design and implementation of an algorithm, leading to unfair outcomes. |
| Data Bias | When an AI system is trained on unrepresentative or biased data, causing it to make biased or discriminatory predictions. |
| Cognitive Bias | AI systems that emulate human cognitive biases, potentially leading to flawed decision-making. |
| Political Bias | AI algorithms that exhibit partisan tendencies or discriminate against certain political views, impacting news and content recommendations. |
| Unintentional Bias | Bias that arises inadvertently in AI systems due to the complexity of their decision-making processes. |
| Acquisition Bias | Bias introduced through the way data is collected or acquired, leading to skewed representations in training datasets. |
Table Title: Impact of Bias in AI Systems
The presence of bias in AI systems can have far-reaching consequences, affecting individuals and society as a whole. The table below highlights some of the potential impacts associated with biased AI systems.
| Impact | Description |
|————————————|—————————————————————————————————————–|
| Unfair treatment | Biased AI systems may lead to individuals being disproportionately disadvantaged or excluded in various contexts. |
| Reinforcement of social biases | Biased AI can reinforce existing social biases, perpetuating discrimination and inequality in society. |
| Loss of opportunities | When AI systems exhibit bias, certain individuals or groups may miss out on important opportunities or resources. |
| Lack of trust and transparency | Biased AI erodes trust in automated decision-making and can raise concerns regarding transparency and accountability. |
| Diminished accuracy and reliability | Biased AI systems may produce inaccurate or unreliable results, rendering their outputs less trustworthy. |
Table Title: Strategies to Mitigate AI Bias
Efforts are being made to develop strategies and techniques to mitigate the impact of biases in AI systems. The table below presents some common approaches used to address AI bias.
| Mitigation Strategy | Description |
|———————————-|———————————————————————————————————————–|
| Diverse and inclusive datasets | Appropriate representation of diverse groups in training data to improve the impartiality and fairness of AI systems. |
| Continuous monitoring | Regular monitoring of AI systems to identify and address biases as they emerge, ensuring ongoing fairness. |
| Bias-aware algorithm design | Developing AI algorithms with an explicit focus on identifying and reducing biases, enhancing fairness and accuracy. |
| Explainability and transparency | Ensuring AI systems provide clear explanations for their decisions, allowing users to understand and challenge biases. |
| Ethical guidelines and regulations | Implementation of comprehensive ethical guidelines and regulations to guide the development and use of AI systems. |
| User feedback and input | Incorporating user feedback and input to improve AI systems, uncover biases, and enhance performance. |
| Bias testing and auditing | Conducting rigorous testing and auditing of AI systems to assess and rectify potential biases before deployment. |
| Collaborations and diversity | Encouraging collaborations among diverse stakeholders, including experts in various disciplines, to address AI bias. |
| Ongoing research and education | Supporting ongoing research and education to understand, uncover, and counter the biases present in AI systems. |
Table Title: Examples of Bias in AI Applications
Bias in AI systems can surface in various real-world applications. The table below illustrates specific examples of AI bias encountered in different domains.
| Application | Bias Type | Description |
|—————-|———————|—————————————————————————————————————————————————————-|
| Criminal justice system | Racial Bias | AI systems used for predicting recidivism have shown a bias against certain racial groups, leading to unfair decisions. |
| Facial recognition technology | Gender Bias | Some facial recognition algorithms have exhibited gender bias, misidentifying individuals belonging to certain genders more frequently. |
| Advertising platforms | Socioeconomic Bias | AI-driven advertising platforms have been found to show bias, resulting in the delivery of ads based on users’ socioeconomic status and perpetuating inequalities. |
| Employment recruitment | Algorithmic Bias | AI systems used in recruitment processes have shown bias against certain groups, leading to discriminatory practices in hiring. |
| Healthcare diagnostics | Bias in Data | AI systems for medical diagnostics can be biased against underrepresented groups if the training data isn’t diverse or inclusive enough. |
| Voice assistants | Cultural Bias | Voice assistants developed by certain companies have exhibited cultural biases, struggling to understand accents or specific dialects. |
| News recommendation systems | Political Bias | AI algorithms used in news recommendation systems have displayed political biases, favoring certain political views over others. |
| Loan approvals | Ethnicity Bias | Some AI systems used for loan approvals have been found to discriminate against individuals based on their ethnicity. |
| Autonomous vehicles | Age Discrimination | AI algorithms in autonomous vehicles have shown age discrimination, making decisions that prioritize the safety of younger individuals over older ones. |
| Education assessments | Socioeconomic Bias | AI-based education assessment systems have exhibited socioeconomic biases that disadvantage students from less privileged backgrounds. |
Table Title: Challenges in Addressing AI Bias
Despite efforts to mitigate AI bias, several challenges still hinder its complete eradication. The table below outlines some of the challenges faced in addressing AI bias.
| Challenge | Description |
|——————————————|—————————————————————————————————————————————|
| Lack of diverse representation in data | Incomplete or biased data samples can lead to biased AI systems, making it crucial to ensure diverse representation in training datasets. |
| Complex interplay of multiple biases | AI systems can exhibit various types of biases simultaneously, making it challenging to unravel their complex interplay and address them. |
| Scalability and resource requirements | Implementing bias mitigation strategies at scale can be resource-intensive, requiring significant computational power and infrastructure. |
| Lack of standardized evaluation metrics | The absence of standardized evaluation metrics makes it difficult to assess the effectiveness and fairness of bias mitigation techniques. |
| Continuous evolution of AI technologies | As AI techniques and technologies rapidly evolve, the effectiveness of existing mitigation strategies must be continuously evaluated and improved. |
| Ethical considerations and trade-offs | Addressing AI bias often involves ethical considerations, necessitating careful trade-offs between various factors such as accuracy and fairness. |
| Lack of interdisciplinary collaborations | Overcoming AI bias requires collaborations across different domains and expertise, which can pose challenges in terms of coordination and communication. |
Table Title: Case Studies: AI Bias in the Real World
Real-world case studies demonstrate the tangible impact of AI bias in various contexts. The table below presents noteworthy examples highlighting AI bias in action.
| Case Study | Domain | Description |
|——————————————|——————–|——————————————————————————————————————————————————————————————————————————————————–|
| Amazon’s gender-biased recruitment tool | Employment | Amazon developed an internal AI recruiting tool that exhibited gender bias, penalizing resumes containing terms frequently used by women. The bias stemmed from training the AI on historically male-dominated résumés. |
| ProPublica’s analysis of COMPAS | Criminal Justice | ProPublica conducted an analysis of the COMPAS recidivism prediction tool used in courtrooms. It discovered that the tool exhibited racial bias, incorrectly favoring white defendants over black defendants in its risk assessments. |
| Google Photos’ racial bias in image labeling | Image Recognition | Google Photos demonstrated a racial bias in its image labeling feature by mislabeling images of African Americans as gorillas. The incident highlighted how biases in training data could lead to discriminatory outputs in AI systems. |
| YouTube’s recommendation system | Content Recommendation | YouTube’s recommendation system has faced criticism for reinforcing extremist views and spreading misinformation due to its biased algorithms. The biases in the recommendation system limit users’ exposure to diverse perspectives. |
| Apple Card’s gender-biased credit limits | Financial Services | Apple Card faced accusations of discriminating against women by offering significantly lower credit limits to women, even when their financial situations were comparable to men. The bias seemed to be embedded in the algorithm used for credit assessments. |
Table Title: Key Players in AI Bias Mitigation
A range of organizations, institutions, and initiatives are actively dedicated to addressing AI bias and promoting fairness in AI systems. The table below highlights some key players contributing to AI bias mitigation efforts.
| Key Player | Description |
|———————————————————|———————————————————————-|
| Algorithmic Justice League | An organization advocating for ethical AI and combating algorithmic bias through research, policy advocacy, and public awareness. |
| Partnership on AI | A consortium of organizations, including tech giants, committed to advancing the understanding and addressing of ethical AI practices. |
| Fairness, Accountability, and Transparency in ML (FAT/ML) | A research community working on fair, accountable, and transparent ML systems, exploring techniques to reduce bias and ensure fairness. |
| World Economic Forum | The World Economic Forum offers guidelines and frameworks addressing AI bias and advocates for responsible AI development and deployment. |
| AI Now Institute | A multidisciplinary research center undertaking critical research on AI’s social implications and highlighting issues of fairness and bias. |
Table Title: Future Aspects and Implications
The future development and widespread deployment of AI systems require a careful examination of biases and their potential implications. The table below explores various aspects and implications related to the future of AI bias.
| Aspect/Implication | Description |
|—————————————-|—————————————————————————————————————————————-|
| Legal and regulatory frameworks | Future legal and regulatory frameworks must be established to govern the development, deployment, and use of AI systems, ensuring fairness. |
| Ethical considerations in AI design | Ethical considerations must be incorporated into the design phase of AI systems to prevent and address biases from the outset. |
| Enhancing diversity in AI development | Encouraging diversity and inclusivity in AI development teams can help reduce bias and ensure a broader perspective in system design. |
| Bias awareness in AI education | Education and training in AI should emphasize the importance of bias awareness, ensuring developers have the skills to tackle AI bias issues. |
| User empowerment and participation | Empowering users to understand and challenge AI systems and involving them in decision-making processes can promote fairer outcomes. |
| Long-term implications for society | Considering the long-term implications of AI bias for various aspects of society, including healthcare, justice, and social equity. |
| Accountability and responsibility | Determining accountability and responsibility in cases of bias in AI systems to ensure appropriate actions and remedies are implemented. |
| Balancing innovation and regulation | Striking a balance between fostering innovation in AI and implementing necessary regulations to mitigate bias and ensure public trust. |
| Collaboration and global standards | Collaboration among governments, organizations, and experts worldwide should be pursued to establish global standards for addressing AI bias. |
| Addressing bias in emerging AI domains | As AI expands into new domains, such as robotics and autonomous vehicles, preemptively addressing bias becomes crucial for equitable outcomes. |
Conclusion
The presence and impact of bias in AI systems have raised concerns about fairness, accountability, and social implications. This article explored the various types of biases observed in AI systems, their consequences, and strategies employed to mitigate bias. Real-world case studies and a diverse range of stakeholders dedicated to addressing AI bias were also highlighted. However, challenges remain in eliminating bias completely due to factors such as data limitations, complex interplay of biases, and evolving technologies. The future will require concerted efforts from researchers, policymakers, developers, and users to create AI systems that are fair, transparent, and trustworthy. By proactively identifying and addressing bias, we can strive for a more inclusive and equitable AI-powered future.
Frequently Asked Questions
What is AI bias?
AI bias refers to the phenomenon where artificial intelligence systems display partiality or favoritism towards particular groups or individuals based on race, gender, age, or other demographic factors. AI bias can lead to unfair treatment and discrimination.
How does AI bias occur?
AI bias can occur due to various reasons including biased training data, the algorithm’s design, and the influence of human bias during the development process. If the training data used to train an AI system contains inherent biases, the system might learn and amplify those biases.
What are the consequences of AI bias?
The consequences of AI bias can be far-reaching. It can perpetuate existing social inequalities, reinforce stereotypes, exacerbate discrimination, and lead to unfair decision-making. AI bias can negatively impact individuals and communities, hindering progress towards more inclusive and equitable societies.
How can AI bias be addressed?
Addressing AI bias requires a multi-faceted approach. It involves ensuring diverse and representative data sets for training AI models, improving algorithmic transparency, designing robust evaluation frameworks, promoting ethical guidelines for AI development, and fostering interdisciplinary collaboration between researchers, developers, policymakers, and affected communities.
Is AI bias intentional?
AI bias is not necessarily intentional. In most cases, biases arise unintentionally as a result of the limitations or flaws in the AI system’s design or training process. However, it is crucial to actively work towards identifying and eliminating biases to ensure fairness and prevent unintended harm.
Who is responsible for addressing AI bias?
The responsibility to address AI bias lies with various stakeholders. Developers and researchers have a responsibility to ensure their AI systems are designed to minimize bias. Policymakers play a role in regulating AI technologies to address bias and promote transparency. Society as a whole needs to engage in discussions and provide input to shape AI development and deployment to prevent bias and discrimination.
Can AI bias be completely eliminated?
While complete elimination of AI bias might be challenging, ongoing efforts can significantly reduce its occurrence. As technology advances and ethical considerations evolve, it is possible to develop more fair and unbiased AI systems. Continuous evaluation, transparency, and accountability are key components in the ongoing fight against AI bias.
What are some real-world examples of AI bias?
Real-world examples of AI bias include instances where facial recognition systems exhibit higher error rates for certain racial or gender groups, automated resume screening algorithms favor candidates from privileged backgrounds, and credit scoring systems discriminate against individuals based on factors unrelated to their creditworthiness. These examples highlight the need to address AI bias to prevent unfair outcomes.
How can AI bias impact decision-making processes?
AI bias can significantly impact decision-making processes. For example, biased AI systems used in law enforcement could lead to disproportionate targeting and unfair treatment of certain communities. In hiring processes, biased algorithms may perpetuate discriminatory practices, hindering diversity and perpetuating existing inequalities. It is essential to strive for bias-free AI systems to promote fairness in decision-making.
What role do human biases play in AI bias?
Human biases can have a significant influence on AI bias. During the development process, if individuals involved hold biased beliefs or if the training data reflects existing biases in society (due to historical discrimination, for example), these biases can be inadvertently encoded into the AI algorithm. Recognizing and addressing human biases is crucial in mitigating AI bias.